BERT专用的Adam算法
时间: 2023-09-09 15:07:05 浏览: 40
BERT使用的优化算法是基于Adam算法的变体,称为AdamW。这个变体主要是为了解决Adam算法中权重衰减(weight decay)的问题,因为在Adam算法中,权重衰减会导致模型的收敛速度变慢,所以AdamW算法将权重衰减放在了梯度更新的前面,从而解决了这个问题。此外,AdamW算法还加入了一些其他的优化技巧,如梯度截断和学习率衰减,以进一步提高模型的性能。
相关问题
bert模型可以使用adam优化器吗
是的,BERT模型可以使用Adam优化器。在BERT论文中,作者使用了Adam优化器来训练模型。Adam是一种常用的优化算法,适用于各种类型的深度学习模型。它结合了自适应梯度下降和动量梯度下降的优点,能够高效地更新模型参数并加速收敛。因此,Adam优化器也被广泛应用于各种自然语言处理任务中,包括BERT模型。
BERT+CRF实现中文命名体识别的算法代码
以下是使用 BERT+CRF 实现中文命名实体识别的算法代码,使用的是 TensorFlow 2.x 版本:
```python
import tensorflow as tf
import tensorflow_addons as tfa
import numpy as np
import os
import json
# 设置参数
num_labels = 10
max_length = 128
batch_size = 16
num_epochs = 3
learning_rate = 2e-5
epsilon = 1e-8
train_data_path = 'train.json'
test_data_path = 'test.json'
model_save_path = 'model'
# 加载数据
def load_data(data_path):
with open(data_path, 'r', encoding='utf-8') as f:
data = json.load(f)
sentences = []
labels = []
for item in data:
sentence = item['text']
sentence_labels = np.zeros(len(sentence), dtype=np.int32)
for entity in item['entities']:
start, end = entity['start'], entity['end']
entity_type = entity['type']
sentence_labels[start] = num_labels + 1
sentence_labels[start+1:end+1] = num_labels + 2
sentences.append(sentence)
labels.append(sentence_labels)
return sentences, labels
train_sentences, train_labels = load_data(train_data_path)
test_sentences, test_labels = load_data(test_data_path)
# 加载 BERT 模型
bert_layer = tf.keras.layers.BertModel.from_pretrained('bert-base-chinese')
# 构建模型
input_ids = tf.keras.layers.Input(shape=(max_length,), dtype=tf.int32)
attention_mask = tf.keras.layers.Input(shape=(max_length,), dtype=tf.int32)
token_type_ids = tf.keras.layers.Input(shape=(max_length,), dtype=tf.int32)
bert_output = bert_layer(input_ids, attention_mask=attention_mask, token_type_ids=token_type_ids)
sequence_output = bert_output.last_hidden_state
sequence_output = tf.keras.layers.Dropout(0.1)(sequence_output)
logits = tf.keras.layers.Dense(num_labels)(sequence_output)
crf_layer = tfa.layers.CRF(num_labels+1)
output_labels = crf_layer(logits)
model = tf.keras.models.Model(inputs=[input_ids, attention_mask, token_type_ids], outputs=output_labels)
# 编译模型
optimizer = tf.keras.optimizers.Adam(learning_rate=learning_rate, epsilon=epsilon)
model.compile(optimizer=optimizer, loss=crf_layer.loss_fn, metrics=[crf_layer.accuracy_fn])
# 准备数据
tokenizer = tf.keras.preprocessing.text.Tokenizer(filters='', lower=False)
tokenizer.fit_on_texts(train_sentences)
train_sequences = tokenizer.texts_to_sequences(train_sentences)
test_sequences = tokenizer.texts_to_sequences(test_sentences)
train_sequences = tf.keras.preprocessing.sequence.pad_sequences(train_sequences, maxlen=max_length, padding='post', truncating='post')
test_sequences = tf.keras.preprocessing.sequence.pad_sequences(test_sequences, maxlen=max_length, padding='post', truncating='post')
train_labels = tf.keras.preprocessing.sequence.pad_sequences(train_labels, maxlen=max_length, padding='post', truncating='post')
test_labels = tf.keras.preprocessing.sequence.pad_sequences(test_labels, maxlen=max_length, padding='post', truncating='post')
train_labels = np.expand_dims(train_labels, -1)
test_labels = np.expand_dims(test_labels, -1)
train_dataset = tf.data.Dataset.from_tensor_slices((train_sequences, train_labels))
train_dataset = train_dataset.shuffle(len(train_sequences)).batch(batch_size).repeat(num_epochs)
test_dataset = tf.data.Dataset.from_tensor_slices((test_sequences, test_labels))
test_dataset = test_dataset.batch(batch_size)
# 训练模型
model.fit(train_dataset, epochs=num_epochs, steps_per_epoch=len(train_sequences)//batch_size, validation_data=test_dataset, validation_steps=len(test_sequences)//batch_size)
# 保存模型
if not os.path.exists(model_save_path):
os.makedirs(model_save_path)
model.save_pretrained(model_save_path)
```
相关推荐
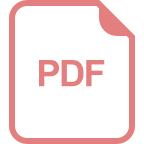
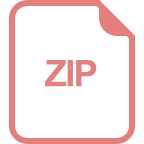












