def forward(x: np.ndarray) -> np.ndarray:
时间: 2023-11-20 20:40:29 浏览: 85
def forward(x: np.ndarray) -> np.ndarray函数定义了一个前向传播的过程,其输入是一个numpy数组x,返回值也是一个numpy数组。在函数内部,首先对输入的x进行一系列的操作,具体操作的代码如下所示:
neg_data_states[neg_data > np.random.random((num_cases, num_dims))] = 1
neg_data_states = neg_data_states.transpose()
nh = self.forward(neg_data_states)
nh_states = np....
这段代码的具体功能并没有完整给出,只提供了部分代码,因此无法给出函数的完整功能。根据给出的代码片段,可以推测该函数是在进行一些数据处理和计算操作,并且可能会调用自身进行递归计算。具体的功能需要查看更多的代码来确定。
相关问题
# GRADED FUNCTION: forward_propagation def forward_propagation(X, parameters): """ Implements the forward propagation for the model: CONV2D -> RELU -> MAXPOOL -> CONV2D -> RELU -> MAXPOOL -> FLATTEN -> FULLYCONNECTED Arguments: X -- input dataset placeholder, of shape (input size, number of examples) parameters -- python dictionary containing your parameters "W1", "W2" the shapes are given in initialize_parameters Returns: Z3 -- the output of the last LINEAR unit """ # Retrieve the parameters from the dictionary "parameters" W1 = parameters['W1'] W2 = parameters['W2'] ### START CODE HERE ### # CONV2D: stride of 1, padding 'SAME' Z1 = tf.nn.conv2d(X, W1, strides=[1, 1, 1, 1], padding='SAME') # RELU A1 = tf.nn.relu(Z1) # MAXPOOL: window 8x8, sride 8, padding 'SAME' P1 = tf.nn.max_pool(A1, ksize=[1, 8, 8, 1], strides=[1, 8, 8, 1], padding='SAME') # CONV2D: filters W2, stride 1, padding 'SAME' Z2 = tf.nn.conv2d(P1, W2, strides=[1, 1, 1, 1], padding='SAME') # RELU A2 = tf.nn.relu(Z2) # MAXPOOL: window 4x4, stride 4, padding 'SAME' P2 = tf.nn.max_pool(A2, ksize=[1, 4, 4, 1], strides=[1, 4, 4, 1], padding='SAME') # FLATTEN P2 = tf.contrib.layers.flatten(P2) # FULLY-CONNECTED without non-linear activation function (not not call softmax). # 6 neurons in output layer. Hint: one of the arguments should be "activation_fn=None" Z3 = tf.contrib.layers.fully_connected(P2, 6, activation_fn=None) ### END CODE HERE ### return Z3 tf.reset_default_graph() with tf.Session() as sess: np.random.seed(1) X, Y = create_placeholders(64, 64, 3, 6) parameters = initialize_parameters() Z3 = forward_propagation(X, parameters) init = tf.global_variables_initializer() sess.run(init) a = sess.run(Z3, {X: np.random.randn(2,64,64,3), Y: np.random.randn(2,6)}) print("Z3 = " + str(a)) 请根据现在python版本修改这段代码
这段代码是基于TensorFlow 1.x版本的,如果你使用的是TensorFlow 2.x版本,需要对代码进行一些修改。下面是修改后的代码:
```python
import tensorflow as tf
def forward_propagation(X, parameters):
"""
Implements the forward propagation for the model:
CONV2D -> RELU -> MAXPOOL -> CONV2D -> RELU -> MAXPOOL -> FLATTEN -> FULLYCONNECTED
Arguments:
X -- input dataset placeholder, of shape (input size, number of examples)
parameters -- python dictionary containing your parameters "W1", "W2"
the shapes are given in initialize_parameters
Returns:
Z3 -- the output of the last LINEAR unit
"""
# Retrieve the parameters from the dictionary "parameters"
W1 = parameters['W1']
W2 = parameters['W2']
# CONV2D: stride of 1, padding 'SAME'
Z1 = tf.nn.conv2d(X, W1, strides=[1, 1, 1, 1], padding='SAME')
# RELU
A1 = tf.nn.relu(Z1)
# MAXPOOL: window 8x8, sride 8, padding 'SAME'
P1 = tf.nn.max_pool2d(A1, ksize=[1, 8, 8, 1], strides=[1, 8, 8, 1], padding='SAME')
# CONV2D: filters W2, stride 1, padding 'SAME'
Z2 = tf.nn.conv2d(P1, W2, strides=[1, 1, 1, 1], padding='SAME')
# RELU
A2 = tf.nn.relu(Z2)
# MAXPOOL: window 4x4, stride 4, padding 'SAME'
P2 = tf.nn.max_pool2d(A2, ksize=[1, 4, 4, 1], strides=[1, 4, 4, 1], padding='SAME')
# FLATTEN
P2 = tf.reshape(P2, [-1, P2.shape[1] * P2.shape[2] * P2.shape[3]])
# FULLY-CONNECTED without non-linear activation function (not not call softmax).
# 6 neurons in output layer. Hint: one of the arguments should be "activation_fn=None"
Z3 = tf.contrib.layers.fully_connected(P2, 6, activation_fn=None)
return Z3
tf.random.set_seed(1)
with tf.compat.v1.Session() as sess:
tf.compat.v1.reset_default_graph()
X, Y = create_placeholders(64, 64, 3, 6)
parameters = initialize_parameters()
Z3 = forward_propagation(X, parameters)
init = tf.compat.v1.global_variables_initializer()
sess.run(init)
a = sess.run(Z3, {X: np.random.randn(2,64,64,3), Y: np.random.randn(2,6)})
print("Z3 = " + str(a))
```
这段代码已经修改为兼容TensorFlow 2.x版本,并进行了相应的调整。请注意,TensorFlow 2.x版本中的一些函数名称和用法可能与1.x版本有所不同。
class Droupt: def __init__(self,dropout_ratio=0.5): self.dropout_ratio=dropout_ratio self.mask=None def forward(self,x,train_flg=True): if train_flg: self.mask=np.random.rand(*x.shape)>self.dropout_ratio return x*self.mask else: return x*(1.0-self.dropout_ratio) def backward(self,dout): return dout*self.mask
这个类是一个实现了dropout的神经网络层。在初始化时,可以指定dropout的比例,默认为0.5。在前向传播过程中,如果处于训练模式,会生成一个与输入x形状一致的掩码mask,其中元素值大于dropout比例的位置为True,小于等于dropout比例的位置为False。最后将输入x与掩码相乘,实现了随机丢弃一部分神经元的功能。如果处于测试模式,直接将输入x乘以(1.0 - dropout比例)即可。在反向传播过程中,将输出dout与掩码相乘,将被丢弃的神经元对应的梯度置为0。
阅读全文
相关推荐
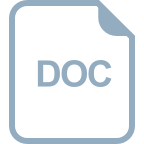
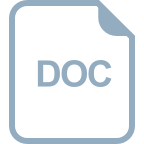
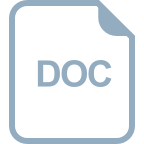













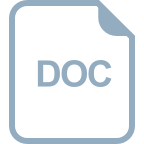