要使用粒子群优化(PSO)算法来求解给定函数$y=f(x_{1}, x_{2})=x_{1}^{2}+x_{2}$的最小值,其中$-10 \leq x_{1}, x_{2} \leq 10$,我们需要编写一个程序来实现PSO算法
时间: 2024-10-20 08:12:45 浏览: 35
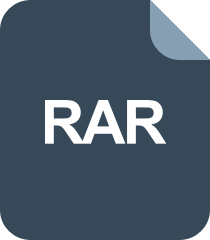
pso.rar_PSO_c 粒子群算法_pso vc_粒子群算法
粒子群优化(Particle Swarm Optimization, PSO)是一种模拟鸟群觅食行为的搜索算法,用于解决优化问题。为了找到函数 $f(x_1, x_2) = x_1^2 + x_2$ 的最小值,我们可以按照以下步骤编写一个基本的Python程序:
1. **初始化粒子**:
- 创建一组粒子,每个粒子代表一组可能的解 $(x_1, x_2)$,通常有固定大小的粒子群体和随机初始位置在给定范围内。
2. **设置参数**:
- 设置学习因子 $\omega$ (inertia weight),惯性作用;
- 设置认知权重 $c_1$ 和协作权重 $c_2$,影响个体探索和整体协作;
- 设定最大迭代次数或精度阈值。
3. **评估适应度**:
- 计算每个粒子当前位置的函数值 $f(x_1, x_2)$,作为其适应度。
4. **全局最佳和个人最佳**:
- 找到整个群体中的全局最优解(全局最低点)和每个粒子的局部最优解。
5. **更新速度和位置**:
- 根据粒子的速度、当前位置、个人最佳位置以及全局最佳位置更新粒子的位置,采用公式:
```
v_i(t+1) = \omega * v_i(t) + c_1 * r_1 * (pbest_i - x_i(t)) + c_2 * r_2 * (gbest - x_i(t))
x_i(t+1) = x_i(t) + v_i(t+1)
```
其中,$r_1$ 和 $r_2$ 是均匀分布的随机数。
6. **循环迭代**:
- 检查是否达到终止条件(如达到最大迭代次数),若未满足,则返回步骤4;否则,返回全局最佳解。
以下是一个简化的Python实现示例:
```python
import numpy as np
def f(x):
return x[0]**2 + x[1]
class ParticleSwarmOptimizer:
def __init__(self, n_particles, bounds, max_iter, w=0.7, c1=2, c2=2, debug=False):
self.n_particles = n_particles
self.bounds = bounds
self.max_iter = max_iter
self.w = w
self.c1 = c1
self.c2 = c2
self.debug = debug
self.particles = self.initialize_particles()
def initialize_particles(self):
# 粒子位置和速度初始化
particles = np.random.uniform(self.bounds[0], self.bounds[1], size=(self.n_particles, 2))
velocities = np.zeros_like(particles)
pbest = particles.copy()
gbest = np.array([np.inf, np.inf])
return particles, velocities, pbest, gbest
def optimize(self):
for t in range(self.max_iter):
if self.debug:
print(f"Iteration {t}:")
for i in range(self.n_particles):
r1, r2 = np.random.rand(2)
new_velocity = self.w * velocities[i] + \
self.c1 * r1 * (pbest[i] - particles[i]) + \
self.c2 * r2 * (gbest - particles[i])
new_position = np.clip(particles[i] + new_velocity, self.bounds[0], self.bounds[1])
fitness_new = f(new_position)
# 更新适应度和最优解
if fitness_new < f(particles[i]):
velocities[i] = new_velocity
particles[i] = new_position
if fitness_new < f(pbest[i]):
pbest[i] = new_position
# 更新全局最优解
if fitness_new < f(gbest):
gbest = new_position
return gbest, pbest[-1]
# 使用
optimizer = ParticleSwarmOptimizer(n_particles=50, bounds=(-10, 10), max_iter=1000)
solution, best_fitness = optimizer.optimize()
print(f"Best solution found: {solution} with a minimum value of {best_fitness}")
```
阅读全文
相关推荐
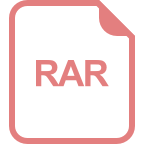
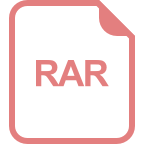
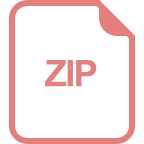
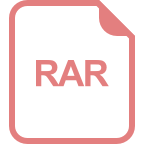
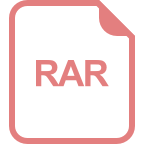
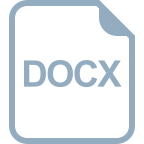
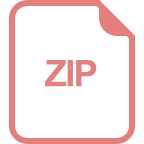
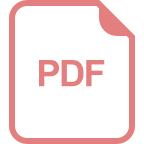
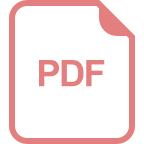
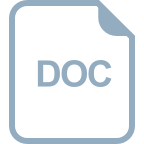
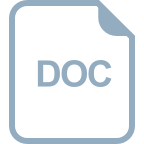
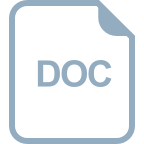
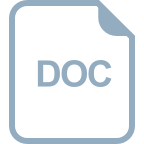
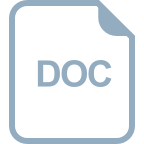
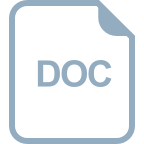
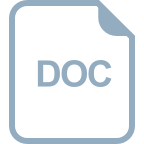

