Applying Lasso Regularization method and will try to increase accuracy of model
时间: 2024-03-11 19:51:44 浏览: 17
Lasso regularization is a method used to reduce overfitting in a linear regression model by adding a penalty term to the loss function. This penalty term is the absolute value of the coefficients multiplied by a hyperparameter alpha. By increasing the value of alpha, some of the coefficients are set to zero, leading to a simpler model that is less prone to overfitting.
To apply Lasso regularization to your model, you can use the Lasso class from the scikit-learn library. First, split your data into training and test sets, and then instantiate the Lasso class with your preferred value of alpha. Finally, fit the model on the training data and evaluate its performance on the test data.
Here is an example code:
```
from sklearn.linear_model import Lasso
from sklearn.model_selection import train_test_split
from sklearn.metrics import r2_score
# Split data into train and test sets
X_train, X_test, y_train, y_test = train_test_split(X, y, test_size=0.2, random_state=42)
# Instantiate Lasso model with alpha=0.1
lasso = Lasso(alpha=0.1)
# Fit model on training data
lasso.fit(X_train, y_train)
# Predict on test data
y_pred = lasso.predict(X_test)
# Evaluate model performance using R-squared score
r2 = r2_score(y_test, y_pred)
print("R-squared score:", r2)
```
You can try different values of alpha to find the one that gives the best performance on your data.
相关推荐
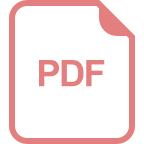
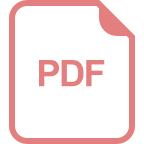
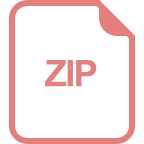














