openmv中的image.draw_keypoints(kpts)函数
时间: 2024-04-21 13:30:09 浏览: 24
在OpenMV中,image.draw_keypoints(kpts)函数也是用来在图像上绘制特征点的。它需要传入一个特征点列表kpts,其中每个特征点包含了其在图像上的坐标、尺度、方向等信息。函数会将特征点用圆形的形式绘制在图像上,以便于观察和分析。此外,OpenMV还提供了其他的绘制函数,如image.draw_line()、image.draw_rectangle()等,可用于在图像上绘制直线、矩形等形状。
相关问题
img.draw_keypoints([(blob.cx(), blob.cy(), int(math.degrees(blob.rotation())))], size=20) print(clock.fps())
引用\[1\]:img.draw_keypoints(\[(blob.cx(), blob.cy(), int(math.degrees(blob.rotation())))\], size=20)是用来绘制关键点的函数。它接受一个包含关键点信息的列表作为参数,每个关键点由(x, y, rotation)组成,其中(x, y)是关键点的坐标,rotation是关键点的旋转角度。size参数指定了绘制关键点的大小。\[1\]
print(clock.fps())是用来打印当前帧率的函数。它会输出当前相机的帧率。\[1\]
所以,这段代码的作用是绘制关键点并打印当前帧率。
#### 引用[.reference_title]
- *1* *3* [OpenMV的单颜色识别讲解](https://blog.csdn.net/qq_63922192/article/details/127149866)[target="_blank" data-report-click={"spm":"1018.2226.3001.9630","extra":{"utm_source":"vip_chatgpt_common_search_pc_result","utm_medium":"distribute.pc_search_result.none-task-cask-2~all~insert_cask~default-1-null.142^v91^insertT0,239^v3^insert_chatgpt"}} ] [.reference_item]
- *2* [K210追小球程序与STM32最小系统板通信(自主学习)](https://blog.csdn.net/qq_60043905/article/details/125655140)[target="_blank" data-report-click={"spm":"1018.2226.3001.9630","extra":{"utm_source":"vip_chatgpt_common_search_pc_result","utm_medium":"distribute.pc_search_result.none-task-cask-2~all~insert_cask~default-1-null.142^v91^insertT0,239^v3^insert_chatgpt"}} ] [.reference_item]
[ .reference_list ]
我对superpoint在github上发布的superpoint_v1.pth文件进行了修改,想提取原图像的特征点并绘制,修改的部分代码如下 : start1 = time.time() pts, desc, heatmap = fe.run(img) # 转换特征点为cv2.KeyPoint对象 cv_keypoints = [cv2.KeyPoint(point[0], point[1], 1) for point in pts[0]] image_color = cv2.cvtColor(img, cv2.COLOR_GRAY2BGR) # 绘制特征点 image_with_keypoints = cv2.drawKeypoints(image_color, cv_keypoints, None, flags=cv2.DRAW_MATCHES_FLAGS_DRAW_RICH_KEYPOINTS) cv2.imshow('Image with Keypoints', image_with_keypoints) cv2.waitKey(0) cv2.destroyAllWindows() 错误信息如下: Traceback (most recent call last): File "D:/SuperPointPretrainedNetwork-master/demo1.py", line 683, in <module> flags=cv2.DRAW_MATCHES_FLAGS_DRAW_RICH_KEYPOINTS) cv2.error: C:\projects\opencv-python\opencv\modules\features2d\src\draw.cpp:108: error: (-5) Incorrect type of input image. in function cv::drawKeypoints warning: Error opening file (/build/opencv/modules/videoio/src/cap_ffmpeg_impl.hpp:792) warning: ./assets/icl_snippet/ (/build/opencv/modules/videoio/src/cap_ffmpeg_impl.hpp:793) 我改如何修改
根据错误信息,问题出现在 `cv2.drawKeypoints` 函数中,错误提示是 "Incorrect type of input image"。这意味着 `image_color` 的类型不正确。
根据您提供的代码,`image_color` 是使用 `cv2.cvtColor` 函数从灰度图像转换为彩色图像的结果。但是,错误信息表明转换后的图像类型不正确。
您可以尝试使用以下方法解决该问题:
1. 确保 `img` 是正确的灰度图像。可以使用 `cv2.imread` 读取图像,并在读取时指定 `cv2.IMREAD_GRAYSCALE` 参数。
2. 使用 `cv2.cvtColor` 将读取的灰度图像转换为彩色图像时,确保正确指定转换的颜色空间。例如,使用 `cv2.COLOR_GRAY2BGR` 将灰度图像转换为 BGR 彩色图像。
3. 在调用 `cv2.drawKeypoints` 之前,检查 `image_color` 的类型,确保它是正确的彩色图像类型(例如,numpy 数组)。
尝试按照上述步骤检查和修改代码,并确保输入图像的类型正确,以解决该错误。
相关推荐
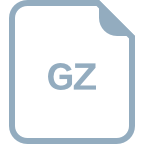













