reproducing kernel hilbert spaces in probability and statistics
时间: 2023-09-20 12:01:56 浏览: 122
在概率统计中,再生核Hilbert空间(RKHS)是一种非常重要的概念。它是一种特殊类型的函数空间,与概率密度函数(PDF)和随机过程分布函数(CDF)的分析有关。
首先,再生核是一种核函数,它是定义在输入空间上的一个函数。它可以通过计算两个输入值之间的相似性来测量它们之间的距离或相关性。
再生核Hilbert空间是由基于再生核的一组函数构成的函数空间。这些函数可以用于表示概率密度函数或随机过程的分布函数。
在概率统计中,我们经常使用RKHS来描述变量之间的关系,通过计算核函数得到变量之间的相似性。例如,在回归分析中,我们可以使用RKHS来建立输入和输出之间的非线性关系,这有助于捕捉数据中的非线性模式。
RKHS在概率统计中有广泛的应用。其中一个重要的应用是核密度估计。通过在RKHS中估计概率密度函数,我们可以通过观察到的样本数据来推断未观察到的数据点的概率分布。
另一个应用是随机过程的建模。通过在RKHS中建立随机过程的分布函数,我们可以推断未来观察到的值的概率分布,并对未来的随机过程行为进行预测。
总的来说,再生核Hilbert空间在概率统计中是一个非常有用的工具。它允许我们在非线性情况下处理随机变量之间的关系,并通过估计概率密度函数和建模随机过程的分布函数来进行推断和预测。
相关问题
Recall that to solve (P2) in the tth time frame, we observe ξt {hti, Qi(t), Yi(t)}Ni=1, consisting of the channel gains {hti}Ni=1 and the system queue states {Qi(t),Yi(t)}Ni=1, and accordingly decide the control action {xt, yt}, including the binary offloading decision xt and the continuous resource allocation yt τit, fit, eti,O, rit,ONi=1. A close observation shows that although (P2) is a non-convex optimization problem, the resource allocation problem to optimize yt is in fact an “easy” convex problem if xt is fixed. In Section IV.B, we will propose a customized algorithm to efficiently obtain the optimal yt given xt in (P2). Here, we denote Gxt,ξt as the optimal value of (P2) by optimizing yt given the offloading decision xt and parameter ξt. Therefore, solving (P2) is equivalent to finding the optimal offloading decision (xt)∗, where (P3) : xt∗ = arg maximize G xt, ξt . (20) xt ∈{0,1}N In general, obtaining (xt)∗ requires enumerating 2N offloading decisions, which leads to significantly high computational complexity even when N is moderate (e.g., N = 10). Other search based methods, such as branch-and-bound and block coordinate descent [29], are also time-consuming when N is large. In practice, neither method is applicable to online decision- making under fast-varying channel condition. Leveraging the DRL technique, we propose a LyDROO algorithm to construct a policy π that maps from the input ξt to the optimal action (xt)∗, i.e., π : ξt → (xt)∗, with very low complexity, e.g., tens of milliseconds computation time (i.e., the time duration from observing ξt to producing a control action {xt, yt}) when N = 10深度强化学习的动作是什么
在这个问题中,深度强化学习的动作是指在给定观测值(如题目中的 ξt)的情况下,通过一个策略函数(如题目中提到的 π)来映射到最优的控制动作(如题目中的 (xt)∗)。也就是说,深度强化学习的动作是指根据当前的状态和策略,来做出最优的决策。在这个问题中,最优的决策是指在给定的约束条件下,能够最大化目标函数(如题目中的 G xt, ξt)。
runtimeerror: expected to have finished reduction in the prior iteration before starting a new one. this error indicates that your module has parameters that were not used in producing loss. you can enable unused parameter detection by (1) passing the keyword argument `find_unused_parameters=true` to `torch.nn.parallel.distributeddataparallel`; (2) making sure all `forward` function outputs participate in calculating loss. if you already have done the above two steps, then the distributed data parallel module wasn't able to locate the output tensors in the return value of your module's `forward` function. please include the loss function and the structure of the return value of `forward` of your module when reporting this issue (e.g. list, dict, iterable).
### 回答1:
这个错误提示意味着你的模块有一些未被用于计算损失的参数。你可以通过两种方式解决这个问题:第一种是在`torch.nn.parallel.distributeddataparallel`函数中传递`find_unused_parameters=true`关键字参数,让程序检测未使用的参数;第二种是确保所有的`forward`函数输出都参与计算损失。如果你已经完成了以上两个步骤,但仍然出现这个错误,则说明分布式数据并行模块无法找到模块`forward`函数的输出张量的位置。在报告这个问题时,请包括损失函数和模块`forward`函数的返回值结构(如列表、字典、可迭代对象)。
### 回答2:
这个错误提示表明你的模块中存在一些参数没有被用于产生loss。如果你使用了`torch.nn.parallel.distributeddataparallel`,你可以通过传递关键字参数`find_unused_parameters=true` 来启用未使用参数检测功能。另外需要确保所有`forward`函数的输出都与loss有关。如果你已经完成了上述两个步骤,那么可能是分布式数据并行模块无法在返回值中找到`forward`函数的输出张量。在报告此问题时,请包括`loss`函数和`forward`函数返回值的结构(例如列表,字典,可迭代对象等)。
### 回答3:
运行时错误:在先前的迭代中完成了减少操作,然后才开始新操作。此错误表示您的模块具有未用于生成损失的参数。您可以通过以下方式启用未使用的参数检测:(1)向torch.nn.parallel.distributeddataparallel传递关键字参数“find_unused_parameters=true”;(2)确保所有“forward”函数输出参与计算损失。如果您已经完成了上述两个步骤,则分布式数据并行模块无法在模块的“forward”函数的返回值中定位输出张量。请在报告此问题时包括损失函数和模块的“forward”函数返回值的结构(例如,列表,字典,可迭代对象)。
此错误通常在使用分布式数据并行训练模型时出现。在分布式数据并行训练中,模型参数通过多个进程分配,并在每个进程中独立更新。在进行此操作时,所有进程必须在同步点达成一致,以确保所有参数在同步点之前更新。否则,将会出现上述错误。
要解决此错误,您可以尝试以下步骤:
1.检查模型的参数是否都参与了生成损失,如果有未使用的参数,可以通过将关键字参数“find_unused_parameters=true”传递给torch.nn.parallel.distributeddataparallel以启用未使用的参数检测。
2.确保所有“forward”函数的输出都与计算损失相关联。
3.如果您已经完成了上述两个步骤,则分布式数据并行模块无法定位输出张量。请检查模型的损失函数和“forward”函数的返回值结构,以确保输出张量位于返回值中,随后使用正确的同步点。如果无法解决问题,可以在报告此问题时将模型的相应代码和数据提供给支持团队,以便他们更好地理解和解决问题。
阅读全文
相关推荐
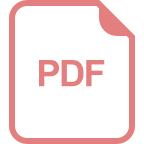
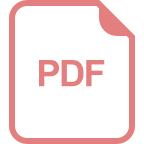
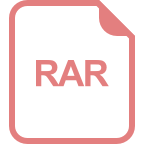
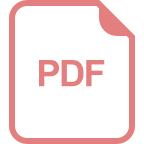
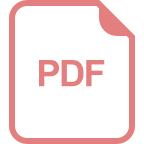
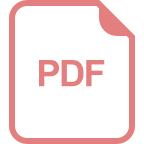
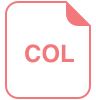
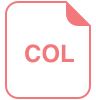
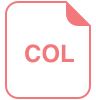
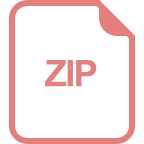
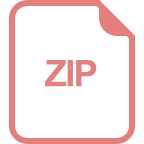