torch.cumsum
时间: 2023-10-14 12:17:33 浏览: 43
`torch.cumsum()` is a PyTorch function that computes the cumulative sum of elements along a specified dimension of a tensor. It takes the following syntax:
```
torch.cumsum(input, dim=None, dtype=None)
```
where:
- `input`: input tensor to compute cumulative sum
- `dim`: dimension along which to compute cumulative sum. If not specified, the flattened tensor is used.
- `dtype`: optional data type of the output tensor.
The function returns a tensor containing the cumulative sum of elements along the specified dimension of the input tensor. The output tensor has the same shape as the input tensor. The first element of the output tensor is the same as the first element of the input tensor, and each subsequent element is the sum of the current element and all previous elements along the specified dimension.
For example, consider the following input tensor:
```
x = torch.tensor([[1, 2, 3], [4, 5, 6]])
```
If we apply `torch.cumsum(x, dim=1)`, we get the following output tensor:
```
tensor([[ 1, 3, 6],
[ 4, 9, 15]])
```
This is because the first row of the output tensor contains the cumulative sum of the elements in the first row of the input tensor, and the second row of the output tensor contains the cumulative sum of the elements in the second row of the input tensor.
相关推荐
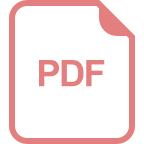
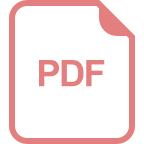













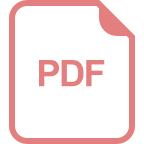
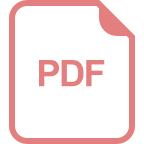