setwd("/Users/15878/Desktop/") data<-read_excel("huizhi.xlsx") b<-ggplot(data,aes(x=Beta(DL)/Beta(IM),y=GMV-Precuneus)) b+geom_point(size=2,color='blue')+ coord_fixed()+theme_classic()+ geom_smooth(method = "lm",formula = y ~ x,size=2,color="black",fill="green")+ theme (aspect.ratio=1,axis.text.x = element_text (size = 15),axis.title.x = element_text (size = 15),axis.title.y = element_text(size = 15),axis.text.y = element_text (size = 15))+ stat_cor(data=data, method = "pearson") ggsave("KRd.png",dpi=300,limitsize = FALSE,width=10,height=10,units="cm")解释代码
时间: 2023-11-14 07:06:11 浏览: 41
这段代码使用了 ggplot2 包来绘制散点图。首先,通过 `setwd()` 函数设置工作目录,然后使用 `read_excel()` 函数读取名为 "huizhi.xlsx" 的 Excel 文件中的数据。接下来,创建了一个 `ggplot()` 对象 `b`,并使用 `aes()` 函数指定了 x 轴和 y 轴的变量。通过 `geom_point()` 函数添加散点图层,并指定点的大小和颜色。使用 `coord_fixed()` 函数使 x 轴和 y 轴的比例相等。使用 `theme_classic()` 函数设置图表主题风格,使用 `geom_smooth()` 函数添加一条拟合直线层,指定了拟合方法为线性回归,并设置线的大小、颜色和填充颜色。使用 `theme()` 函数设置图表的主题元素,例如坐标轴标签和刻度标签的字体大小等。最后,使用 `stat_cor()` 函数添加了一个相关系数文本框,并使用 `ggsave()` 函数将图表保存为名为 "KRd.png" 的 PNG 文件,并指定了分辨率、大小和单位等参数。
相关问题
library(xlsx) setwd("D:/R") # 读取数据 data1 <- read.xlsx("试验.xlsx",sheetName = "man") data2 <- read.xlsx("试验.xlsx",sheetName = "weman") data3 <- read.xlsx("试验.xlsx",sheetName = "all") B <- data1$男孩 F <- data3$父亲 G <- data2$女孩 M <- data3$母亲 # t检验 t1=(mean(B)-mean(F))/(sd(B)/sqrt(length(B))) t1 p1 <- pt(t1,length(B)-1) p1 if (p1>0.05){ print("男生高于父亲") } else{ print("男生不高于父亲") } #检验结果为t=-5.123508,显著性P值=1.488898e-06>0.05,接受原假设,认为男生不高于父亲 t2=(mean(G)-mean(M))/(sd(G)/sqrt(length(G))) t2 p2 <- pt(t2,length(G)-1) p2 if (p2>0.05){ print("女生高于母亲") } else{ print("女生不高于母亲") } #检验结果为t=3.994843,显著性P值=0.9999377>0.05,接受原假设,认为女生高于母亲 # 计算结果 results <- list() # 中亲 - 子女 x <- data3$学生 y <- data3$中亲 lm.model <- lm(x ~ y, data = data3) model_summary <- summary(lm.model) model_coeffs <- coef(lm.model) standard_error <- model_summary$sigma CI <- confint(lm.model) cor_coef <- cor(x, y) p_value <- model_summary$coefficients[2,4] results[["中亲-子女"]] <- data.frame(coefficients = model_coeffs, std_error = standard_error, conf_int = CI, cor_coef = cor_coef, p_value = p_value) 方法分析
这段代码是一个用于统计学分析的R语言代码。主要包括以下几个方面的内容:
1. 读取数据:使用xlsx包中的read.xlsx函数读取名为“试验.xlsx”的Excel文件中的三个表格数据。
2. t检验:计算男孩身高和父亲身高的t检验值和P值,以及女孩身高和母亲身高的t检验值和P值,并根据P值判断身高是否有显著差异。
3. 线性回归:使用lm函数对数据进行线性回归分析,计算中亲与子女的相关系数、回归系数、标准误差、置信区间和P值,并将结果存储在名为results的列表中。
这些方法可以用于探索数据之间的关系和差异,帮助做出决策和推断。
library(showtext) showtext_auto() # 配置环境,读取数据 setwd("/Users/caitan/Desktop/方法论/rDataAnalysis") data <- read.csv("train.csv", header = FALSE) # 从data中读取温度、湿度、风速、损坏使用数据、登记使用数据,租赁总数据 temperature <- as.numeric(data[,6]) humidity <- as.numeric(data[,8]) windspeed <- as.numeric(data[,9]) casual <- as.numeric(data[,10]) register <- as.numeric(data[,11]) total <- as.numeric(data[,12])这段代码无法运行
这段代码可能存在以下问题:
1. 在执行 `showtext_auto()` 命令前,您需要先安装 `showtext` 包。您可以使用 `install.packages("showtext")` 命令安装该包。
2. 在读取 CSV 文件时,您需要指定正确的文件路径。
3. 如果您的 CSV 文件中存在空值或非数字值,那么在将数据转换为数值型时会出现错误。您可以使用 `na.strings` 参数来指定空值的表示方式。
4. 在读取数据后,您需要检查数据的类型和结构是否正确,以确保后续操作的正确性。
以下是可能可以运行的代码示例:
```{r}
library(showtext)
install.packages("showtext") # 安装 showtext 包
showtext_auto() # 配置环境
setwd("/Users/caitan/Desktop/方法论/rDataAnalysis")
data <- read.csv("train.csv", header = FALSE, na.strings = c("", "NA")) # 读取 CSV 文件并指定空值的表示方式
temperature <- as.numeric(data[,6])
humidity <- as.numeric(data[,8])
windspeed <- as.numeric(data[,9])
casual <- as.numeric(data[,10])
register <- as.numeric(data[,11])
total <- as.numeric(data[,12])
str(data) # 检查数据的类型和结构
```
相关推荐
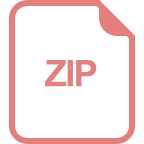
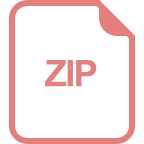
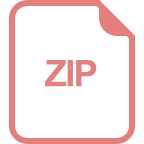













