import os import json import csv import cv2 from segment_anything import SamPredictor, sam_model_registry folder_path = 'D:\\segment-anything-main\\segment-anything-main\\input\\Normal\\' # 替换为实际的文件夹路径 output_file = 'D:\\细胞识别\\output.csv' # 替换为实际的输出文件路径 data_list = [] # 用于存储所有的坐标信息 for filename in os.listdir(folder_path): if filename.endswith('.json'): json_path = os.path.join(folder_path, filename) # 读取JSON文件 with open(json_path) as file: data = json.load(file) # 获取多边形坐标 shapes = data['shapes'] polygon_points = shapes[0]['points'] # 假设只有一个多边形标注 # 计算最小包围框的左上角和右下角坐标 x_coordinates = [point[0] for point in polygon_points] y_coordinates = [point[1] for point in polygon_points] min_x = min(x_coordinates) min_y = min(y_coordinates) max_x = max(x_coordinates) max_y = max(y_coordinates) # 将坐标信息添加到列表中 data_list.append({'Filename': filename, 'Min_X': min_x, 'Min_Y': min_y, 'Max_X': max_x, 'Max_Y': max_y}) # 写入CSV文件 with open(output_file, 'w', newline='') as file: fieldnames = ['Filename', 'Min_X', 'Min_Y', 'Max_X', 'Max_Y'] writer = csv.DictWriter(file, fieldnames=fieldnames) writer.writeheader() writer.writerows(data_list) # 生成input_prompts input_prompts = [] for data in data_list: input_prompt = f"处理文件:{data['Filename']},左上角坐标:({data['Min_X']}, {data['Min_Y']}),右下角坐标:({data['Max_X']}, {data['Max_Y']})" input_prompts.append(input_prompt) sam = sam_model_registry["default"](checkpoint="D:\\segment-anything-main\\segment-anything-main\\sam_vit_h_4b8939.pth") predictor = SamPredictor(sam) for filename in os.listdir(folder_path): if filename.lower().endswith(('.png', '.jpg', '.jpeg')): image_path = os.path.join(folder_path, filename) # Load and set the image for prediction your_image = cv2.imread(image_path) predictor.set_image(your_image) # Perform prediction using input prompts masks, _, _ = predictor.predict(input_prompts) # Perform further processing or analysis on the predicted masks for i, mask in enumerate(masks): mask_filename = f"mask_{i + 1}_{filename}" mask_path = os.path.join(folder_path, mask_filename) cv2.imwrite(mask_path, mask)
时间: 2024-04-17 22:28:41 浏览: 184
这段代码是一个细胞识别的脚本,它将指定文件夹中的图片进行细胞分割,并将分割后的结果保存为图片和CSV文件。具体的流程如下:
1. 首先,通过读取指定文件夹中的JSON文件,获取细胞的多边形坐标信息,并计算出最小包围框的左上角和右下角坐标。
2. 将坐标信息添加到一个列表中,用于后续写入CSV文件。
3. 使用列表中的坐标信息生成输入提示语句。
4. 加载预训练的细胞识别模型,并创建一个细胞识别器。
5. 遍历指定文件夹中的图片文件,对每张图片进行细胞识别:
a. 加载并设置待预测的图片。
b. 使用输入提示语句进行细胞识别,得到分割后的细胞掩模。
c. 可以对预测的掩模进行后续处理或分析。
d. 将分割后的掩模保存为图片。
请注意,这段代码中使用了第三方库 `segment_anything` 进行细胞分割,你需要先安装该库才能运行这段代码。
相关问题
ModuleNotFoundError Traceback (most recent call last) Cell In[1], line 10 8 from tensorflow.keras.preprocessing.image import load_img 9 from importlib import reload ---> 10 import segmenteverygrain as seg 11 from segment_anything import sam_model_registry, SamAutomaticMaskGenerator, SamPredictor 12 from tqdm import trange File ~\segmenteverygrain-main\segmenteverygrain\segmenteverygrain.py:42 39 from tensorflow.keras.optimizers import Adam 40 from tensorflow.keras.preprocessing.image import load_img ---> 42 from segment_anything import sam_model_registry, SamAutomaticMaskGenerator, SamPredictor 44 def predict_image_tile(im_tile,model): 45 if len(np.shape(im_tile)) == 2: File D:\Anaconda\lib\site-packages\segment_anything\__init__.py:14 1 # Copyright (c) Meta Platforms, Inc. and affiliates. 2 # All rights reserved. 3 4 # This source code is licensed under the license found in the 5 # LICENSE file in the root directory of this source tree. 7 from .build_sam import ( 8 build_sam, 9 build_sam_vit_h, (...) 12 sam_model_registry, 13 ) ---> 14 from .predictor import SamPredictor 15 from .automatic_mask_generator import SamAutomaticMaskGenerator File D:\Anaconda\lib\site-packages\segment_anything\predictor.py:14 10 from segment_anything.modeling import Sam 12 from typing import Optional, Tuple ---> 14 from .utils.transforms import ResizeLongestSide 17 class SamPredictor: 18 def __init__( 19 self, 20 sam_model: Sam, 21 ) -> None: File D:\Anaconda\lib\site-packages\segment_anything\utils\transforms.py:10 8 import torch 9 from torch.nn import functional as F ---> 10 from torchvision.transforms.functional import resize, to_pil_image # type: ignore 12 from copy import deepcopy 13 from typing import Tuple ModuleNotFoundError: No module named 'torchvision'
这个错误是由于缺少 `torchvision` 模块引起的。`torchvision` 是 PyTorch 的一个扩展库,提供了一些图像处理和计算机视觉相关的功能,包括图像变换、数据集加载等。
要解决这个问题,您可以尝试通过运行以下命令来安装 `torchvision`:
```
pip install torchvision
```
如果您正在使用 Anaconda 环境,可以运行以下命令:
```
conda install torchvision -c pytorch
```
安装完毕后,重新运行代码,应该就能成功导入 `torchvision` 模块了。
Cython将.pth模型封装为.so后,能不能在C++里调用这个.so文件,我的封装代码是import sys import numpy as np import matplotlib import matplotlib.pyplot as plt import cv2 sys.path.append("/app/PythonProjects/segment-anything-main") from segment_anything import sam_model_registry, SamAutomaticMaskGenerator, SamPredictor # model.pyxcdef class MyModel: def __init__(self, str model_type,str sam_checkpoint): self.sam = sam_model_registry[model_type](checkpoint=sam_checkpoint) def __callmodel__(self): return self.sam,请给我一篇C++代码
当你使用Cython封装Python代码时,最终会生成一个动态链接库(.so文件)。对于C++来说,你可以使用动态链接库来调用Python函数并传递参数。下面是一个简单的示例代码:
```c++
#include <Python.h>
int main(int argc, char *argv[]) {
Py_Initialize();
// 加载 Python 模块和函数
PyObject* pModule = PyImport_ImportModule("your_module_name");
PyObject* pFunc = PyObject_GetAttrString(pModule, "your_function_name");
// 准备参数
PyObject* pArgs = PyTuple_New(1); // 1 表示参数数量
PyTuple_SetItem(pArgs, 0, PyUnicode_FromString("your_parameter"));
// 调用函数
PyObject* pRetVal = PyObject_CallObject(pFunc, pArgs);
// 处理返回值
if (pRetVal) {
// TODO: 处理返回值
Py_DECREF(pRetVal);
} else {
// TODO: 处理错误
}
// 清理
Py_DECREF(pArgs);
Py_DECREF(pFunc);
Py_DECREF(pModule);
Py_Finalize();
}
```
在上面的示例代码中,你需要做以下几件事情:
1. 在 `main` 函数中初始化 Python 解释器(`Py_Initialize`)和关闭 Python 解释器(`Py_Finalize`)。
2. 使用 `PyImport_ImportModule` 加载 Python 模块,使用 `PyObject_GetAttrString` 获取模块中的函数对象。
3. 创建参数元组对象(`PyTuple_New`),将参数添加到元组中(`PyTuple_SetItem`)。
4. 使用 `PyObject_CallObject` 调用 Python 函数,并传递参数。
5. 处理返回值,清理内存(使用 `Py_DECREF`)。
你需要根据你的具体情况修改上面的代码,并将其编译为可执行文件。注意,你需要在编译时链接 Python 库和你的动态链接库。
阅读全文
相关推荐
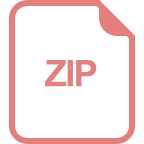
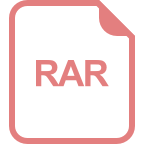














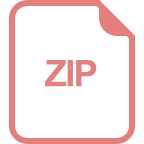