pd.to_datetime dayfirst
时间: 2023-11-09 08:48:28 浏览: 76
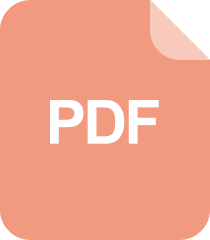
python中时间转换datetime和pd.to_datetime详析
通过设置`dayfirst`参数为True,可以将字符串解析为日期时间对象,并将日期的日部分视为首先出现的部分。
例如,使用`pd.to_datetime`函数将字符串解析为日期时间对象时,可以如下设置`dayfirst=True`:
```python
import pandas as pd
date_str = '31-12-2022' # 日期字符串
date = pd.to_datetime(date_str, dayfirst=True)
print(date)
```
输出:
```
2022-12-31 00:00:00
```
在上述示例中,由于`dayfirst=True`被设置为True,因此日期字符串中的日部分被解析为首先出现的部分,生成了对应的日期时间对象。
阅读全文
相关推荐
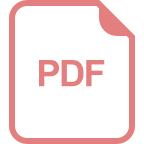
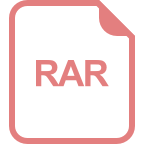
















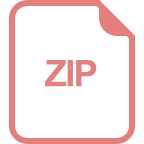