contours = sorted(contours, key=cv2.contourArea, reverse=True)[:2] cv2.drawContours(image, contours, -1, (0, 0, 255), 3) cv2.imshow('Result', image)
时间: 2023-06-27 09:08:09 浏览: 48
这段代码的作用是在图像中找到最大的两个轮廓并进行绘制。首先使用 `cv2.findContours()` 函数找到所有轮廓,然后使用 `sorted()` 函数按照轮廓面积从大到小对轮廓进行排序,取前两个作为最大的两个轮廓。接着使用 `cv2.drawContours()` 函数将这两个轮廓绘制到原图像上,并显示结果。其中 `(0, 0, 255)` 是绘制轮廓的颜色,`3` 是轮廓线宽度。
相关问题
contours = sorted(contours, key=cv2.contourArea, reverse=True)[:10]中的sorted是什么意思
sorted是Python内置的函数,用于对可迭代对象进行排序。在这段代码中,sorted用于对轮廓列表进行排序,其中key参数指定了排序的关键字,即按照cv2.contourArea计算的轮廓面积进行排序,reverse参数指定了排序的顺序,即按照轮廓面积从大到小排序。最后,[:10]表示选取排序后的前10个轮廓。
我运行车牌识别的代码中contours = sorted(contours, key=cv2.contourArea, reverse=True)[:10]这一行出了TypeError: Required argument 'contour' (pos 1) not found这样的问题,该怎么解决
这个错误提示说明该行代码中的 `contours` 参数没有被正确传递或者没有被定义。你可以检查一下代码中是否已经定义了 `contours` 变量,或者在调用 `sorted()` 函数时是否正确传递了 `contours` 参数。
另外,你也可以尝试使用 `cv2.findContours()` 函数来获取轮廓,例如:
```
image = cv2.imread('image.jpg')
gray = cv2.cvtColor(image, cv2.COLOR_BGR2GRAY)
ret, thresh = cv2.threshold(gray, 127, 255, cv2.THRESH_BINARY)
contours, hierarchy = cv2.findContours(thresh, cv2.RETR_EXTERNAL, cv2.CHAIN_APPROX_SIMPLE)
contours = sorted(contours, key=cv2.contourArea, reverse=True)[:10]
```
这样做可以确保 `contours` 变量被正确定义并传递到 `sorted()` 函数中。
相关推荐
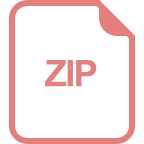
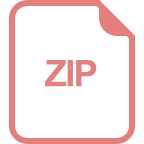













