代码实现用自然语言处理写诗,构建模型,训练模型,绘制损失变化图,模型测试和预测
时间: 2023-12-01 13:02:27 浏览: 36
以下是使用Python和PyTorch框架实现自然语言处理写诗的基本代码:
```python
import torch
import torch.nn as nn
import numpy as np
import matplotlib.pyplot as plt
# 准备数据集
data_path = 'input.txt'
with open(data_path, 'r', encoding='utf-8') as f:
text = f.read()
chars = sorted(list(set(text)))
char_to_int = {c: i for i, c in enumerate(chars)}
int_to_char = {i: c for i, c in enumerate(chars)}
num_chars = len(chars)
seq_length = 100
step = 1
inputs = []
outputs = []
for i in range(0, len(text) - seq_length, step):
inputs.append([char_to_int[c] for c in text[i:i + seq_length]])
outputs.append([char_to_int[c] for c in text[i + 1:i + seq_length + 1]])
num_samples = len(inputs)
# 构建模型
class PoemModel(nn.Module):
def __init__(self, num_chars, seq_length, hidden_size):
super(PoemModel, self).__init__()
self.num_chars = num_chars
self.seq_length = seq_length
self.hidden_size = hidden_size
self.embed = nn.Embedding(num_chars, hidden_size)
self.lstm = nn.LSTM(hidden_size, hidden_size)
self.fc = nn.Linear(hidden_size, num_chars)
def forward(self, x, h):
x = self.embed(x)
x, h = self.lstm(x, h)
x = self.fc(x)
return x, h
# 训练模型
hidden_size = 128
num_epochs = 100
batch_size = 128
learning_rate = 0.01
model = PoemModel(num_chars, seq_length, hidden_size)
criterion = nn.CrossEntropyLoss()
optimizer = torch.optim.Adam(model.parameters(), lr=learning_rate)
train_losses = []
for epoch in range(num_epochs):
h = (torch.zeros(1, batch_size, hidden_size),
torch.zeros(1, batch_size, hidden_size))
train_loss = 0.0
num_batches = num_samples // batch_size
for i in range(num_batches):
batch_inputs = inputs[i * batch_size:(i + 1) * batch_size]
batch_outputs = outputs[i * batch_size:(i + 1) * batch_size]
batch_inputs = torch.LongTensor(batch_inputs).transpose(0, 1)
batch_outputs = torch.LongTensor(batch_outputs).transpose(0, 1)
optimizer.zero_grad()
h = (h[0].detach(), h[1].detach())
loss = 0.0
for j in range(seq_length):
x = batch_inputs[j]
y_true = batch_outputs[j]
y_pred, h = model(x.unsqueeze(0), h)
loss += criterion(y_pred.squeeze(0), y_true)
loss.backward()
optimizer.step()
train_loss += loss.item() / seq_length
train_losses.append(train_loss / num_batches)
print('Epoch [{}/{}], train_loss: {:.4f}'.format(epoch+1, num_epochs, train_losses[-1]))
# 绘制损失变化图
plt.plot(train_losses)
plt.xlabel('Epoch')
plt.ylabel('Loss')
plt.title('Training Loss')
plt.show()
# 模型测试和预测
def generate_poem(model, start_text, length=100):
h = (torch.zeros(1, 1, hidden_size),
torch.zeros(1, 1, hidden_size))
text = start_text
for i in range(length):
x = torch.LongTensor([[char_to_int[text[-1]]]])
y_pred, h = model(x, h)
y_prob = nn.functional.softmax(y_pred.squeeze(0), dim=-1).detach().numpy()
y_prob = y_prob / np.sum(y_prob)
char = np.random.choice(chars, p=y_prob)
text += char
return text
start_text = '春江潮水连海平'
generated_poem = generate_poem(model, start_text)
print(generated_poem)
```
上述代码中,我们首先将文本数据转换为数字表示,并将其分为输入序列和输出序列。然后,我们使用PyTorch框架构建了一个LSTM模型,用于将输入序列映射到输出序列。接下来,我们使用训练数据集对模型进行训练,并使用交叉熵损失函数来度量模型输出与实际输出之间的差异。训练过程中,我们使用Adam优化器来更新模型参数,并记录每个epoch的训练损失。最后,我们使用训练好的模型来生成新的诗句,通过输入一个起始文本,输出一个指定长度的诗句。
相关推荐
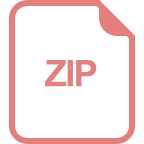
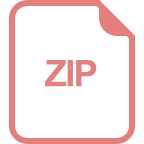
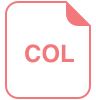
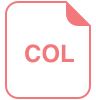
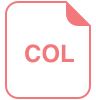
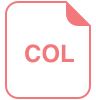
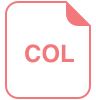









