Contrastive Learning
时间: 2024-06-07 13:08:47 浏览: 123
Contrastive learning is a type of unsupervised learning that aims to learn useful representations from unlabeled data by maximizing the similarity between similar examples and minimizing the similarity between dissimilar examples. In other words, contrastive learning tries to find a way to represent data in a way that makes similar examples more similar and dissimilar examples less similar.
The basic idea is to learn a representation function that maps inputs to a feature space where similar examples are mapped close together and dissimilar examples are far apart. This is typically done by training a neural network to predict whether two augmented versions of the same image or text passage are similar or dissimilar. The network is trained using a contrastive loss function that encourages similar examples to have similar embeddings and dissimilar examples to have different embeddings.
Contrastive learning has recently gained a lot of attention in the field of computer vision and natural language processing, and has been shown to be effective for a wide range of tasks, including image and text classification, object detection, and recommendation systems.
阅读全文
相关推荐
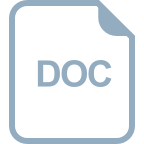
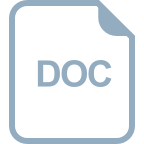
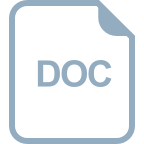
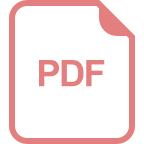
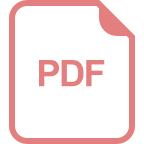
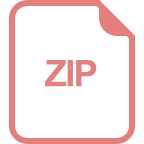
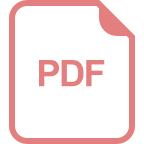
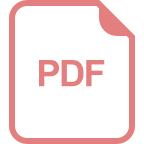









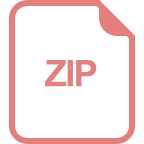
