写Python代码实现从NFA (非确定有限状态自动机) 到DFA (确定有限状态自动机),然后对DFA进行最小化的过程
时间: 2024-11-12 19:32:32 浏览: 14
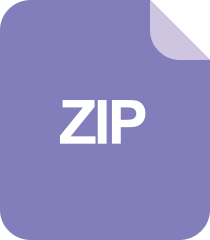
【编译原理实验】NFA确定化与DFA最小化
在Python中,实现从NFA到DFA再到最小化的完整流程比较复杂,因为这涉及到图的理论和状态机的设计。下面是一个简化的示例,仅展示了如何使用`networkx`库进行NFA到DFA的基本转换,并简要描述了最小化过程。对于最小化部分,我们这里只会给出基本的概念,而不做具体实现,因为它通常涉及到一些高级算法如哈密尔顿回路等。
首先安装必要的库(如果还没有安装):
```bash
pip install networkx
```
然后我们可以开始编写代码:
```python
import networkx as nx
from itertools import product
# 定义NFA类
class NFA:
def __init__(self, states, alphabet, transitions):
self.states = set(states)
self.alphabet = set(alphabet)
self.transitions = {state: {char: next_states for char, next_states in transitions[state].items()} for state in states}
# NFA到DFA的转换
def nfa_to_dfa(nfa):
initial_state = list(nfa.states)[0]
dfa = DFA({frozenset([initial_state])}, nfa.alphabet)
q = [frozenset([initial_state])]
while q:
p = q.pop()
for char in nfa.alphabet:
new_q = []
for s in p:
if (char, s) in nfa.transitions:
new_s = frozenset(nfa.transitions[char][s])
if new_s not in dfa.accepting_states and new_s not in dfa.non_accepting_states:
new_q.append(new_s)
if new_s == dfa.accepting_states or new_s.intersection(dfa.accepting_states):
dfa.accepting_states.add(new_s)
else:
new_q.extend([new_s])
q.extend(new_q)
return dfa
# 定义DFA类,但未包含最小化部分
class DFA:
def __init__(self, states, alphabet):
self.states = set(states)
self.accepting_states = set()
self.non_accepting_states = set(self.states) - self.accepting_states
self.alphabet = alphabet
# 最小化DFA(这里省略,你需要了解Myhill-Nerode算法或其他最小化策略)
# def minimize_dfa(dfa):
# ... (留空)
nfa_example = # 创建一个NFA实例
dfa_result = nfa_to_dfa(nfa_example)
minimized_dfa = minimize_dfa(dfa_result) # 这里假设已实现最小化
# 示例中省略了验证和展示结果的部分
```
**相关问题**:
1. 如何理解NFA到DFA转换过程中使用的frozenset?
2. 对于复杂的NFA,这个简单实现能否应对?如果不能,如何改进?
3. DFA最小化之后,它的状态和接受状态有何变化?
阅读全文
相关推荐
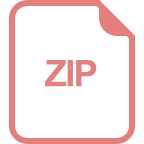
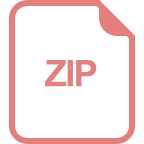
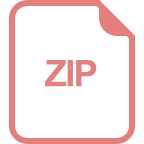

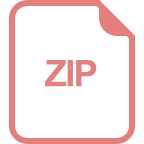
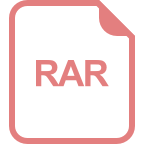
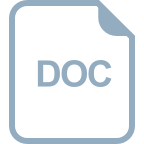
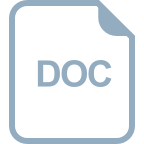
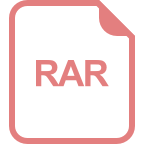
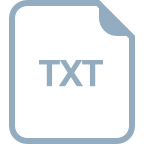
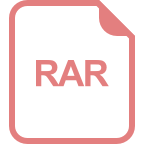
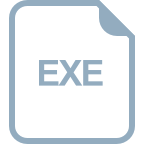
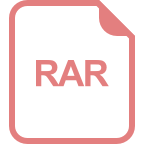
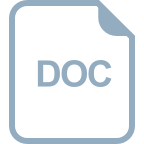
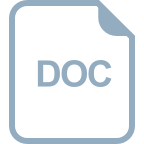
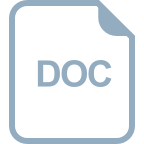

