nn.Parameter.detach()
时间: 2023-06-24 11:07:50 浏览: 47
`nn.Parameter.detach()`方法是将一个`nn.Parameter`对象从其所在的计算图中分离出来,返回一个不再连接到计算图的普通张量。这个方法常用于将模型中的某些参数冻结,使其在训练过程中不再更新。
在PyTorch中,`nn.Parameter`是继承自`torch.Tensor`的一个特殊类型,它会被自动加入到计算图中,并且可以通过反向传播来更新。但有时我们希望将某些参数固定下来,不参与梯度计算,这时就可以使用`detach()`方法将其从计算图中分离出来。
需要注意的是,`detach()`方法返回的张量与原张量共享内存,因此修改其中一个张量的值会影响到另一个张量。如果需要对返回的张量进行修改而不影响原张量,可以使用`clone()`方法创建一个新的张量。
相关问题
class AAMsoftmax(nn.Module): def __init__(self, n_class, m, s): super(AAMsoftmax, self).__init__() self.m = m self.s = s self.weight = torch.nn.Parameter(torch.FloatTensor(n_class, 256), requires_grad=True) self.ce = nn.CrossEntropyLoss() nn.init.xavier_normal_(self.weight, gain=1) self.cos_m = math.cos(self.m) self.sin_m = math.sin(self.m) self.th = math.cos(math.pi - self.m) self.mm = math.sin(math.pi - self.m) * self.m def forward(self, x, label=None): cosine = F.linear(F.normalize(x), F.normalize(self.weight)) sine = torch.sqrt((1.0 - torch.mul(cosine, cosine)).clamp(0, 1)) phi = cosine * self.cos_m - sine * self.sin_m phi = torch.where((cosine - self.th) > 0, phi, cosine - self.mm) one_hot = torch.zeros_like(cosine) one_hot.scatter_(1, label.view(-1, 1), 1) output = (one_hot * phi) + ((1.0 - one_hot) * cosine) output = output * self.s loss = self.ce(output, label) prec1 = accuracy(output.detach(), label.detach(), topk=(1,))[0]
这段代码是一个 PyTorch 的模型实现,实现了一个 AAMsoftmax 的分类器。这个分类器的输入是一个 x 矩阵,代表输入的特征,label 是一个张量,代表输入的标签。该模型的 forward 函数中,首先对输入的特征 x 进行归一化,然后通过线性变换将 x 投影到一个维度为 256 的空间中,得到一个 cosine 矩阵。接下来通过一系列的数学计算,将 cosine 转化为一个 phi 矩阵,使得在 phi 矩阵上的分类间的 margin 更大,以提高分类的精度。最后,将 phi 矩阵和原始的 cosine 矩阵加权相加,得到最终的输出。同时,计算交叉熵损失和精度,并返回。
Traceback (most recent call last): File "D:\yolov7-lpr\yolov7_plate-master\models\lprnet_plate_recognition.py", line 50, in <module> out_data = model(img_tensor) # out_data.shape == [1, 68, 18] File "D:\Anaconda\lib\site-packages\torch\nn\modules\module.py", line 1102, in _call_impl return forward_call(*input, **kwargs) File "D:\yolov7-lpr\yolov7_plate-master\models\LPRNet.py", line 62, in forward x = layer(x) File "D:\Anaconda\lib\site-packages\torch\nn\modules\module.py", line 1102, in _call_impl return forward_call(*input, **kwargs) File "D:\Anaconda\lib\site-packages\torch\nn\modules\conv.py", line 446, in forward return self._conv_forward(input, self.weight, self.bias) File "D:\Anaconda\lib\site-packages\torch\nn\modules\conv.py", line 442, in _conv_forward return F.conv2d(input, weight, bias, self.stride, TypeError: conv2d() received an invalid combination of arguments - got (Tensor, Parameter, Parameter, tuple, tuple, tuple, int), but expected one of: * (Tensor input, Tensor weight, Tensor bias, tuple of ints stride, tuple of ints padding, tuple of ints dilation, int groups) didn't match because some of the arguments have invalid types: (Tensor, !Parameter!, !Parameter!, !tuple!, !tuple!, !tuple!, int) * (Tensor input, Tensor weight, Tensor bias, tuple of ints stride, str padding, tuple of ints dilation, int groups) didn't match because some of the arguments have invalid types: (Tensor, !Parameter!, !Parameter!, !tuple!, !tuple!, !tuple!, int)
这个错误是由于在使用`F.conv2d()`函数时传入了一个`Parameter`类型的参数,但是这个函数期望传入的是一个`Tensor`类型参数。
可能的原因是在模型定义时使用了`nn.Parameter`来定义权重或偏置,而在调用模型进行推理时未将其转换为`Tensor`类型。
解决方法是将权重和偏置参数转换为`Tensor`类型,可以使用`nn.Parameter.detach()`方法将其转换为`Tensor`类型。具体来说,可以在模型定义中将`nn.Parameter`改为`nn.Tensor`,或者在模型调用时使用`nn.Parameter.detach()`方法转换为`Tensor`类型。
相关推荐
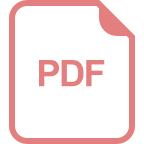
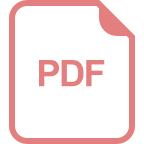
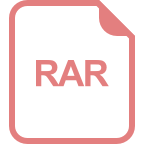













