from datasets import load_dataset, load_json_data
时间: 2023-12-07 16:05:10 浏览: 25
这两个方法都不是 Python 内置方法,是需要安装相应的第三方库才能使用的。
`load_dataset` 方法来自 Hugging Face 公司的 `datasets` 库,用于加载各种常见的自然语言处理数据集,例如语言模型训练数据、文本分类数据等等。你可以使用 `pip` 命令安装该库:
```
pip install datasets
```
`load_json_data` 方法不太清楚是哪个库提供的。可能是你自己写的一个工具方法,也可能是某个外部库提供的。需要更多上下文才能确定。
相关问题
python load_dataset
As an AI language model, I don't have access to any local files or datasets. However, I can provide you with the general steps to load a dataset in python:
1. Install any necessary libraries: Depending on the type and format of your dataset, you may need to install specific libraries like pandas, numpy, or scikit-learn.
2. Import the necessary libraries: Once you've installed the necessary libraries, you'll need to import them into your python script.
3. Load the dataset: There are various ways to load a dataset into python, depending on the format of the data. For example, if you have a CSV file, you can use the pandas library to read it using the read_csv() function. If you have an Excel file, you can use the read_excel() function. If you have a JSON file, you can use the json library to load it.
4. Preprocess the data: Once you've loaded the dataset, you may need to preprocess the data to clean it up or transform it into a suitable format for analysis.
5. Analyze the data: With the dataset loaded and preprocessed, you can start analyzing the data using various statistical and machine learning techniques.
Here's an example of loading a CSV file using pandas:
```
import pandas as pd
# Load the dataset
df = pd.read_csv('my_dataset.csv')
# Preprocess the data
# ...
# Analyze the data
# ...
```
paddlenlp.datasets.dataset.DatasetBuilder模块,如何使用_get_data方法,_read方法和read方法获取本地数据,生成数据集,请代码展示
好的,以下是使用`paddlenlp.datasets.dataset.DatasetBuilder`模块中的`_get_data`方法、`_read`方法和`read`方法获取本地数据,生成数据集的代码示例:
```python
import os
import json
import random
import paddle
from paddlenlp.datasets.dataset_builder import DatasetBuilder
# 自定义数据集,继承DatasetBuilder类
class MyDataset(DatasetBuilder):
# 数据集名称
SPLITS = ['train', 'dev', 'test']
# 数据集文件名
FILENAME = 'my_dataset.json'
# 数据集存储目录
BASE_PATH = 'my_dataset'
# 重写_get_data方法,获取数据集
def _get_data(self, mode, **kwargs):
data_file = os.path.join(self.BASE_PATH, self.FILENAME)
if not os.path.exists(data_file):
raise FileNotFoundError("Dataset file {} not found.".format(data_file))
with open(data_file, 'r', encoding='utf-8') as f:
data = json.load(f)
return data
# 重写_read方法,对数据进行处理
def _read(self, data):
for sample in data:
# 对数据进行处理
yield {'text': sample['text'], 'label': sample['label']}
# 生成数据集
def generate_dataset():
# 数据处理函数
def convert_example(example):
# 对样本进行转换,例如分词、转id等
text = example['text']
label = example['label']
return {'text': text, 'label': label}
# 初始化数据集
dataset = MyDataset()
# 得到不同的数据集划分
train_ds, dev_ds, test_ds = dataset.get_datasets()
# 对数据进行转换
train_ds = train_ds.map(convert_example)
dev_ds = dev_ds.map(convert_example)
test_ds = test_ds.map(convert_example)
# 打印数据集大小
print("Train dataset size:", len(train_ds))
print("Dev dataset size:", len(dev_ds))
print("Test dataset size:", len(test_ds))
# 打印一条数据
print("Example data:", train_ds[0])
return train_ds, dev_ds, test_ds
# 测试代码
if __name__ == '__main__':
train_ds, dev_ds, test_ds = generate_dataset()
```
在此示例中,我们自定义了一个数据集`MyDataset`,继承了`paddlenlp.datasets.dataset_builder.DatasetBuilder`类,并实现了`_get_data`和`_read`两个方法。在`_get_data`方法中,我们从本地文件中读取数据集,而在`_read`方法中,我们对数据进行处理。最后,我们调用`get_datasets`方法获取数据集的不同划分,然后对数据进行转换并打印数据集大小和一条数据。
需要注意的是,这里的数据处理函数`convert_example`是根据具体的任务进行定义的,如果是文本分类任务,则通常需要进行分词、转id等操作。此处只是一个示例,需要根据实际情况进行修改。
相关推荐
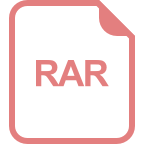
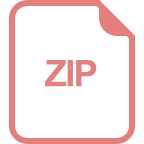












