transformer代码 keras
时间: 2024-12-26 12:15:58 浏览: 3
### 使用 Keras 实现 Transformer 模型
#### 创建多头自注意力机制作为 Keras 层
为了创建一个多头自注意力层,可以利用 `Layer` 类来自定义这一功能。此操作允许模型关注输入的不同部分。
```python
import tensorflow as tf
from tensorflow.keras.layers import Layer, Dense, Dropout
class MultiHeadSelfAttention(Layer):
def __init__(self, embed_dim, num_heads=8):
super(MultiHeadSelfAttention, self).__init__()
self.embed_dim = embed_dim
self.num_heads = num_heads
if embed_dim % num_heads != 0:
raise ValueError(
f"embedding dimension {embed_dim} should be divisible by number of heads {num_heads}"
)
self.projection_dim = embed_dim // num_heads
self.query_dense = Dense(embed_dim)
self.key_dense = Dense(embed_dim)
self.value_dense = Dense(embed_dim)
self.combine_heads = Dense(embed_dim)
def attention(self, query, key, value):
score = tf.matmul(query, key, transpose_b=True)
dim_key = tf.cast(tf.shape(key)[-1], tf.float32)
scaled_score = score / tf.math.sqrt(dim_key)
weights = tf.nn.softmax(scaled_score, axis=-1)
output = tf.matmul(weights, value)
return output, weights
def separate_heads(self, x, batch_size):
x = tf.reshape(x, (batch_size, -1, self.num_heads, self.projection_dim))
return tf.transpose(x, perm=[0, 2, 1, 3])
def call(self, inputs):
batch_size = tf.shape(inputs)[0]
query = self.query_dense(inputs)
key = self.key_dense(inputs)
value = self.value_dense(inputs)
query = self.separate_heads(query, batch_size)
key = self.separate_heads(key, batch_size)
value = self.separate_heads(value, batch_size)
attention, weights = self.attention(query, key, value)
attention = tf.transpose(attention, perm=[0, 2, 1, 3])
concat_attention = tf.reshape(attention, (batch_size, -1, self.embed_dim))
outputs = self.combine_heads(concat_attention)
return outputs
```
#### 构建一个 Transformer 块作为一个层
Transformer 的核心在于其能够并行化处理数据的能力以及它所使用的自我注意机制。下面是一个简单的 Transformer 块实现:
```python
class TransformerBlock(Layer):
def __init__(self, embed_dim, num_heads, ff_dim, rate=0.1):
super(TransformerBlock, self).__init__()
self.att = MultiHeadSelfAttention(embed_dim, num_heads)
self.ffn = tf.keras.Sequential(
[Dense(ff_dim, activation="relu"), Dense(embed_dim),]
)
self.layernorm1 = tf.keras.layers.LayerNormalization(epsilon=1e-6)
self.layernorm2 = tf.keras.layers.LayerNormalization(epsilon=1e-6)
self.dropout1 = tf.keras.layers.Dropout(rate)
self.dropout2 = tf.keras.layers.Dropout(rate)
def call(self, inputs, training):
attn_output = self.att(inputs)
attn_output = self.dropout1(attn_output, training=training)
out1 = self.layernorm1(inputs + attn_output)
ffn_output = self.ffn(out1)
ffn_output = self.dropout2(ffn_output, training=training)
return self.layernorm2(out1 + ffn_output)
```
#### 定义嵌入层
对于文本分类任务而言,在将单词送入 Transformer 模型之前先通过嵌入层是非常重要的一步。这会把离散的词汇映射到连续向量空间中去。
```python
class TokenAndPositionEmbedding(Layer):
def __init__(self, maxlen, vocab_size, embed_dim):
super(TokenAndPositionEmbedding, self).__init__()
self.token_emb = Embedding(input_dim=vocab_size, output_dim=embed_dim)
self.pos_emb = Embedding(input_dim=maxlen, output_dim=embed_dim)
def call(self, x):
maxlen = tf.shape(x)[-1]
positions = tf.range(start=0, limit=maxlen, delta=1)
positions = self.pos_emb(positions)
x = self.token_emb(x)
return x + positions
```
#### 下载和准备数据集
这里以 IMDb 数据集为例说明如何下载并准备好用于训练的数据集。
```python
max_features = 20000 # 只考虑最常见的前两万个词
max_len = 200 # 将每条评论截断或填充至长度为 max_len
(x_train, y_train), (x_val, y_val) = keras.datasets.imdb.load_data(num_words=max_features)
print(len(x_train), "Training sequences")
print(len(x_val), "Validation sequences")
x_train = keras.preprocessing.sequence.pad_sequences(x_train, maxlen=max_len)
x_val = keras.preprocessing.sequence.pad_sequences(x_val, maxlen=max_len)
```
#### 创建基于 Transformer 的分类器模型
现在有了所有的组件之后就可以组合起来形成最终的分类器了。
```python
embed_dim = 32 # 插件维度
num_heads = 2 # 注意力头的数量
ff_dim = 32 # FeedForward网络中的隐藏层单元数
inputs = Input(shape=(max_len,))
embedding_layer = TokenAndPositionEmbedding(max_len, max_features, embed_dim)
x = embedding_layer(inputs)
transformer_block = TransformerBlock(embed_dim, num_heads, ff_dim)
x = transformer_block(x)
x = GlobalAveragePooling1D()(x)
x = Dropout(0.1)(x)
x = Dense(20, activation="relu")(x)
x = Dropout(0.1)(x)
outputs = Dense(2, activation="softmax")(x)
model = Model(inputs=inputs, outputs=outputs)
```
#### 训练与评估
完成上述工作后即可编译并开始训练过程。
```python
model.compile(optimizer='adam', loss='sparse_categorical_crossentropy', metrics=['accuracy'])
history = model.fit(
x_train, y_train,
batch_size=32,
epochs=2,
validation_data=(x_val, y_val)
)
```
阅读全文
相关推荐
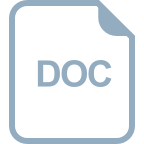
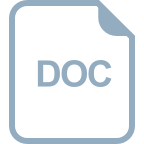
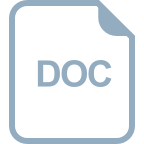

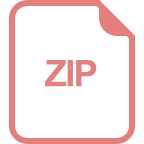
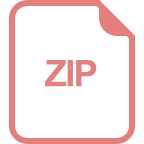












