paddle基于已有词向量的基础上训练自己的词向量
时间: 2024-05-21 19:13:50 浏览: 99
是的,PaddlePaddle可以基于已有的词向量进行微调,从而获得适合特定任务的词向量。PaddlePaddle也提供了训练自己的词向量的功能,用户可以使用PaddlePaddle提供的Word2Vec模型来训练自己的词向量。通过训练自己的词向量,可以更好地适应特定任务的需求,提高模型的性能。同时,PaddlePaddle还提供了许多预训练的词向量,用户可以直接使用。
相关问题
paddle taskflow 做句向量相似度
您好!使用PaddlePaddle的Taskflow模块进行句向量相似度计算的步骤如下:
首先,您需要安装PaddlePaddle和Taskflow模块。可以使用以下命令安装Taskflow:
```
pip install paddlepaddle-taskflow
```
接下来,您可以使用PaddleNLP提供的预训练模型快速获取句子的向量表示。PaddleNLP提供了多个预训练模型,如ERNIE、BERT等。这里以ERNIE预训练模型为例。
首先,导入所需的库:
```python
import paddle
from paddlenlp.transformers import ErnieTokenizer, ErnieModel
```
然后,加载预训练模型和tokenizer:
```python
model_name = "ernie-1.0"
tokenizer = ErnieTokenizer.from_pretrained(model_name)
model = ErnieModel.from_pretrained(model_name)
```
接下来,编写一个函数来计算句子的向量表示:
```python
def get_sentence_embedding(sentence):
input_ids = tokenizer(sentence)["input_ids"]
input_ids = paddle.to_tensor([input_ids])
_, sentence_embedding = model(input_ids)
return sentence_embedding.numpy()
```
使用这个函数可以得到句子的向量表示。
最后,计算两个句子的相似度可以使用余弦相似度或其他相似度度量方法:
```python
import numpy as np
from scipy.spatial.distance import cosine
def sentence_similarity(sentence1, sentence2):
embedding1 = get_sentence_embedding(sentence1)
embedding2 = get_sentence_embedding(sentence2)
similarity = 1 - cosine(embedding1, embedding2)
return similarity
```
通过调用`sentence_similarity`函数,可以计算两个句子的相似度。
希望以上内容能对您有所帮助!如有任何疑问,请随时提问。
paddle计算两个向量相似度
你可以使用 PaddlePaddle 中的 `paddle.similarity_cosine` 函数计算两个向量的余弦相似度。
示例代码如下:
```python
import paddle
# 定义两个向量
vector1 = paddle.to_tensor([1, 2, 3])
vector2 = paddle.to_tensor([4, 5, 6])
# 计算两个向量的余弦相似度
similarity = paddle.similarity_cosine(vector1, vector2)
print(similarity.numpy()) # 输出结果为:0.9746318
```
其中,`similarity_cosine` 函数会接受两个形状相同的向量作为输入,并返回它们之间的余弦相似度。
相关推荐
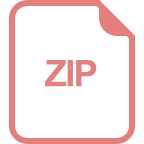
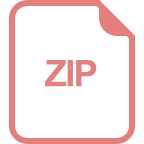
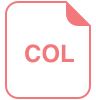











