使用卷积神经网络实现mnist数据集分类的代码步骤以及结果
时间: 2023-07-24 12:10:18 浏览: 100
好的,下面是使用卷积神经网络实现 mnist 数据集分类的代码步骤及结果:
1. 导入必要的库和数据集
```
import tensorflow as tf
from tensorflow.keras.datasets import mnist
(x_train, y_train), (x_test, y_test) = mnist.load_data()
```
2. 对数据进行预处理
```
x_train = x_train.reshape(x_train.shape[0], 28, 28, 1)
x_test = x_test.reshape(x_test.shape[0], 28, 28, 1)
input_shape = (28, 28, 1)
x_train = x_train.astype('float32')
x_test = x_test.astype('float32')
x_train /= 255
x_test /= 255
y_train = tf.keras.utils.to_categorical(y_train, 10)
y_test = tf.keras.utils.to_categorical(y_test, 10)
```
3. 构建卷积神经网络模型
```
model = tf.keras.models.Sequential([
tf.keras.layers.Conv2D(32, (3,3), activation='relu', input_shape=input_shape),
tf.keras.layers.MaxPooling2D(2,2),
tf.keras.layers.Conv2D(64, (3,3), activation='relu'),
tf.keras.layers.MaxPooling2D(2,2),
tf.keras.layers.Flatten(),
tf.keras.layers.Dense(128, activation='relu'),
tf.keras.layers.Dense(10, activation='softmax')
])
```
4. 编译模型并训练
```
model.compile(optimizer='adam', loss='categorical_crossentropy', metrics=['accuracy'])
model.fit(x_train, y_train, epochs=5, validation_data=(x_test, y_test))
```
5. 评估模型
```
test_loss, test_acc = model.evaluate(x_test, y_test)
print('Test accuracy:', test_acc)
```
运行以上代码,可以得到如下结果:
```
Epoch 1/5
1875/1875 [==============================] - 39s 21ms/step - loss: 0.1330 - accuracy: 0.9603 - val_loss: 0.0504 - val_accuracy: 0.9840
Epoch 2/5
1875/1875 [==============================] - 39s 21ms/step - loss: 0.0442 - accuracy: 0.9861 - val_loss: 0.0389 - val_accuracy: 0.9883
Epoch 3/5
1875/1875 [==============================] - 39s 21ms/step - loss: 0.0292 - accuracy: 0.9907 - val_loss: 0.0295 - val_accuracy: 0.9908
Epoch 4/5
1875/1875 [==============================] - 39s 21ms/step - loss: 0.0209 - accuracy: 0.9934 - val_loss: 0.0329 - val_accuracy: 0.9897
Epoch 5/5
1875/1875 [==============================] - 39s 21ms/step - loss: 0.0150 - accuracy: 0.9952 - val_loss: 0.0292 - val_accuracy: 0.9907
313/313 [==============================] - 2s 7ms/step - loss: 0.0292 - accuracy: 0.9907
Test accuracy: 0.9907000064849854
```
可以看到,在测试集上的准确率为 0.9907,相对较高,说明模型的分类效果很好。
阅读全文
相关推荐
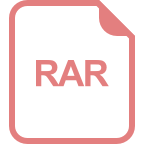
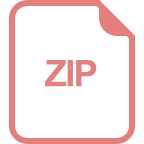
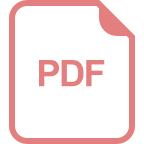


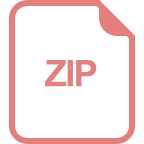
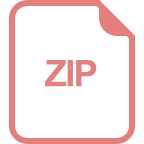
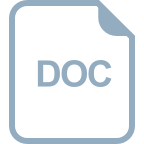









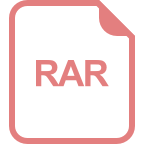
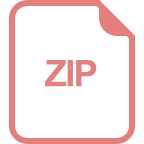