x1=np.array(DATA.loc[,'单价(元/平米)'])
时间: 2024-04-17 07:28:15 浏览: 56
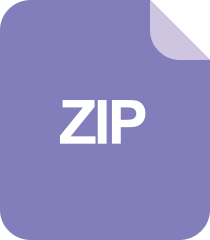
商品查找,单价计算
在这个代码中,`DATA` 是一个 DataFrame 对象,`x1` 是一个 numpy 数组。`np.array()` 函数用于将 `DATA.loc[,'单价(元/平米)']` 的结果转换为 numpy 数组。`DATA.loc[,'单价(元/平米)']` 表示从 DataFrame `DATA` 中选择 `单价(元/平米)` 这一列数据。
所以,`x1=np.array(DATA.loc[,'单价(元/平米)'])` 的作用是将 DataFrame `DATA` 中的 `单价(元/平米)` 这一列数据转换为 numpy 数组并赋值给变量 `x1`。
阅读全文
相关推荐
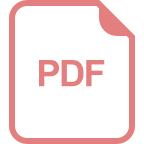
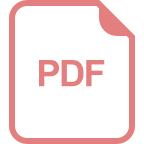






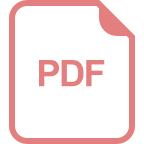


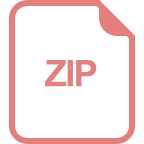
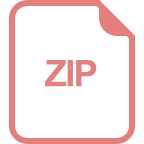
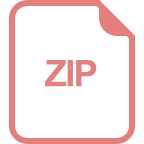
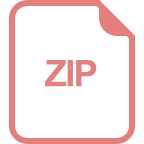
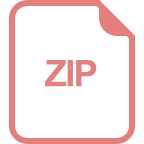