from sklearn.datasets import load_iris from sklearn.cluster import KMeans from sklearn.metrics import fowlkes_mallows_score
时间: 2023-12-13 19:05:54 浏览: 192
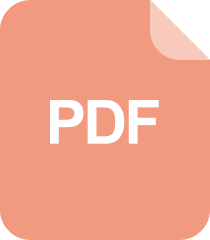
机器学习笔记2–sklearn之iris数据集
这段代码的作用是加载鸢尾花数据集,使用KMeans算法进行聚类,并计算Fowlkes-Mallows指数的得分。具体解释如下:
1. `load_iris`函数可以加载鸢尾花数据集,该数据集包含150个样本,每个样本包含4个特征(花萼长度、花萼宽度、花瓣长度、花瓣宽度),并且每个样本都属于三个类别之一(山鸢尾、变色鸢尾、维吉尼亚鸢尾)。
2. `KMeans`类是一种无监督学习算法,可以将样本分为预先指定的簇数。在这里,我们使用KMeans算法将鸢尾花数据集分为3个簇。
3. `fowlkes_mallows_score`函数可以计算Fowlkes-Mallows指数的得分,该指数用于评估聚类算法的性能。它将聚类结果与真实类别进行比较,并计算聚类结果与真实类别的相似程度。得分越高,表示聚类结果越好。
因此,这段代码的作用是使用KMeans算法对鸢尾花数据集进行聚类,并计算聚类结果的Fowlkes-Mallows指数得分。
阅读全文
相关推荐















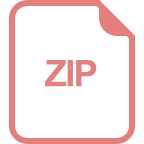