regression plots of the nn model for training, validation, testing, and all
时间: 2023-12-19 13:02:18 浏览: 29
神经网络模型的回归图显示了模型在训练、验证、测试和全部数据集上的表现。在训练数据集上,回归图展示了模型在拟合训练数据方面的表现。如果回归线与训练数据点紧密地对齐,表明模型在训练数据上表现良好。
在验证数据集上,回归图显示了模型对未见过的数据的拟合能力。如果模型在验证数据上的表现与训练数据相似,那么模型可能是泛化良好的。反之,如果模型在验证数据上的表现较差,可能意味着模型过拟合了训练数据。
在测试数据集上,回归图展示了模型对未知数据的预测能力。如果模型在测试数据上表现良好,说明模型对新数据的泛化能力较强。
最后,在所有数据集上的回归图汇总了模型在整个数据范围内的拟合情况。这可以帮助我们更全面地评估模型在各个数据集上的表现,揭示模型的整体性能。
通过分析这些回归图,可以更好地了解模型在各个数据集上的表现,并做出相应的调整和改进。因此,回归图是评估神经网络模型性能的重要工具,可以帮助我们优化模型的拟合能力和泛化能力。
相关问题
Apply the multiple linear regression model for the dataset rotifer in R
To apply the multiple linear regression model for the dataset "rotifer" in R, you can follow these steps:
1. Load the dataset into R using the `read.csv()` function.
```
rotifer <- read.csv("path/to/dataset.csv")
```
2. Create a linear regression model using the `lm()` function. In this case, we will use "abundance" as the response variable and "temperature", "phosphorus", and "phytoplankton" as the predictor variables.
```
model <- lm(abundance ~ temperature + phosphorus + phytoplankton, data = rotifer)
```
3. Check the summary of the model using the `summary()` function.
```
summary(model)
```
This will display the coefficients, standard errors, t-values, and p-values for each predictor variable. You can use this information to assess the significance and strength of the relationship between each predictor variable and the response variable.
4. Make predictions using the model using the `predict()` function. For example, to predict the abundance of rotifers at a temperature of 20°C, a phosphorus concentration of 0.5 mg/L, and a phytoplankton concentration of 5 µg/L, you can use the following code:
```
newdata <- data.frame(temperature = 20, phosphorus = 0.5, phytoplankton = 5)
prediction <- predict(model, newdata)
```
This will give you the predicted abundance of rotifers under these conditions.
Implement linear regression model and use autograd to optimize it by Pytorch.
Here is an example implementation of a linear regression model using PyTorch and Autograd for optimization:
```python
import torch
import numpy as np
# Generate some random data
np.random.seed(42)
x = np.random.rand(100, 1)
y = 2 + 3 * x + 0.1 * np.random.randn(100, 1)
# Convert data to PyTorch tensors
x_tensor = torch.from_numpy(x).float()
y_tensor = torch.from_numpy(y).float()
# Define the model
class LinearRegression(torch.nn.Module):
def __init__(self):
super(LinearRegression, self).__init__()
self.linear = torch.nn.Linear(1, 1)
def forward(self, x):
return self.linear(x)
model = LinearRegression()
# Define the loss function
criterion = torch.nn.MSELoss()
# Define the optimizer
optimizer = torch.optim.SGD(model.parameters(), lr=0.01)
# Train the model
num_epochs = 1000
for epoch in range(num_epochs):
# Forward pass
y_pred = model(x_tensor)
loss = criterion(y_pred, y_tensor)
# Backward pass and optimization
optimizer.zero_grad()
loss.backward()
optimizer.step()
# Print progress
if (epoch+1) % 100 == 0:
print('Epoch [{}/{}], Loss: {:.4f}'.format(epoch+1, num_epochs, loss.item()))
# Print the learned parameters
w, b = model.parameters()
print('w =', w.item())
print('b =', b.item())
```
In this example, we define a linear regression model as a subclass of `torch.nn.Module`, with a single linear layer. We use the mean squared error loss function and stochastic gradient descent optimizer to train the model on the randomly generated data. The model parameters are learned through backpropagation using the `backward()` method, and are optimized using the `step()` method of the optimizer. After training, we print the learned values of the slope and intercept parameters.
相关推荐
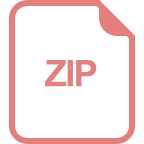
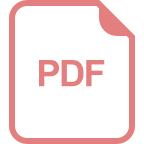
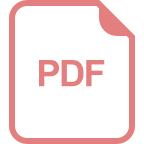













