pytorch设置随机数种子
时间: 2023-09-05 22:12:59 浏览: 52
在 PyTorch 中设置随机数种子可以提高可重复性和稳定性。可以通过以下代码设置 PyTorch 的随机数种子:
```python
import torch
# 设置随机数种子
torch.manual_seed(1234)
# 如果使用 GPU
if torch.cuda.is_available():
torch.cuda.manual_seed_all(1234)
```
在设置完随机数种子之后,每次运行代码的结果将会是相同的。注意,设置随机数种子只能保证在同一台机器上运行相同代码时的结果相同,如果在不同机器上运行或者修改了代码,结果可能会不同。
相关问题
pytorch 设置随机数种子
在使用 PyTorch 进行深度学习模型训练时,为了保证每次训练的结果一致,需要设置随机数种子。可以通过设置随机数种子,在 GPU 或 CPU 上固定每一次的训练结果。在 PyTorch 中,可以通过以下代码设置随机数种子:
1. 定义一个可以设置随机种子的函数:
```
def setup_seed(seed):
torch.manual_seed(seed)
torch.cuda.manual_seed_all(seed)
np.random.seed(seed)
random.seed(seed)
torch.backends.cudnn.deterministic = True
# 设置随机数种子
setup_seed(1234)
```
2. 在程序执行的开始处添加以下代码:
```
def seed_everything():
''' 设置整个开发环境的seed
:param seed:
:param device:
:return:
'''
import os
import random
import numpy as np
random.seed(seed)
os.environ['PYTHONHASHSEED'] = str(seed)
np.random.seed(seed)
torch.manual_seed(seed)
torch.cuda.manual_seed(seed)
torch.cuda.manual_seed_all(seed)
# some cudnn methods can be random even after fixing the seed
# unless you tell it to be deterministic
torch.backends.cudnn.deterministic = True
```
以上两种方法都可以设置随机数种子,保证每次训练的结果一致。需要注意的是,如果使用了 GPU 进行训练,需要同时设置 CPU 和 GPU 的随机数种子。
pytorch固定随机数种子
要在PyTorch中固定随机数种子,可以按照以下步骤进行操作:
首先,导入相关的库:
```python
import random
import numpy as np
import torch
```
然后,设置随机数种子:
```python
seed = 42
# 设置Python随机数种子
random.seed(seed)
# 设置NumPy随机数种子
np.random.seed(seed)
# 设置PyTorch随机数种子
torch.manual_seed(seed)
torch.cuda.manual_seed(seed)
torch.cuda.manual_seed_all(seed)
# 设置CUDNN随机数种子
torch.backends.cudnn.deterministic = True
torch.backends.cudnn.benchmark = False
```
通过以上步骤,你就可以在PyTorch中固定随机数种子。这将确保每次运行代码时生成的随机数都是相同的。
相关推荐
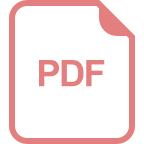
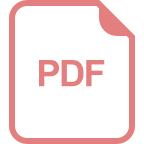
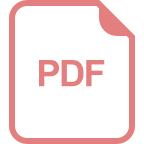












