with open('tf_idf.txt', 'w', encoding='utf-8') as f: for word, tf in tf_values.items(): idf = idf_values.get(word, 0) tf_idf = tf * idf f.write('{}\t{}\n'.format(word, tf_idf))将上述代码的结果改为以tf-idf的值降序输出
时间: 2023-12-01 12:03:26 浏览: 60
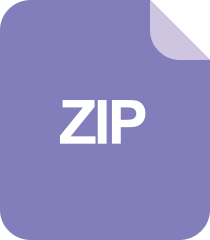
TF-IDF.py.zip_TF-IDF WEIGHT_tf-idf_tf_idf_特征提取
可以按照以下方式修改代码,使用sorted进行排序:
```
with open('tf_idf.txt', 'w', encoding='utf-8') as f:
for word, tf in tf_values.items():
idf = idf_values.get(word, 0)
tf_idf = tf * idf
f.write('{}\t{}\n'.format(word, tf_idf))
with open('tf_idf.txt', 'r', encoding='utf-8') as f:
lines = f.readlines()
sorted_lines = sorted(lines, key=lambda x: -float(x.split('\t')[1]))
with open('tf_idf_sorted.txt', 'w', encoding='utf-8') as f2:
f2.writelines(sorted_lines)
```
这个代码会将原本输出的文件名为 `tf_idf.txt` 的文件,读取出来按照 tf-idf 的值进行降序排序,然后将排序后的结果输出到文件名为 `tf_idf_sorted.txt` 的新文件中。
阅读全文
相关推荐
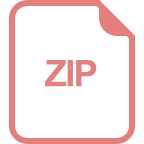
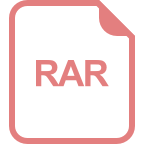

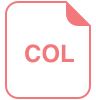
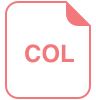
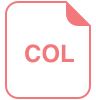





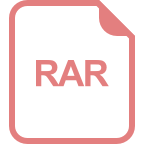
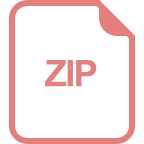
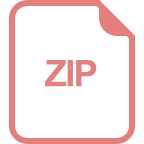
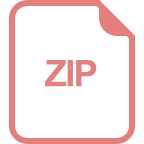
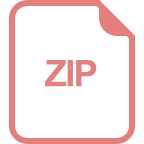