def _grow_tree(self, X, y, depth=0): num_samples_per_class = [np.sum(y == i) for i in range(self.n_classes_)] predicted_class = np.argmax(num_samples_per_class) node = Node(predicted_class=predicted_class) if depth < self.max_depth: idx, thr = self._best_split(X, y) if idx is not None: indices_left = X[:, idx] < thr X_left, y_left = X[indices_left], y[indices_left] X_right, y_right = X[~indices_left], y[~indices_left] node.feature_index = idx node.threshold = thr node.left = self._grow_tree(X_left, y_left, depth + 1) node.right = self._grow_tree(X_right, y_right, depth + 1) return node def _predict(self, inputs): node = self.tree_ while node.left: if inputs[node.feature_index] < node.threshold: node = node.left else: node = node.right return node.predicted_class class Node: def __init__(self, *, predicted_class): self.predicted_class = predicted_class self.feature_index = 0 self.threshold = 0 self.left = None self.right = None解释这段代码
时间: 2024-02-15 16:28:53 浏览: 193
这段代码实现了分类树的核心功能,其中包括两个方法和一个类:
1. `_grow_tree(self, X, y, depth=0)`:生成决策树的方法,其中 `X` 是输入数据的特征矩阵,`y` 是对应的类别标签,`depth` 是当前节点的深度。它首先统计每个类别在当前节点中的数量,然后计算出数量最多的类别作为当前节点的预测类别。如果当前节点深度还未达到最大深度,则调用 `_best_split` 方法找到最佳分裂点,然后根据分裂点将当前节点分裂成左右两个子节点,分别递归调用 `_grow_tree` 方法生成左右子树。最后,返回当前节点。
2. `_predict(self, inputs)`:根据输入数据进行分类的方法,其中 `inputs` 是用于分类的输入特征向量。它使用当前节点的特征索引和阈值判断输入数据应该进入左子树还是右子树,直到找到叶子节点为止,最终返回叶子节点的预测类别。
3. `Node` 类:表示分类树的一个节点,其中包含预测类别、特征索引、阈值、左子节点和右子节点等属性。
总体来说,这段代码实现了一个基本的分类树,可以用于对输入数据进行分类。但是,它还有一些需要改进的地方,比如可以考虑使用其他的分裂指标、处理缺失值等。
相关问题
python决策树算法代码_决策树的Python实现(含代码)
下面是一份使用Python实现决策树算法的代码:
```python
import numpy as np
def entropy(y):
"""计算熵"""
_, counts = np.unique(y, return_counts=True)
p = counts / len(y)
return -np.sum(p * np.log2(p))
class DecisionTree:
def __init__(self, max_depth=None):
self.max_depth = max_depth
def fit(self, X, y):
self.n_features_ = X.shape[1]
self.tree_ = self._grow_tree(X, y)
def predict(self, X):
return [self._predict(inputs) for inputs in X]
def _best_split(self, X, y):
"""找到最好的特征和分割点"""
m = y.size
if m <= 1:
return None, None
num_parent = [np.sum(y == c) for c in range(self.n_classes_)]
best_gini = 1.0 - sum((n / m) ** 2 for n in num_parent)
best_idx, best_thr = None, None
for idx in range(self.n_features_):
thresholds, classes = zip(*sorted(zip(X[:, idx], y)))
num_left = [0] * self.n_classes_
num_right = num_parent.copy()
for i in range(1, m):
c = classes[i - 1]
num_left[c] += 1
num_right[c] -= 1
gini_left = 1.0 - sum((num_left[x] / i) ** 2 for x in range(self.n_classes_))
gini_right = 1.0 - sum((num_right[x] / (m - i)) ** 2 for x in range(self.n_classes_))
gini = (i * gini_left + (m - i) * gini_right) / m
if thresholds[i] == thresholds[i - 1]:
continue
if gini < best_gini:
best_gini = gini
best_idx = idx
best_thr = (thresholds[i] + thresholds[i - 1]) / 2
return best_idx, best_thr
def _grow_tree(self, X, y, depth=0):
"""递归地构建决策树"""
num_samples_per_class = [np.sum(y == i) for i in range(self.n_classes_)]
predicted_class = np.argmax(num_samples_per_class)
node = Node(
predicted_class=predicted_class,
num_samples=len(y),
num_samples_per_class=num_samples_per_class,
)
# 停止递归条件
if (
depth < self.max_depth
and np.unique(y).size > 1
and X.shape[0] > self.min_samples_split
):
idx, thr = self._best_split(X, y)
if idx is not None:
indices_left = X[:, idx] < thr
X_left, y_left = X[indices_left], y[indices_left]
X_right, y_right = X[~indices_left], y[~indices_left]
node.feature_index = idx
node.threshold = thr
node.left = self._grow_tree(X_left, y_left, depth + 1)
node.right = self._grow_tree(X_right, y_right, depth + 1)
return node
def _predict(self, inputs):
"""预测一个样本"""
node = self.tree_
while node.left:
if inputs[node.feature_index] < node.threshold:
node = node.left
else:
node = node.right
return node.predicted_class
class Node:
def __init__(self, *, predicted_class, num_samples, num_samples_per_class):
self.predicted_class = predicted_class
self.num_samples = num_samples
self.num_samples_per_class = num_samples_per_class
self.feature_index = 0
self.threshold = 0
self.left = None
self.right = None
```
这份代码实现了基于 Gini 系数的分类决策树算法。其中 `max_depth` 参数表示树的最大深度,`min_samples_split` 参数表示一个节点至少需要包含多少个样本才能进行分裂。使用时,可以按照下面的方式实例化并调用:
```python
clf = DecisionTree(max_depth=3)
clf.fit(X_train, y_train)
y_pred = clf.predict(X_test)
```
其中 `X_train` 和 `y_train` 分别表示训练集的特征和标签,`X_test` 表示测试集的特征。
在不用sklearn库的情况下写一个决策树代码
当然可以!以下是一个简单的决策树的实现代码示例:
```python
class DecisionTree:
def __init__(self, max_depth=None):
self.max_depth = max_depth
def fit(self, X, y):
self.X = X
self.y = y
self.n_features = X.shape[1]
self.n_classes = len(set(y))
self.tree = self._grow_tree()
def _grow_tree(self, depth=0):
num_samples_per_class = [np.sum(self.y == i) for i in range(self.n_classes)]
predicted_class = np.argmax(num_samples_per_class)
node = {'predicted_class': predicted_class}
if depth < self.max_depth:
best_gain = 0.0
best_feature = None
best_threshold = None
for feature in range(self.n_features):
unique_values = set(self.X[:, feature])
for threshold in unique_values:
gain = self._information_gain(feature, threshold)
if gain > best_gain:
best_gain = gain
best_feature = feature
best_threshold = threshold
if best_gain > 0.0:
left_indices = self.X[:, best_feature] <= best_threshold
right_indices = ~left_indices
node['feature'] = best_feature
node['threshold'] = best_threshold
node['left'] = self._grow_tree(depth + 1)
node['right'] = self._grow_tree(depth + 1)
return node
def _information_gain(self, feature, threshold):
parent_entropy = self._entropy(self.y)
left_indices = self.X[:, feature] <= threshold
right_indices = ~left_indices
left_entropy = self._entropy(self.y[left_indices])
right_entropy = self._entropy(self.y[right_indices])
n = len(self.y)
left_weight = len(self.y[left_indices]) / n
right_weight = len(self.y[right_indices]) / n
information_gain = parent_entropy - (left_weight * left_entropy + right_weight * right_entropy)
return information_gain
def _entropy(self, y):
class_counts = np.bincount(y)
probabilities = class_counts / len(y)
entropy = 0.0
for prob in probabilities:
if prob > 0:
entropy -= prob * np.log2(prob)
return entropy
def predict(self, X):
return np.array([self._traverse_tree(x, self.tree) for x in X])
def _traverse_tree(self, x, node):
if 'predicted_class' in node:
return node['predicted_class']
else:
if x[node['feature']] <= node['threshold']:
return self._traverse_tree(x, node['left'])
else:
return self._traverse_tree(x, node['right'])
```
这是一个基本的决策树实现,其中没有使用任何sklearn库。你可以使用`fit`方法训练决策树模型,然后使用`predict`方法对新的样本进行预测。注意,这个实现是一个简化版本,可能不具备sklearn中决策树的所有功能和优化。
阅读全文
相关推荐


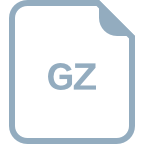













