def find_center(img): h, w = img.shape roi_h = int(h * 2 / 3) roi_img = img[roi_h:, :] img_blur = cv2.GaussianBlur(roi_img, (15, 15), 0) # 高斯模糊 ret, th2 = cv2.threshold(img_blur, 0, 255, cv2.THRESH_BINARY + cv2.THRESH_OTSU) g2 = cv2.getStructuringElement(cv2.MORPH_RECT, (3, 3)) open_img = cv2.morphologyEx(th2, cv2.MORPH_OPEN, g2, iterations=3) x_sum = np.sum(open_img, axis=0) x_point = np.where(x_sum > 0) point_x = int((x_point[0][0] + x_point[0][-1]) / 2) # print(roi_h, w) # np.savetxt('reshape_data.txt', x_point, delimiter=' ', fmt='%i') return point_x c++ opencv如何实现
时间: 2023-12-10 21:03:47 浏览: 74
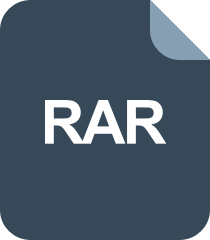
eeprom.rar_.h_eeprom_pcf8584_sm_单片机 EEPROM
以下是 C++ OpenCV 实现 find_center 函数的代码:
```c++
#include <iostream>
#include <opencv2/opencv.hpp>
using namespace std;
using namespace cv;
int find_center(Mat img) {
int h = img.rows;
int w = img.cols;
int roi_h = h * 2 / 3;
Mat roi_img = img(Rect(0, roi_h, w, h - roi_h));
Mat img_blur;
GaussianBlur(roi_img, img_blur, Size(15, 15), 0); // 高斯模糊
Mat th2;
threshold(img_blur, th2, 0, 255, THRESH_BINARY + THRESH_OTSU);
Mat g2 = getStructuringElement(MORPH_RECT, Size(3, 3));
Mat open_img;
morphologyEx(th2, open_img, MORPH_OPEN, g2, Point(-1, -1), 3);
Mat x_sum = Mat::zeros(1, w, CV_32F);
reduce(open_img, x_sum, 0, REDUCE_SUM, CV_32F);
int nonzero_count = countNonZero(x_sum);
if (nonzero_count == 0) {
return -1;
}
int nonzero_start = 0;
int nonzero_end = w - 1;
for (int i = 0; i < w; i++) {
if (x_sum.at<float>(0, i) > 0) {
nonzero_start = i;
break;
}
}
for (int i = w - 1; i >= 0; i--) {
if (x_sum.at<float>(0, i) > 0) {
nonzero_end = i;
break;
}
}
int point_x = (nonzero_start + nonzero_end) / 2;
return point_x;
}
int main() {
Mat img = imread("test.jpg", IMREAD_GRAYSCALE);
int center = find_center(img);
cout << "Center point x-coordinate: " << center << endl;
return 0;
}
```
需要注意的是,在 C++ 中,OpenCV 中的函数名和参数可能会略有不同,需要根据情况进行调整。
阅读全文
相关推荐
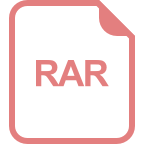
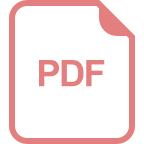



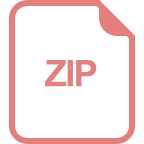
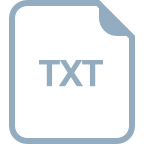
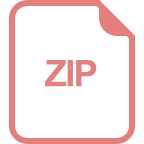
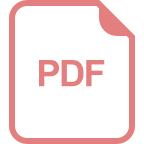
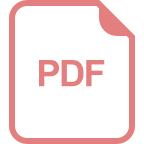
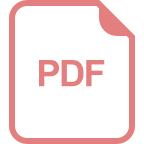
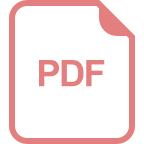
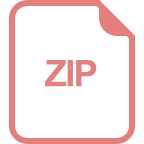
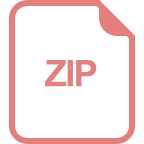
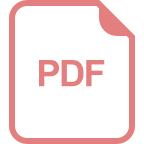
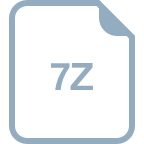