bifpn代码pytorch版本
时间: 2024-06-20 21:03:07 浏览: 167
BiFPN(Bidirectional Feature Pyramid Network)是一种用于目标检测的网络结构,其最初由Google在2019年提出。其主要特点是可以自适应地融合不同层级的特征图,同时考虑到了信息的上下文关系。BiFPN模块通常被嵌入到目标检测网络中,以提高检测性能。
以下是BiFPN代码的PyTorch实现版本的示例:
```python
import torch
import torch.nn as nn
import torch.nn.functional as F
class BiFPNLayer(nn.Module):
def __init__(self, channels):
super(BiFPNLayer, self).__init__()
self.conv6_up = nn.Conv2d(channels, channels, kernel_size=1, stride=1, padding=0)
self.conv5_up = nn.Conv2d(channels, channels, kernel_size=1, stride=1, padding=0)
self.conv4_up = nn.Conv2d(channels, channels, kernel_size=1, stride=1, padding=0)
self.conv3_up = nn.Conv2d(channels, channels, kernel_size=1, stride=1, padding=0)
self.conv4_down = nn.Conv2d(channels, channels, kernel_size=1, stride=1, padding=0)
self.conv5_down = nn.Conv2d(channels, channels, kernel_size=1, stride=1, padding=0)
self.conv6_down = nn.Conv2d(channels, channels, kernel_size=1, stride=1, padding=0)
self.conv7_down = nn.Conv2d(channels, channels, kernel_size=1, stride=1, padding=0)
self.p6_upsample = nn.Upsample(scale_factor=2.0, mode='nearest')
self.p5_upsample = nn.Upsample(scale_factor=2.0, mode='nearest')
self.p4_upsample = nn.Upsample(scale_factor=2.0, mode='nearest')
self.p3_upsample = nn.Upsample(scale_factor=2.0, mode='nearest')
self.p4_downsample = nn.MaxPool2d(kernel_size=2, stride=2)
self.p5_downsample = nn.MaxPool2d(kernel_size=2, stride=2)
self.p6_downsample = nn.MaxPool2d(kernel_size=2, stride=2)
self.relu = nn.ReLU()
def forward(self, p3_in, p4_in, p5_in, p6_in):
# Bottom-up pathway
p6_td = self.conv6_up(p6_in)
p5_td = self.conv5_up(p5_in) + F.interpolate(p6_td, scale_factor=2.0)
p4_td = self.conv4_up(p4_in) + F.interpolate(p5_td, scale_factor=2.0)
p3_out = self.conv3_up(p3_in) + F.interpolate(p4_td, scale_factor=2.0)
# Top-down pathway
p4_out = self.conv4_down(p4_in) + F.interpolate(p3_out, scale_factor=0.5)
p5_out = self.conv5_down(p5_in) + F.interpolate(p4_out, scale_factor=0.5)
p6_out = self.conv6_down(p6_in) + F.interpolate(p5_out, scale_factor=0.5)
p7_out = self.conv7_down(p6_out)
# Fusion
p6_td = F.interpolate(p6_td, size=p7_out.shape[-2:]) + p7_out
p5_td = F.interpolate(p5_td, size=p6_td.shape[-2:]) + self.p6_upsample(p6_td)
p4_td = F.interpolate(p4_td, size=p5_td.shape[-2:]) + self.p5_upsample(p5_td)
p3_out = F.interpolate(p3_out, size=p4_td.shape[-2:]) + self.p4_upsample(p4_td)
p4_out = self.relu(self.conv4_down(p4_out) + self.p4_downsample(p3_out))
p5_out = self.relu(self.conv5_down(p5_out) + self.p5_downsample(p4_out))
p6_out = self.relu(self.conv6_down(p6_out) + self.p6_downsample(p5_out))
return p3_out, p4_out, p5_out, p6_out
```
阅读全文
相关推荐
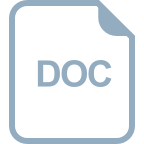
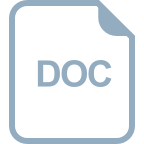
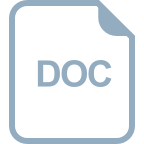



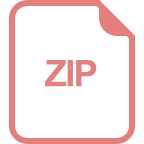
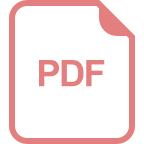
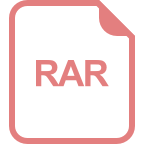
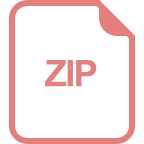
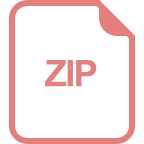
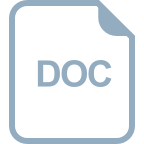
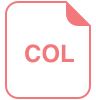



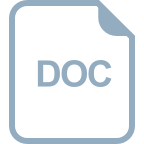
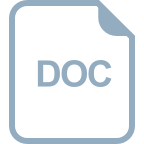
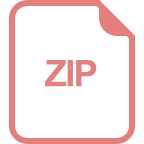