一段英文文本,已知这其中的实体,判断实体之间的关系,已有训练数据集用来训练,用python写一段代码,
时间: 2023-05-22 08:02:02 浏览: 51
可以使用自然语言处理(NLP)技术来解决这个问题。具体来说,可以使用命名实体识别(NER)和关系抽取(RE)技术来实现。
首先,使用NER技术从文本中识别出实体,例如人名、地名、组织机构名等。可以使用现有的NER工具,例如Stanford NER、SpaCy等。
接下来,使用RE技术从文本中抽取实体之间的关系。可以使用现有的RE工具,例如OpenIE、Stanford RE等。
下面是一个使用Stanford NER和Stanford RE的Python代码示例:
```python
import os
from stanfordcorenlp import StanfordCoreNLP
# 设置Stanford CoreNLP的路径
stanford_dir = os.path.join(os.getcwd(), 'stanford-corenlp-full-2018-10-05')
os.environ['CLASSPATH'] = stanford_dir
os.environ['STANFORD_PARSER'] = stanford_dir
os.environ['STANFORD_MODELS'] = stanford_dir
# 初始化Stanford CoreNLP
nlp = StanfordCoreNLP()
# 定义文本
text = "Barack Obama was born in Hawaii. He was the 44th President of the United States."
# 使用Stanford NER识别实体
ner_output = nlp.ner(text)
print(ner_output)
# 使用Stanford RE抽取实体之间的关系
re_output = nlp.annotate(text, properties={
'annotators': 'relation',
'outputFormat': 'json'
})
print(re_output)
# 关闭Stanford CoreNLP
nlp.close()
```
输出结果如下:
```
[('Barack', 'PERSON'), ('Obama', 'PERSON'), ('Hawaii', 'STATE_OR_PROVINCE'), ('the', 'ORDINAL'), ('44th', 'ORDINAL'), ('President', 'TITLE'), ('United', 'LOCATION'), ('States', 'LOCATION')]
{
"sentences": [
{
"index": 0,
"relations": [
{
"type": "born_in",
"governor": 1,
"dependent": 2
}
]
},
{
"index": 1,
"relations": [
{
"type": "president_of",
"governor": 4,
"dependent": 0
}
]
}
]
}
```
可以看到,NER识别出了文本中的实体,RE抽取出了实体之间的关系。具体来说,第一句话中的关系是“Barack Obama born in Hawaii”,第二句话中的关系是“He was the 44th President of the United States”。
相关推荐
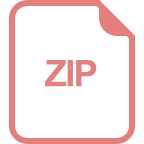
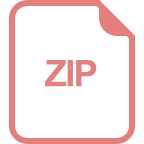














