WGAN自动生成动漫头像PyTorch 代码
时间: 2024-05-04 17:20:29 浏览: 8
以下是使用WGAN生成动漫头像的PyTorch代码,其中使用了DCGAN的结构和WGAN的损失函数。
首先需要导入必要的库:
```python
import torch
import torch.nn as nn
import torch.optim as optim
import torchvision.utils as vutils
import torchvision.datasets as dset
import torchvision.transforms as transforms
from torch.utils.data import DataLoader
from torch.autograd import Variable
import numpy as np
import matplotlib.pyplot as plt
import matplotlib.animation as animation
from IPython.display import HTML
```
接下来定义一些超参数:
```python
# Root directory for dataset
dataroot = "./data"
# Number of workers for dataloader
workers = 2
# Batch size during training
batch_size = 64
# Spatial size of training images. All images will be resized to this
# size using a transformer.
image_size = 64
# Number of channels in the training images. For color images this is 3
nc = 3
# Size of z latent vector (i.e. size of generator input)
nz = 100
# Size of feature maps in generator
ngf = 64
# Size of feature maps in discriminator
ndf = 64
# Number of training epochs
num_epochs = 5
# Learning rate for optimizers
lr = 0.00005
# Beta1 hyperparam for Adam optimizers
beta1 = 0.5
# Number of GPUs available. Use 0 for CPU mode.
ngpu = 0
# Number of critic iterations per generator iteration
n_critic = 5
# Clipping parameter for WGAN
clip_value = 0.01
# Output directory for generated images
output_dir = "./output"
```
接下来定义数据加载器:
```python
# Create the dataset
dataset = dset.ImageFolder(root=dataroot,
transform=transforms.Compose([
transforms.Resize(image_size),
transforms.CenterCrop(image_size),
transforms.ToTensor(),
transforms.Normalize((0.5, 0.5, 0.5), (0.5, 0.5, 0.5)),
]))
# Create the dataloader
dataloader = torch.utils.data.DataLoader(dataset, batch_size=batch_size,
shuffle=True, num_workers=workers)
```
接下来定义生成器和判别器的结构:
```python
# Generator Code
class Generator(nn.Module):
def __init__(self, ngpu):
super(Generator, self).__init__()
self.ngpu = ngpu
self.main = nn.Sequential(
# input is Z, going into a convolution
nn.ConvTranspose2d(nz, ngf * 8, 4, 1, 0, bias=False),
nn.BatchNorm2d(ngf * 8),
nn.ReLU(True),
# state size. (ngf*8) x 4 x 4
nn.ConvTranspose2d(ngf * 8, ngf * 4, 4, 2, 1, bias=False),
nn.BatchNorm2d(ngf * 4),
nn.ReLU(True),
# state size. (ngf*4) x 8 x 8
nn.ConvTranspose2d(ngf * 4, ngf * 2, 4, 2, 1, bias=False),
nn.BatchNorm2d(ngf * 2),
nn.ReLU(True),
# state size. (ngf*2) x 16 x 16
nn.ConvTranspose2d(ngf * 2, ngf, 4, 2, 1, bias=False),
nn.BatchNorm2d(ngf),
nn.ReLU(True),
# state size. (ngf) x 32 x 32
nn.ConvTranspose2d(ngf, nc, 4, 2, 1, bias=False),
nn.Tanh()
# state size. (nc) x 64 x 64
)
def forward(self, input):
return self.main(input)
# Discriminator Code
class Discriminator(nn.Module):
def __init__(self, ngpu):
super(Discriminator, self).__init__()
self.ngpu = ngpu
self.main = nn.Sequential(
# input is (nc) x 64 x 64
nn.Conv2d(nc, ndf, 4, 2, 1, bias=False),
nn.LeakyReLU(0.2, inplace=True),
# state size. (ndf) x 32 x 32
nn.Conv2d(ndf, ndf * 2, 4, 2, 1, bias=False),
nn.BatchNorm2d(ndf * 2),
nn.LeakyReLU(0.2, inplace=True),
# state size. (ndf*2) x 16 x 16
nn.Conv2d(ndf * 2, ndf * 4, 4, 2, 1, bias=False),
nn.BatchNorm2d(ndf * 4),
nn.LeakyReLU(0.2, inplace=True),
# state size. (ndf*4) x 8 x 8
nn.Conv2d(ndf * 4, ndf * 8, 4, 2, 1, bias=False),
nn.BatchNorm2d(ndf * 8),
nn.LeakyReLU(0.2, inplace=True),
# state size. (ndf*8) x 4 x 4
nn.Conv2d(ndf * 8, 1, 4, 1, 0, bias=False),
)
def forward(self, input):
return self.main(input).view(-1, 1).squeeze(1)
```
接下来定义初始化生成器和判别器:
```python
# Initialize generator and discriminator
netG = Generator(ngpu).cuda()
netD = Discriminator(ngpu).cuda()
```
接下来定义优化器和损失函数:
```python
# Initialize optimizer
optimizerD = optim.RMSprop(netD.parameters(), lr=lr)
optimizerG = optim.RMSprop(netG.parameters(), lr=lr)
# Initialize loss functions
criterion = nn.BCEWithLogitsLoss()
```
接下来定义训练过程:
```python
# Training Loop
# Lists to keep track of progress
img_list = []
G_losses = []
D_losses = []
iters = 0
print("Starting Training Loop...")
# For each epoch
for epoch in range(num_epochs):
# For each batch in the dataloader
for i, data in enumerate(dataloader, 0):
############################
# (1) Update D network
###########################
for n in range(n_critic):
# Initialize gradients
netD.zero_grad()
# Format batch
real_cpu = data[0].cuda()
b_size = real_cpu.size(0)
label = torch.full((b_size,), 1, device=torch.device('cuda'))
# Forward pass real batch through D
output = netD(real_cpu).view(-1)
# Calculate loss on real batch
D_loss_real = -output.mean()
# Calculate gradients for D in backward pass
D_loss_real.backward()
# Sample noise as input for G
noise = torch.randn(b_size, nz, 1, 1, device=torch.device('cuda'))
# Generate fake image batch with G
fake = netG(noise)
# Classify fake batch with D
output = netD(fake.detach()).view(-1)
# Calculate loss on fake batch
D_loss_fake = output.mean()
# Calculate gradients for D in backward pass
D_loss_fake.backward()
# Compute gradient penalty
alpha = torch.rand(b_size, 1, 1, 1).cuda()
x_hat = (alpha * real_cpu.data + (1 - alpha) * fake.data).requires_grad_(True)
out = netD(x_hat).view(-1)
grad = torch.autograd.grad(outputs=out, inputs=x_hat,
grad_outputs=torch.ones(out.size()).cuda(),
create_graph=True, retain_graph=True, only_inputs=True)[0]
gp = ((grad.norm(2, dim=1) - 1) ** 2).mean() * 10
gp.backward()
# Add the gradients from the all critic iterations
D_loss = D_loss_real + D_loss_fake + gp
Wasserstein_D = D_loss_real - D_loss_fake
# Update D
optimizerD.step()
# Clip weights of D
for p in netD.parameters():
p.data.clamp_(-clip_value, clip_value)
############################
# (2) Update G network
###########################
netG.zero_grad()
# Generate a batch of images
noise = torch.randn(b_size, nz, 1, 1, device=torch.device('cuda'))
fake = netG(noise)
# Classify the generated batch with D
output = netD(fake).view(-1)
# Calculate G's loss based on this output
G_loss = -output.mean()
# Update G
G_loss.backward()
optimizerG.step()
# Output training stats
if i % 50 == 0:
print('[%d/%d][%d/%d]\tLoss_D: %.4f\tLoss_G: %.4f\tWasserstein_D: %.4f'
% (epoch, num_epochs, i, len(dataloader),
D_loss.item(), G_loss.item(), Wasserstein_D.item()))
# Save Losses for plotting later
G_losses.append(G_loss.item())
D_losses.append(D_loss.item())
# Check how the generator is doing by saving G's output on fixed noise
if (iters % 500 == 0) or ((epoch == num_epochs-1) and (i == len(dataloader)-1)):
with torch.no_grad():
fake = netG(fixed_noise).detach().cpu()
img_list.append(vutils.make_grid(fake, padding=2, normalize=True))
iters += 1
```
接下来定义输出结果:
```python
# Output generated images
fig = plt.figure(figsize=(8, 8))
plt.axis("off")
ims = [[plt.imshow(np.transpose(i, (1, 2, 0)), animated=True)] for i in img_list]
ani = animation.ArtistAnimation(fig, ims, interval=1000, repeat_delay=1000, blit=True)
HTML(ani.to_jshtml())
# Save generated images as GIF file
fig = plt.figure(figsize=(8, 8))
plt.axis("off")
ims = [[plt.imshow(np.transpose(i, (1, 2, 0)), animated=True)] for i in img_list]
ani = animation.ArtistAnimation(fig, ims, interval=1000, repeat_delay=1000, blit=True)
ani.save(output_dir + "/anime.gif", writer='pillow', fps=2)
```
相关推荐
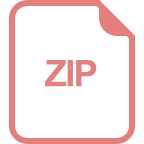
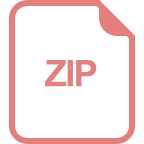
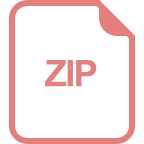














