def softmax(x): y = np.exp(x) / np.sum(np.exp(x)) return np.array(y) A=[0.3,2.9,4.0] y=softmax(A) print("归一化后的数组:", y) print("数组中所有元素的和:", sum(y))分析以上代码
时间: 2023-10-23 15:08:26 浏览: 72
以上代码实现了 softmax 函数,将输入的一维数组归一化为一个概率分布。具体来说,对于输入的一维数组 x,softmax 函数首先对其进行指数运算,然后将运算结果除以运算结果的总和,最终得到一个概率分布。
在代码中,输入的一维数组 A 为 [0.3, 2.9, 4.0],经过 softmax 函数处理后,得到的归一化后的数组为 [0.01821127, 0.24519181, 0.73659691],其中数组中所有元素的和为 1,符合概率分布的定义。
值得注意的是,由于指数运算可能会导致数值溢出,因此在实际应用中,需要进行数值稳定性处理,例如对输入的数组中的每个元素都减去数组中的最大值,从而避免指数运算过大。
相关问题
# coding: utf-8 import numpy as np def identity_function(x): return x def step_function(x): return np.array(x > 0, dtype=np.int) def sigmoid(x): return 1 / (1 + np.exp(-x)) def sigmoid_grad(x): return (1.0 - sigmoid(x)) * sigmoid(x) def relu(x): return np.maximum(0, x) def relu_grad(x): grad = np.zeros(x) grad[x>=0] = 1 return grad def softmax(x): if x.ndim == 2: x = x.T x = x - np.max(x, axis=0) y = np.exp(x) / np.sum(np.exp(x), axis=0) return y.T x = x - np.max(x) # 溢出对策 return np.exp(x) / np.sum(np.exp(x)) def mean_squared_error(y, t): return 0.5 * np.sum((y-t)**2) def cross_entropy_error(y, t): if y.ndim == 1: t = t.reshape(1, t.size) y = y.reshape(1, y.size) # 监督数据是one-hot-vector的情况下,转换为正确解标签的索引 if t.size == y.size: t = t.argmax(axis=1) batch_size = y.shape[0] return -np.sum(np.log(y[np.arange(batch_size), t] + 1e-7)) / batch_size def softmax_loss(X, t): y = softmax(X) return cross_entropy_error(y, t)
这是一个包含各种常用神经网络函数的 Python 代码。其中 identity_function 表示恒等函数,step_function 表示阶跃函数,sigmoid 和 sigmoid_grad 表示 sigmoid 函数及其导数,relu 和 relu_grad 表示 ReLU 函数及其导数,softmax 表示 softmax 函数,mean_squared_error 和 cross_entropy_error 表示均方误差损失函数和交叉熵损失函数,softmax_loss 表示将 softmax 函数和交叉熵损失函数合并成一个层。
这些函数在神经网络的训练和测试中都有重要作用。例如,sigmoid 函数常被用于神经网络中的神经元激活函数,用于二分类任务的预测;ReLU 函数则常被用于卷积神经网络中的卷积层激活函数,用于提取图像特征;softmax 函数则常被用于神经网络中的输出层激活函数,用于多分类任务的预测。损失函数则常被用于评估神经网络的性能,用于反向传播算法的求解。
解读这段代码 def value(self, x: np.ndarray) -> np.ndarray: ''' Parameters: x is the input to the softmax function. x is a two dimensional numpy array. Each row is the input to the softmax function Returns: output of the softmax function. The returned value is with the same shape as that of x. ''' #### write your code below #### x_max = np.max(x, axis=1, keepdims=True) exp_x = np.exp(x - x_max) softmax = exp_x / np.sum(exp_x, axis=1, keepdims=True) return softmax
这段代码定义了一个名为 `value()` 的函数,用于计算 softmax 函数的输出。
函数的输入参数 `x` 是一个二维的 numpy 数组,其中每一行表示 softmax 函数的输入。函数的返回值是 softmax 函数的输出,具有与输入 `x` 相同的形状。
在函数的实现中,首先使用 `np.max()` 函数计算 `x` 中每一行的最大值,并使用 `keepdims=True` 保持输出的维度与输入相同。然后,使用 `np.exp()` 计算 `x` 减去最大值后的指数值。这是为了避免指数溢出。
接下来,通过计算指数值除以每一行指数值的和,得到 softmax 函数的输出。使用 `np.sum()` 函数计算每一行指数值的和,并使用 `keepdims=True` 保持输出的维度与输入相同。
最后,将计算得到的 softmax 输出作为函数的返回值。
你可以调用这个函数,并传递一个二维数组作为输入,以计算 softmax 函数的输出。
阅读全文
相关推荐
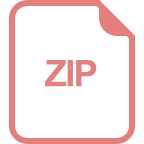



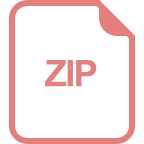
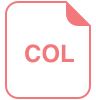
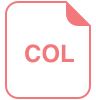
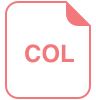






