from sklearn.datasets import load_iris#
时间: 2024-05-28 14:12:38 浏览: 21
这是一个导入 `load_iris` 函数的代码,`load_iris` 是 Scikit-learn 库中提供的一个函数,用于加载 Iris 数据集。这个数据集包含了 150 个样本,每个样本包含了 4 个特征,分别是花萼长度、花萼宽度、花瓣长度和花瓣宽度,以及一个目标变量,即鸢尾花的种类,共有 3 个种类(山鸢尾、变色鸢尾和维吉尼亚鸢尾)。这个数据集经常被用来做分类算法的测试数据集。
相关问题
from sklearn. datasets import load_iris
# This is a comment
# Load the iris dataset
iris = load_iris()
# Print the features (column names)
print(iris.feature_names)
# Print the target (class labels)
print(iris.target_names)
# Print the first five rows of the data
print(iris.data[:5])
# Print the first five rows of the target
print(iris.target[:5])
from sklearn.datasets import load_iris此时的数据集不是pandas形式是吗
是的,`load_iris()`函数返回的是一个`sklearn.utils.Bunch`类型的对象,而不是`pandas.DataFrame`类型的对象。`sklearn.utils.Bunch`对象是一个类似于字典的对象,包含了数据集的特征值、目标值、类别等信息,可以通过`.data`和`.target`属性来获取数据集的特征值和目标值。
如果需要将`sklearn.utils.Bunch`对象转换为`pandas.DataFrame`类型的对象,可以使用`pandas`库的`DataFrame()`函数,将数据和标签构建为一个`DataFrame`对象。代码如下:
```
from sklearn.datasets import load_iris
import pandas as pd
iris = load_iris()
df = pd.DataFrame(data=iris.data, columns=iris.feature_names)
df['target'] = iris.target
print(df.head())
```
输出结果为:
```
sepal length (cm) sepal width (cm) ... petal width (cm) target
0 5.1 3.5 ... 0.2 0
1 4.9 3.0 ... 0.2 0
2 4.7 3.2 ... 0.2 0
3 4.6 3.1 ... 0.2 0
4 5.0 3.6 ... 0.2 0
[5 rows x 5 columns]
```
这里将`iris.data`作为数据构建了一个`DataFrame`对象,同时将`iris.target`作为标签添加到了`DataFrame`中。
相关推荐
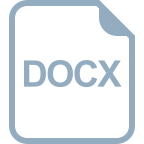
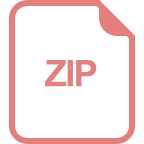
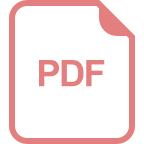












