System overview analysis of the computer mall system paper, no less than 300 words
时间: 2023-03-24 18:03:27 浏览: 67
非常感谢您的提问。关于计算机商城系统的系统概述分析,我可以为您提供以下的回答。
计算机商城系统是一个基于互联网的电子商务平台,旨在为消费者提供方便快捷的购物体验。该系统主要由前台展示页面、后台管理系统、订单管理系统、支付系统、物流系统等模块组成。
前台展示页面是用户访问商城的入口,通过该页面用户可以浏览商品、下单购买等。后台管理系统是商城管理员的工作台,通过该系统管理员可以管理商品、订单、用户等信息。订单管理系统是商城处理订单的核心模块,通过该系统可以实现订单的生成、修改、取消等操作。支付系统是商城的收款模块,通过该系统可以实现在线支付、货到付款等多种支付方式。物流系统是商城的配送模块,通过该系统可以实现订单的发货、物流跟踪等功能。
总体来说,计算机商城系统的设计目标是为用户提供便捷的购物体验,为商家提供高效的销售渠道。该系统的核心功能包括商品展示、订单管理、支付收款、物流配送等,通过这些功能的有机结合,实现了一个完整的电子商务平台。
相关问题
an overview of statistical learning theory
统计学习理论是一种研究机器学习算法的理论基础,它主要关注于如何在给定数据集合的情况下,通过统计方法来构建模型,并在新的数据点上进行预测。这个领域包括多种模型和方法,包括支持向量机、决策树、神经网络等。统计学习理论不仅涉及概率论和统计学的知识,还需要涉及计算机科学,特别是算法和数据结构方面的知识。
an overview of gradient descent optimization algorithms
梯度下降优化算法概述
梯度下降是一种常用的优化方法,可以帮助我们找到使目标函数最小化或最大化的参数。随着机器学习和深度学习的发展,各种梯度下降算法也不断涌现。以下是一些常用的梯度下降优化算法的概述:
1. 批量梯度下降(Batch Gradient Descent):在每次迭代中,批量梯度下降使用所有样本的梯度来更新模型参数。适用于训练集较小、模型参数较少的情况。
2. 随机梯度下降(Stochastic Gradient Descent):在每次迭代中,随机梯度下降使用一个单独的样本来更新模型参数。适用于训练集较大、模型参数较多的情况。
3. 小批量梯度下降(Mini-batch Gradient Descent):小批量梯度下降是一种介于批量梯度下降和随机梯度下降之间的方法。它在每次迭代中使用一小部分样本的梯度来更新模型参数。适用于训练集规模较大的情况。
4. 动量(Momentum):动量算法加入了“惯性”的概念,可以加速梯度下降的收敛速度。在每次迭代中,动量算法使用上一次的梯度信息来更新模型参数。
5. 自适应梯度下降(Adaptive Gradient Descent):自适应梯度下降可以自适应地调整每个模型参数的学习率,以便更快地收敛到最优解。比如,Adagrad算法可以针对每个参数单独地调整学习率。
6. 自适应矩估计(Adaptive Moment Estimation):Adam算法是一种结合了Momentum和Adaptive Gradient Descent的算法。它可以自适应调整每个参数的学习率,并利用二阶矩来调整动量。
每种梯度下降算法都有其适用的场合,需要根据问题的性质来选择合适的算法。
相关推荐
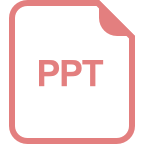
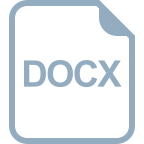
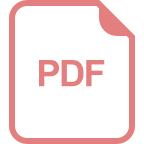












