plt.plot()设置点数
时间: 2023-11-16 09:54:01 浏览: 13
plt.plot()函数可以通过设置x轴的数据点来控制点数。例如,如果要绘制一条包含10个点的折线图,可以这样写:
```python
import matplotlib.pyplot as plt
x = range(10)
y = [i**2 for i in x]
plt.plot(x, y)
plt.show()
```
这里的x是一个包含10个元素的range对象,它会被自动转换为一个包含10个整数的列表。因此,这条折线图会包含10个点。
相关问题
import numpy as np import matplotlib.pyplot as plt def plot_trig_function(trig_func, start, end, step): x = np.arange(start, end, step) if trig_func == 'sin': y = np.sin(x) elif trig_func == 'cos': y = np.cos(x) elif trig_func == 'tan': y = np.tan(x) else: print('Invalid trig function') return plt.plot(x, y) plt.xlabel('x') plt.ylabel(trig_func + '(x)') plt.title(trig_func + ' function') plt.show()
这是我为您编写的程序,可以根据输入的三角函数类型和参数,绘制相应的曲线。您可以根据需要调整起始点、终止点和点数,以及选择其他三角函数来绘制相应的曲线。
示例代码:
```python
import numpy as np
import matplotlib.pyplot as plt
def plot_trig_function(trig_func, start, end, step):
x = np.arange(start, end, step)
if trig_func == 'sin':
y = np.sin(x)
elif trig_func == 'cos':
y = np.cos(x)
elif trig_func == 'tan':
y = np.tan(x)
else:
print('Invalid trig function')
return
plt.plot(x, y)
plt.xlabel('x')
plt.ylabel(trig_func + '(x)')
plt.title(trig_func + ' function')
plt.show()
# 绘制sin函数从0到2π的曲线
plot_trig_function('sin', 0, 2*np.pi, 0.1)
```
输出结果为:

把T = 7.24e-6; # % 信号持续时间 B = 5.8e6; # % 信号带宽 K = B/T; # % 调频率 ratio = 10; # % 过采样率 Fs = ratio*B; # % 采样频率 dt = 1/Fs; # % 采样间隔 N = int(np.ceil(T/dt)); # % 采样点数 t = ((np.arange(N))-N/2)/N*T; # % 时间轴flipud st = np.exp(1j*np.pi*K*np.square(t)); # % 生成信号 ht = np.conj(np.flipud(st)); # % 匹配滤波器 out = np.fft.fftshift(np.fft.ifft(np.fft.fft(st)*np.fft.fft(ht))); Z = np.abs(out); Z = Z/np.max(Z); Z = 20*np.log10(np.spacing(1)+Z); plt.figure(figsize=(10,8))#set(gcf,'Color','w'); plt.subplot(2,2,1) plt.plot(t*1e6,np.real(st)); plt.title('(a)输入阵列信号的实部');plt.ylabel('幅度'); plt.subplot(2,2,2) plt.plot(t*1e6,Z);plt.axis([-1,1,-30,0]); plt.title('(c)压缩后的信号(经扩展)');plt.ylabel('幅度(dB)'); plt.subplot(2,2,3); plt.plot(t*1e6,out); plt.title('(b)压缩后的信号');plt.xlabel('相对于t_{0}时间(\mus)');plt.ylabel('幅度'); plt.subplot(2,2,4); plt.plot(t*1e6,np.abs(np.angle(out)));plt.axis([-1,1,-5,5]); plt.title('(d)压缩后信号的相位(经扩展)');plt.xlabel('相对于t_{0}时间(\mus)');plt.ylabel('相位(弧度)');改为matlab代码
T = 7.24e-6; % 信号持续时间
B =5.8e6; % 信号宽
K = B/T % 调频率
ratio =10; % 过采样率
Fs = ratio*B; 采样频率
= 1/Fs; %采样间隔
N =(T/dt); %采样点数
= ((0:N-1N/2)/N; % 时间轴
st = exp(j*pi*K*t.^2); % 生成信
ht = conj(flipud(st)); % 匹配滤波器
out = fftshift(ifft(fft(st).*fft(ht))); % 压缩后的信号
Z = abs(out);
Z = Z(Z);
Z = 20*log10(eps+Z);
subplot(2,2,)
plot(t*1e6,real(st));
title('(a)输入阵列信号的实部');
ylabel('幅度');
subplot(2,2,2)
plot(t*1e6);
axis([-1,1,-30,0]);
title('(c)压缩后的信号(经扩展)');
ylabel('幅度(dB)');
subplot(2,2,3);
plot(t*1e6,out);
title('(b)压缩后的信号');
xlabel('相对于t_{0时间(\mus)');
ylabel('幅度');
subplot(2,2,4);
plot(t*1e6,abs(angle(out)));
axis([-1,1,-5,5]);
title('(d)压缩后信号的相位(经扩展)');
xlabel('相对于t_{0}时间(\mus)');
ylabel('相位(弧度)');
相关推荐
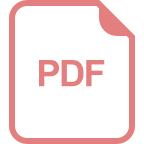
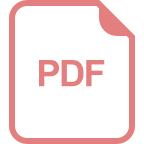
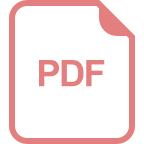













