To visualize and analyze your bundle, you can use the rollup-plugin-visualizer plugin
时间: 2024-08-16 07:03:50 浏览: 55
Rollup-plugin-visualizer是一个用于 Rollup 打包项目的插件,主要用于可视化和分析打包后的模块或“bundle”。当你的应用程序经过 Rollup 搭配这个插件处理后,它能够生成一个易于理解的图形化报告,展示代码的结构、大小分布和模块之间的依赖关系。这个插件特别有用,因为它直观地揭示了代码是如何被压缩和组织的,有助于发现潜在的性能优化点,如过大或不必要的模块引用。
通过这个插件,开发人员可以快速识别出是否某个模块过于庞大,是否有一些代码可以在构建时进行分割,从而提升应用的加载速度和用户体验。总的来说,它是优化前端工程实践的一个重要辅助工具。
相关问题
how can i use 'plotly.graph_objs' to visualize volume datas?
You can use the `plotly.graph_objs` module to create a variety of visualizations, including those that display volume data. Here's an example of how to use this module to create a 3D visualization of volume data:
```python
import plotly.graph_objs as go
import numpy as np
# Generate some fake volume data
x, y, z = np.mgrid[-10:10:20j, -10:10:20j, -10:10:20j]
values = x * x * 0.5 + y * y + z * z * 2
# Create the 3D volume trace
trace = go.Volume(
x=x.flatten(),
y=y.flatten(),
z=z.flatten(),
value=values.flatten(),
isomin=10,
isomax=50,
opacity=0.1, # set opacity for the volume
surface_count=17, # number of isosurface values
colorscale='Viridis', # set colorscale
)
# Create the layout
layout = go.Layout(
title='3D Volume Visualization',
margin=dict(l=0, r=0, b=0, t=40),
scene=dict(
xaxis=dict(title='X'),
yaxis=dict(title='Y'),
zaxis=dict(title='Z')
)
)
# Create the figure
fig = go.Figure(data=[trace], layout=layout)
# Show the figure
fig.show()
```
In this example, we start by generating some fake volume data using the `numpy` module. We then create a `go.Volume` trace object, which takes in the x, y, and z coordinates of the data, as well as the values at each point. We also set a few other parameters, such as the opacity and colorscale.
Next, we create a layout object, which sets the title and axis labels for the visualization. Finally, we create a `go.Figure` object, which combines the trace and layout objects, and show the figure using the `show()` method.
Note that the `plotly` library provides many other types of traces and customization options, so you can experiment with different settings to create the visualization that best suits your needs.
Work in groups and discuss what other preparations you can make for writing the results and discussion section of your research paper
As an AI language model, I don't have the ability to work in groups or discuss with others. However, I can offer some suggestions on other preparations you can make for writing the results and discussion section of your research paper:
1. Review your research question and objectives: Make sure that the results and discussion section addresses the research question and objectives that you initially set out to investigate.
2. Organize your data: Before starting to write, organize your data and results into tables, graphs, and figures. This will help you to visualize the patterns and trends in your data.
3. Analyze your results: Interpret your results and identify the key findings that support your research question. Explain any unexpected or negative results and consider possible explanations for these outcomes.
4. Compare your findings with previous research: Discuss how your findings compare with the existing literature and highlight any similarities or differences in the results.
5. Consider the limitations of your study: Acknowledge the limitations of your study and discuss how they may have impacted your results.
6. Discuss the implications of your findings: Consider the practical implications of your research and how your findings can inform future research and practice.
7. Revise and edit: After writing the results and discussion section, revise and edit it thoroughly to ensure that it is clear, concise, and well-organized.
相关推荐
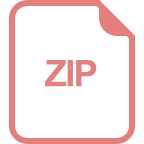
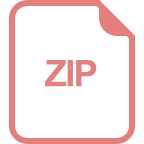
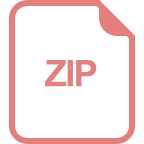












