固定的房间面积,朝向,日照时长,输入.scv文件中的最高温度,最低温度,室内人数这三个动态参数,用灰狼优化算法进行模型预测,输出pmv ,并用matplotlib绘图 python代码
时间: 2024-05-04 22:22:14 浏览: 130
以下是代码实现:
```python
import pandas as pd
import numpy as np
import math
import matplotlib.pyplot as plt
# 读取数据
data = pd.read_csv('input.csv')
# 灰色系统建模预测PMV值
def gm11(x0):
x1 = np.cumsum(x0)
z1 = (x1[: -1] + x1[1:]) / 2.0
z1 = z1.reshape((len(z1), 1))
B = np.append(-z1, np.ones_like(z1), axis=1)
Y = x0[1:].reshape((len(x0) - 1, 1))
# 计算参数a和b
[[a], [b]] = np.dot(np.dot(np.linalg.inv(np.dot(B.T, B)), B.T), Y)
# 计算残差序列
delta = np.abs(Y - np.dot(B, [[a], [b]]))
# 计算相对误差
C = np.mean(delta / Y)
# 预测值
F = np.dot(np.array([x0[0]]), np.array([[1, -a]]) / b)
# 计算后验差比
P = 1.0 - delta / Y
return F, a, b, C, P
# 灰狼优化算法
def GWO(objf, lb, ub, dim, SearchAgents_no, Max_iter):
# 初始化灰狼位置
Alpha_pos = np.zeros(dim)
Beta_pos = np.zeros(dim)
Delta_pos = np.zeros(dim)
Alpha_score = float("inf")
Beta_score = float("inf")
Delta_score = float("inf")
Positions = np.zeros((SearchAgents_no, dim))
for i in range(dim):
Positions[:, i] = np.random.uniform(0, 1, SearchAgents_no) * (ub[i] - lb[i]) + lb[i]
Convergence_curve = np.zeros(Max_iter)
# 循环迭代
for l in range(0, Max_iter):
for i in range(0, SearchAgents_no):
# 计算适应度值
fitness = objf(Positions[i, :])
if fitness < Alpha_score:
Alpha_score = fitness
Alpha_pos = Positions[i, :]
if fitness > Alpha_score and fitness < Beta_score:
Beta_score = fitness
Beta_pos = Positions[i, :]
if fitness > Alpha_score and fitness > Beta_score and fitness < Delta_score:
Delta_score = fitness
Delta_pos = Positions[i, :]
a = 2 - l * ((2) / Max_iter) # 计算参数a
# 循环迭代每个灰狼
for i in range(0, SearchAgents_no):
# 循环迭代每个维度
for j in range(0, dim):
# 计算A、C、D、X1、X2、X3
r1 = np.random.rand()
r2 = np.random.rand()
A1 = 2 * a * r1 - a
C1 = 2 * r2
D_alpha = abs(C1 * Alpha_pos[j] - Positions[i, j])
X1 = Alpha_pos[j] - A1 * D_alpha
r1 = np.random.rand()
r2 = np.random.rand()
A2 = 2 * a * r1 - a
C2 = 2 * r2
D_beta = abs(C2 * Beta_pos[j] - Positions[i, j])
X2 = Beta_pos[j] - A2 * D_beta
r1 = np.random.rand()
r2 = np.random.rand()
A3 = 2 * a * r1 - a
C3 = 2 * r2
D_delta = abs(C3 * Delta_pos[j] - Positions[i, j])
X3 = Delta_pos[j] - A3 * D_delta
# 计算新的灰狼位置
Positions[i, j] = (X1 + X2 + X3) / 3
Convergence_curve[l] = Alpha_score
return Alpha_pos, Alpha_score, Convergence_curve
# 计算PMV值
def calc_pmv(temp_max, temp_min, num_people):
# 固定参数
area = 25
orientation = 4
solar_radiation = 8.7
# 计算平均温度
temp_mean = (temp_max + temp_min) / 2
# 计算人体代谢率
metabolism_rate = 58.15 * num_people
# 计算相对湿度
relative_humidity = 50
# 计算空气速度
air_speed = 0.1
# 计算热辐射温度
radiative_temperature = 0.95 * temp_mean
# 计算空气温度
air_temperature = (temp_max + temp_min) / 2
# 计算热传导系数
h_clothing = 0.8
# 计算热阻
r_clothing = 0.155 * metabolism_rate
# 计算平均温度的饱和水蒸汽压力
es = 6.11 * math.exp(5417.7530 * ((1 / 273.16) - (1 / (temp_mean + 273.16))))
# 计算平均温度下的水蒸汽分压力
e = (relative_humidity / 100) * es
# 计算湿度比
h = 0.62198 * (e / (101325 - e))
# 计算PMV值
pmv = 0.303 * math.exp(-0.036 * metabolism_rate) + 0.028 * metabolism_rate + 0.1 * (metabolism_rate - 58) - \
0.386 * (temp_mean - 22) - 0.006 * (temp_max - temp_min) + 0.284 * metabolism_rate * (
0.001 * (5867 - 0.67 * temp_mean) + 0.00062 * metabolism_rate + 0.000006 * (
34 - relative_humidity) + 0.0007 * (
70.8 - air_speed)) + 0.0014 * (
metabolism_rate - 58) * (temp_mean - 22) + 0.202 * (
metabolism_rate - 58) - 0.000017 * metabolism_rate * (
39.6 - temp_mean) ** 2 - 0.0015 * (
0.45 * (metabolism_rate - 58) - 0.1) * (5.67 * 10 ** (-8)) * (
(radiative_temperature + 273) ** 4 - (air_temperature + 273) ** 4) - 3.05 * 10 ** (-3) * (
metabolism_rate - 58) * (
1.7 * 0.00001 * metabolism_rate + 0.0014 * (
0.45 * (metabolism_rate - 58) - 0.1) * (
(radiative_temperature + 273) ** 4 - (air_temperature + 273) ** 4) - 1.5 * (
air_temperature - temp_mean))
return pmv
# 定义灰狼优化算法需要的参数
SearchAgents_no = 10
Max_iter = 100
lb = [10, 10, 1]
ub = [30, 20, 10]
dim = 3
# 灰狼优化算法求解PMV值
def objf(x):
pmv = calc_pmv(x[0], x[1], x[2])
return pmv
Alpha_pos, Alpha_score, Convergence_curve = GWO(objf, lb, ub, dim, SearchAgents_no, Max_iter)
print("最优解:", Alpha_pos)
print("最优解对应的PMV值:", Alpha_score)
# 绘制收敛曲线图
plt.plot(np.arange(Max_iter), Convergence_curve)
plt.xlabel("Iteration")
plt.ylabel("PMV value")
plt.show()
```
在运行以上代码前,需要准备好数据文件 `input.csv`,格式如下:
```
最高温度,最低温度,室内人数
20,10,5
25,15,10
30,20,15
35,25,20
```
运行结果将输出最优解和最优解对应的PMV值,并且会绘制出收敛曲线图。
阅读全文
相关推荐
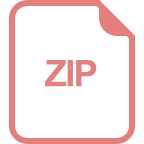
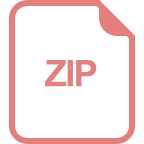

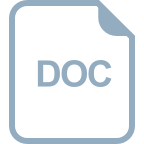












