loss为什么要用test_loss / (batch_idx + 1)进行计算
时间: 2024-05-30 19:11:09 浏览: 98
这是因为Loss是用来衡量模型预测与真实标签之间的误差的。在模型训练时,我们需要根据Loss的大小来进行参数调整,以使得模型的预测更加准确。而对于大数据集来说,每个epoch会有成千上万个batch需要计算Loss,因此在计算Loss时,将每个batch的Loss相加再求平均能够更好地衡量整个数据集的模型表现。所以,我们使用test_loss / (batch_idx + 1)来计算每个batch的平均Loss。
相关问题
total_test_loss = 0 for batch_idx, (imgL, imgR, disp_L) in enumerate(TestImgLoader): test_loss = test(imgL, imgR, disp_L) print('Iter %d test loss = %.3f' % (batch_idx, test_loss)) total_test_loss += test_loss print('total test loss = %.3f' % (total_test_loss / len(TestImgLoader)))什么意思
这段代码是在函数`main()`中的循环体外部,用于测试模型在测试集上的性能,并输出测试损失。具体含义如下:
- `total_test_loss = 0`:初始化测试损失为0。
- `for batch_idx, (imgL, imgR, disp_L) in enumerate(TestImgLoader):`:使用`TestImgLoader`迭代器依次获取测试数据,循环执行直到获取完所有测试数据。
- `test_loss = test(imgL, imgR, disp_L)`:调用`test()`函数在当前测试数据上进行测试,返回测试损失。
- `print('Iter %d test loss = %.3f' % (batch_idx, test_loss))`:输出当前测试数据的测试损失。
- `total_test_loss += test_loss`:将当前测试数据的测试损失累加到总的测试损失中。
- `print('total test loss = %.3f' % (total_test_loss / len(TestImgLoader)))`:输出所有测试数据的平均测试损失。
需要注意的是,这里的`test()`函数是用于测试模型的,根据上下文无法确定该函数的实现细节。同时,由于缺少函数`test()`的代码和变量定义,可能无法理解该代码的完整含义。
import idx2numpy import numpy as np from functions import * from two_layer_network import * #导入训练集和训练集对应的标签并将其初始化 X_train,T_train=idx2numpy.convert_from_file('emnist/emnist-letters-train-images-idx3-ubyte'),idx2numpy.convert_from_file('emnist/emnist-letters-train-labels-idx1-ubyte') X_train,T_train=X_train.copy(),T_train.copy() X_train=X_train.reshape((X_train.shape[0],-1)) T_train=T_train-1 T_train=np.eye(26)[T_train] #导入测试集和测试集对应的标签标签并将其初始化 X_test,T_test=idx2numpy.convert_from_file('emnist/emnist-letters-test-images-idx3-ubyte'),idx2numpy.convert_from_file('emnist/emnist-letters-test-labels-idx1-ubyte') X_test,T_test=X_test.copy(),T_test.copy() X_test=X_test.reshape((X_test.shape[0],-1)) T_test=T_test-1 T_test=np.eye(26)[T_test] network=TwoLayerNet(input_size=784,hidden_size=45,output_size=26) train_size=X_train.shape[0] batch_size=100 iters_num=100000 learning_rate=0.01 train_loss_list=[] train_acc_list=[] test_acc_list=[] iter_per_epoch=max(train_size/batch_size,1) for i in range(iters_num): batch_mask=np.random.choice(train_size,batch_size) X_batch=X_train[batch_mask] T_batch=T_train[batch_mask] #从数据集里抽取batch_size笔数据 #grad=network.numerical_gradient(X_batch,T_batch)(跑不出来,卡在None None) grad=network.gradient(X_batch,T_batch) #计算梯度 for key in ('W1','b1','W2','b2') : network.params[key]-=learning_rate*grad[key] #误差反向传播法调整参数 loss=network.loss(X_batch,T_batch) train_loss_list.append(loss) #记录学习过程 if i % iter_per_epoch==0: train_acc=network.accuracy(X_train,T_train) test_acc=network.accuracy(X_test,T_test) train_acc_list.append(train_acc) test_acc_list.append(test_acc) print(train_acc,test_acc) #调整学习率 if i > 0 and i % 1000 == 0 and i<3000: learning_rate *= 0.1 print("learning rate reduced to " + str(learning_rate)) print(network.params) print(train_acc_list[-1],test_acc_list[-1])如果我想存储参数,该怎么做
如果想要存储参数,可以使用Python内置的pickle模块。具体步骤如下:
1. 在代码中加入以下代码,将训练后的参数保存到文件中:
```
import pickle
with open("params.pkl", "wb") as f:
pickle.dump(network.params, f)
```
其中,"params.pkl"是你想要存储参数的文件名,network.params是你的神经网络的参数。
2. 在需要使用训练后的参数进行预测时,可以通过以下代码从文件中读取参数:
```
import pickle
with open("params.pkl", "rb") as f:
params = pickle.load(f)
network.params = params
```
其中,params是从文件中读取到的参数,将其赋给network.params即可。
阅读全文
相关推荐
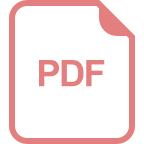
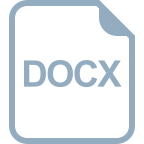
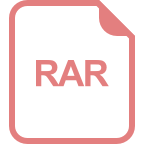














