def _normalize_idx(self, idx): nidx = idx if nidx < 0: nidx += len(self) if nidx < 0: nidx = 0 return nidx def __getitem__(self, idx): """Implements `x = self[idx]`""" assert(isinstance(idx, int)) nidx = self._normalize_idx(idx) if nidx >= self.length: raise IndexError else: #start at head, skip the NONE sentinel, now at index 0, while loop to walk to nidx, return data # or figure out closer to head or head.prior(tail), make the walk shorter if idx< (self.length/2): #start at head.next, walk forward idx number of nodes current=self.head.next count=0 while count<nidx: current=current.next count+=1 else: #start at head.prior, walk backward len(self.data)-idx-1 number of nodes current=self.head.prior count=self.length-1 while count>nidx: current=current.prior count-=1 return current.val 改写代码风格并保持变量名,代码意思不变
时间: 2024-01-12 21:05:45 浏览: 118
下面是改写后的代码风格:
```
def _normalize_idx(self, idx):
nidx = idx
if nidx < 0:
nidx += len(self)
if nidx < 0:
nidx = 0
return nidx
def __getitem__(self, idx):
"""Implements `x = self[idx]`"""
assert(isinstance(idx, int))
nidx = self._normalize_idx(idx)
if nidx >= self.length:
raise IndexError
else:
# Start at head, skip the NONE sentinel, now at index 0, while loop to walk to nidx, return data
# Or figure out closer to head or head.prior(tail), make the walk shorter
if idx < (self.length/2): # Start at head.next, walk forward idx number of nodes
current = self.head.next
count = 0
while count < nidx:
current = current.next
count += 1
else: # Start at head.prior, walk backward len(self.data)-idx-1 number of nodes
current = self.head.prior
count = self.length-1
while count > nidx:
current = current.prior
count -= 1
return current.val
```
注意,变量名没有改变,只是对代码进行了一些格式化和注释。
相关推荐
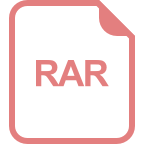
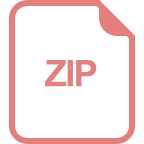
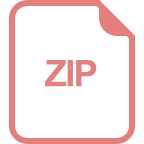














