clusters, centers = kmeans(data, 4)ValueError: Unable to coerce to Series/DataFrame, dimension must be <= 2: (4, 1, 2)
时间: 2024-01-11 18:03:07 浏览: 80
这个错误通常是因为你的数据格式不正确导致的。kmeans算法需要的输入数据是二维的,但是你的数据似乎有三个维度,因此无法进行聚类。
你可以尝试使用reshape函数将数据转换为二维格式,例如:
```python
import numpy as np
data = np.random.rand(4, 1, 2)
data_reshaped = data.reshape(4, 2)
# 执行聚类算法
clusters, centers = kmeans(data_reshaped, 4)
```
这里假设你的数据是一个形状为(4, 1, 2)的三维数组,我们可以使用reshape函数将其转换为一个形状为(4, 2)的二维数组,然后再执行聚类算法。
相关问题
import numpy as np import matplotlib.pyplot as plt import pandas as pd def kmeans(data, k): # 手动指定聚类中心 centers = np.array([[21.0, 1.0], [-1.0, 20.0], [0.0, 0.0],[-1.0, -8.0]]) # 记录每个数据点所属的簇 clusters = np.zeros(len(data)) # 聚类迭代次数 max_iter = 100 for i in range(max_iter): # 计算每个数据点到聚类中心的距离 distances = np.sqrt(((data - centers[:, np.newaxis])**2).sum(axis=2)) # 将每个数据点分配到最近的聚类中心所在的簇 clusters = np.argmin(distances, axis=0) # 更新聚类中心 for j in range(k): centers[j] = data[clusters == j].mean(axis=0) return clusters, centers # 生成数据集 data = pd.read_excel('allindex2.xlsx') # 聚类 clusters, centers = kmeans(data, 4) # 绘制结果 plt.scatter(data[:, 0], data[:, 1], c=clusters) plt.scatter(centers[:, 0], centers[:, 1], marker='x', s=200, linewidths=3, color='r') plt.show()ValueError: Unable to coerce to Series/DataFrame, dimension must be <= 2: (4, 1, 2)
这个错误是因为在绘制散点图时,使用了一个二维的数据集,而数据集实际上是三维的。这是因为在 `kmeans` 函数中,我们使用了一个三维的数组来存储数据点的坐标,其中第一维是数据点的编号,第二维和第三维是数据点的坐标。
要解决这个问题,我们需要在调用 `plt.scatter` 函数时,只传入前两维的数据。具体来说,可以使用 `data[:, 0]` 和 `data[:, 1]` 来分别表示数据点的 x 坐标和 y 坐标,然后将它们传递给 `plt.scatter` 函数即可。修改后的代码如下所示:
```python
import numpy as np
import matplotlib.pyplot as plt
import pandas as pd
def kmeans(data, k):
# 手动指定聚类中心
centers = np.array([[21.0, 1.0], [-1.0, 20.0], [0.0, 0.0],[-1.0, -8.0]])
# 记录每个数据点所属的簇
clusters = np.zeros(len(data))
# 聚类迭代次数
max_iter = 100
for i in range(max_iter):
# 计算每个数据点到聚类中心的距离
distances = np.sqrt(((data - centers[:, np.newaxis])**2).sum(axis=2))
# 将每个数据点分配到最近的聚类中心所在的簇
clusters = np.argmin(distances, axis=0)
# 更新聚类中心
for j in range(k):
centers[j] = data[clusters == j].mean(axis=0)
return clusters, centers
# 生成数据集
data = pd.read_excel('allindex2.xlsx').values
# 聚类
clusters, centers = kmeans(data, 4)
# 绘制结果
plt.scatter(data[:, 0], data[:, 1], c=clusters)
plt.scatter(centers[:, 0], centers[:, 1], marker='x', s=200, linewidths=3, color='r')
plt.show()
```
这样就可以正确绘制出散点图了。
ValueError Traceback (most recent call last) Cell In[39], line 3 1 from sklearn.cluster import KMeans 2 model_kmean = KMeans(n_clusters=3) ----> 3 cluster_labels_1= model_kmean.fit_predict(df1) 4 cluster_labels1=pd.DataFrame(cluster_labels_1, columns=['clusters']) 5 merge_data1=pd.concat([a, pd.Series(cluster_labels_1, index=df1.index)], axis=1) File ~\anaconda3\lib\site-packages\sklearn\cluster\_kmeans.py:1033, in _BaseKMeans.fit_predict(self, X, y, sample_weight) 1010 def fit_predict(self, X, y=None, sample_weight=None): 1011 """Compute cluster centers and predict cluster index for each sample. 1012 1013 Convenience method; equivalent to calling fit(X) followed by (...) 1031 Index of the cluster each sample belongs to. 1032 """ -> 1033 return self.fit(X, sample_weight=sample_weight).labels_
这段代码出现了一个 ValueError,可能是由于数据类型不匹配或参数设置错误导致的。建议检查一下 df1 的数据类型和值是否符合 KMeans 模型的要求。另外,也可以尝试调整一下 n_clusters 参数的值看看是否能够解决问题。
阅读全文
相关推荐
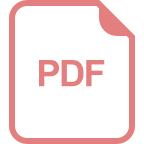
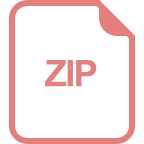
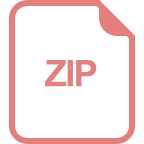
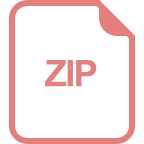
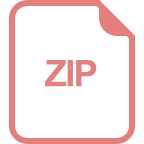
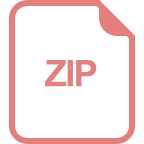
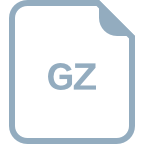
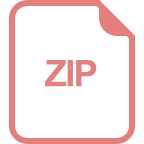
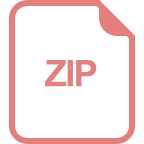
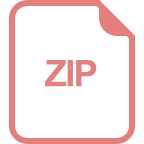
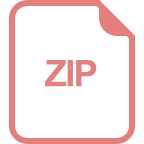
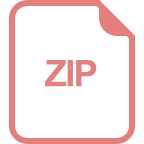
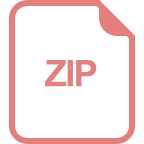
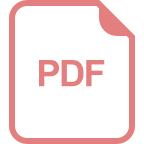
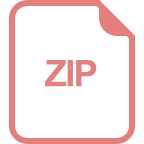

