dijkstra 最短路径 python
时间: 2023-05-14 09:03:31 浏览: 146
Dijkstra算法是一种经典的图论算法,用于在带权图中找到最短路径。Python是一种非常流行的编程语言,也可以用来实现Dijkstra算法。
在Python中实现Dijkstra算法,需要首先使用一个图类来表示带权图。可以使用邻接列表或邻接矩阵来表示图。对于邻接列表,每个节点都有一个其它节点的列表,存储与该节点相邻的节点和对应的边权重。对于邻接矩阵,则是将节点之间的距离存储在矩阵中。
在实现Dijkstra算法之前,需要先定义一个优先队列来存储每个节点到起点的最短距离。可以使用Python内置的heapq库来实现基于堆的优先队列。
Dijkstra算法的实现步骤如下:
1. 初始化所有节点到起点的距离为无穷大,起点到起点的距离为0。
2. 将起点加入优先队列。
3. 从优先队列中取出距离起点最近的节点,更新该节点的相邻节点的距离。
4. 将所有可达的节点加入优先队列,并按照距离进行排序。
5. 重复步骤3和步骤4,直到优先队列为空或找到终点。
在Python中实现Dijkstra算法,可以使用一个列表来存储每个节点的最短距离,一个字典来存储每个节点对应的邻接列表,以及一个堆来实现优先队列。具体实现可以参考已有的Dijkstra算法代码。
相关问题
dijkstra最短路径算法python
### 回答1:
Dijkstra算法可以用Python来实现,它是一种用于计算从一个节点到另一个节点的最短路径的算法。以下是Python中实现Dijkstra算法的代码,用于寻找给定有向加权图中两个节点之间的最短路径:
```python
import heapq
def dijkstra(graph, start, end):
# 初始化距离字典
distances = {vertex: float('inf') for vertex in graph}
distances[start] = 0
# 初始化堆
heap = [(0, start)]
while heap:
# 取出堆顶节点
(current_distance, current_vertex) = heapq.heappop(heap)
# 如果当前节点已经处理过,则跳过
if current_distance > distances[current_vertex]:
continue
# 处理当前节点的邻居节点
for neighbor, weight in graph[current_vertex].items():
distance = current_distance + weight
# 如果新的距离更短,则更新距离字典并加入堆中
if distance < distances[neighbor]:
distances[neighbor] = distance
heapq.heappush(heap, (distance, neighbor))
# 返回起点到终点的最短距离
return distances[end]
```
其中,`graph` 是一个字典,表示有向加权图的邻接表,`start` 和 `end` 分别表示起点和终点的名称。该算法利用堆来实现优先队列,从而提高了处理效率。
### 回答2:
Dijkstra最短路径算法(Dijkstra's algorithm)是求解带权重图(Weighted Graph)中单源最短路径问题的一种经典算法。该算法最初由荷兰计算机科学家Edsger W.Dijkstra于1956年提出,属于贪心算法的一种。
在Python中,实现Dijkstra最短路径算法需要定义一个二元组(pair)集合来保存每个顶点的最短路径(distance)和前驱节点(previous),其中,distance表示从起始节点到该节点的距离,previous表示该节点的前驱节点。另外,需要一个任务集合(unvisited)来保存未被访问过的节点集合,并找出其中距离起点最近的点(minVertex)。
根据Dijkstra算法的思想,每次需要找到未访问过的点集中距离起点最近的那一个。然后,计算该点的临近点到起点的距离,并与原路径的距离比较。如果路径更短,则将临近点的距离更新为更短的路径,并将前驱节点设置为该最近点。
重复上述步骤,直到所有节点都被访问且路径都被计算出来。
下面是一个简单的Python程序,实现了Dijkstra最短路径算法:
```
# 定义一个函数,实现Dijkstra算法
def Dijkstra(G, s):
# 初始化起点
unvisited = set(G.nodes())
distance = dict.fromkeys(list(G.nodes()), float('inf'))
previous = dict.fromkeys(list(G.nodes()), None)
distance[s] = 0
while unvisited:
# 找到距离起点最近的节点
minVertex = None
for vertex in unvisited:
if minVertex == None:
minVertex = vertex
elif distance[vertex] < distance[minVertex]:
minVertex = vertex
# 如果找不到,则结束
if distance[minVertex] == float('inf'):
break
# 寻找与最近点相邻的节点
for neighbor in G.neighbors(minVertex):
alt = distance[minVertex] + G[minVertex][neighbor]['weight']
# 更新临近节点的距离和前驱节点
if alt < distance[neighbor]:
distance[neighbor] = alt
previous[neighbor] = minVertex
# 将最近的点标记为已访问
unvisited.remove(minVertex)
return distance, previous
```
该程序中,输入参数G代表一个带权重的图,s代表起始节点。输出结果包括distance和previous两个字典,分别表示起始节点到其他点的最短距离和前驱节点。算法的时间复杂度为O(V^2),其中V为节点数。
总之,Dijkstra最短路径算法在Python语言中的实现较为简单,基于贪心算法的思想,可以较快地解决单源最短路径问题。
### 回答3:
Dijkstra最短路径算法是一种用于解决带权重图中单源最短路径问题的算法,也是最为常见的最短路径算法之一。在Python中实现该算法,可以采用以下步骤:
1.定义数据结构:首先,需要定义数据结构,包括节点、边和权重等信息。通常使用字典来存储节点和边信息,采用列表或集合来存储待处理节点。权重可以采用二维数组或字典存储,这里推荐使用字典存储,可以更方便地进行读写操作。
2.初始化算法:初始化算法需要准备两个列表,一个是记录节点的父节点,初始为None;另一个是记录每个节点到源节点的距离,初始化为无穷大。然后将源节点加入待处理节点列表(记为unvisited)中,并将源节点到源节点的距离赋值为0。
3.迭代:在每个迭代中,从待处理节点列表中选出一个距离源节点最近的点(记为current),并将该节点从待处理节点列表中移除。接着,遍历当前节点的所有邻居(记为neighbor),更新它们到源节点的距离,如果更新后的距离更短,则更新父节点和距离。最后,将更新过的邻居节点加入待处理节点列表中。
4.返回结果:算法完成后,可以通过遍历每个节点的父节点链,从源节点到任意节点得到最短路径。
以下是Python代码示例(仅供参考):
```
def dijkstra(graph, start):
# 初始化算法
unvisited = list(graph.keys())
distance = {node: float('inf') for node in graph}
distance[start] = 0
parent = {node: None for node in graph}
current = start
# 迭代
while unvisited:
unvisited.remove(current)
for neighbor, cost in graph[current].items():
new_distance = distance[current] + cost
if new_distance < distance[neighbor]:
distance[neighbor] = new_distance
parent[neighbor] = current
if not unvisited:
break
candidates = {node: distance[node] for node in unvisited}
current = min(candidates, key=candidates.get)
# 返回结果
path = {}
for node in graph:
if node != start:
path[node] = [node]
current = node
while parent[current] != start:
path[node].append(parent[current])
current = parent[current]
path[node].append(start)
path[node].reverse()
return distance, path
```
以上就是Dijkstra最短路径算法在Python中的简要实现方法。在实际使用中,还需根据具体需求进行适当的修改和优化。
DIJKSTRA最短路径路由python代码
以下是一个简单的 Python 实现 Dijkstra 算法的代码:
```python
import heapq
def dijkstra(graph, start):
"""
计算从 start 到其他节点的最短路径
graph: 字典类型,key 为节点名称,value 为连接的节点和距离的字典
start: 起始节点名称
"""
distances = {} # 存储从 start 到各个节点的最短距离
for node in graph:
distances[node] = float('inf')
distances[start] = 0
pq = [(0, start)]
while pq:
(dist, current_node) = heapq.heappop(pq)
if dist > distances[current_node]:
continue
for neighbor, weight in graph[current_node].items():
distance = dist + weight
if distance < distances[neighbor]:
distances[neighbor] = distance
heapq.heappush(pq, (distance, neighbor))
return distances
# 测试
graph = {
'A': {'B': 2, 'C': 5},
'B': {'A': 2, 'C': 1},
'C': {'A': 5, 'B': 1, 'D': 3},
'D': {'C': 3}
}
print(dijkstra(graph, 'A')) # 输出 {'A': 0, 'B': 2, 'C': 3, 'D': 6}
```
注:以上代码中,使用 heapq 模块实现了一个优先队列,用于存储节点及其到起始节点的距离。
阅读全文
相关推荐
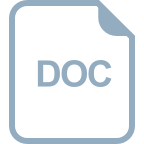
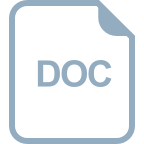
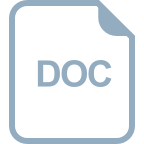
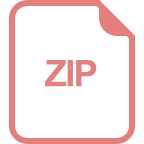
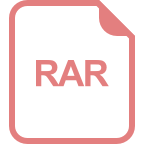






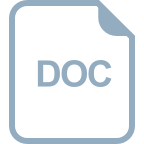
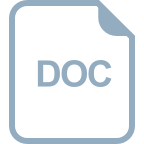
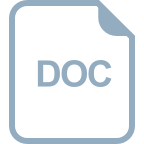


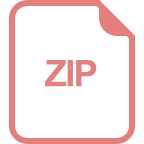
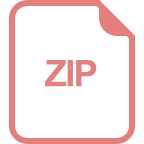