图像分类label embedding
时间: 2023-10-26 22:36:14 浏览: 193
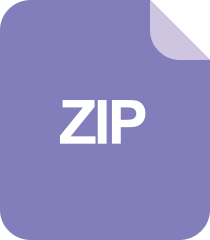
matlab图像相嵌代码-Adaptive-Graph-Guided-Embedding-for-Multi-label-Annotation
图像分类中的标签嵌入是将标签映射到高维向量空间中的过程。这种映射可以通过训练一个神经网络来实现,使得网络的输出是标签的嵌入向量。嵌入向量的维度通常比标签数量要小得多,因此可以在计算上更有效地表示标签。
一种常见的方法是使用预训练的卷积神经网络(CNN)来提取图像特征,然后将这些特征作为输入,训练一个全连接神经网络来生成标签嵌入。这个全连接网络可以使用诸如交叉熵损失函数等标准的监督学习方法进行训练。
另一种方法是使用无监督学习技术,如自编码器、变分自编码器或生成对抗网络(GAN)来学习标签嵌入。这些方法不需要标注数据,因此可以在没有标注数据的情况下学习嵌入向量。
标签嵌入可以用于许多应用程序,如图像检索、图像分类、图像聚类等。
阅读全文
相关推荐
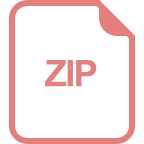
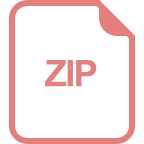
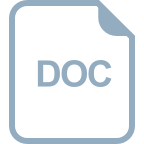
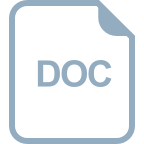
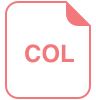
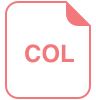
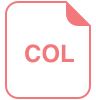
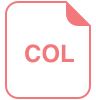
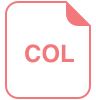
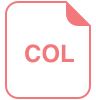
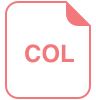
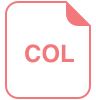





