sample_object_model_3d
时间: 2024-04-16 11:21:00 浏览: 29
sample_object_model_3d是一个用于创建和操作维模型的示例对象模型。它提供了一组类和方法,可以用于加载、编辑和渲染三维模型。
该对象模型通常包含以下几个主要类:
1. Model:表示一个三维模型,包含模型的几何形状、材质和纹理等信息。可以通过该类的方法进行模型的加载、保存和渲染操作。
2. Mesh:表示模型的几何形状,由一系列顶点、面和法线等组成。可以通过该类的方法进行顶点和面的添加、删除和修改操作。
3. Material:表示模型的材质,包含颜色、纹理和光照等属性。可以通过该类的方法进行材质的设置和修改操作。
4. Texture:表示模型的纹理,可以用于给模型表面添加图案或者贴图。可以通过该类的方法进行纹理的加载和映射操作。
除了上述主要类之外,还可能包含其他辅助类和方法,用于处理模型的动画、碰撞检测、光照计算等功能。
使用sample_object_model_3d,你可以创建自己的三维模型,并对其进行各种操作,如旋转、缩放、平移等。同时,你还可以将模型导出为常见的三维文件格式,如OBJ、FBX等,以便在其他三维软件中使用或分享。
相关问题
AttributeError: 'OPTICS' object has no attribute 'core_sample_indices_'
这个错误提示表明OPTICS类没有core_sample_indices_属性。在scikit-learn 0.20版本之前,是可以通过`core_sample_indices_`属性获取OPTICS算法的核心点的。但是在0.20版本之后,这个属性被废弃了。
如果你使用的是0.20版本或更高版本的scikit-learn,可以通过下面的代码获取OPTICS算法的核心点:
```python
import numpy as np
from sklearn.cluster import OPTICS, cluster_optics_dbscan
# 生成随机数据集
np.random.seed(0)
n_points_per_cluster = 250
C1 = [-5, -2] + .8 * np.random.randn(n_points_per_cluster, 2)
C2 = [4, -1] + .1 * np.random.randn(n_points_per_cluster, 2)
C3 = [1, -2] + .2 * np.random.randn(n_points_per_cluster, 2)
C4 = [-2, 3] + .3 * np.random.randn(n_points_per_cluster, 2)
C5 = [3, -2] + .3 * np.random.randn(n_points_per_cluster, 2)
C6 = [5, 6] + .2 * np.random.randn(n_points_per_cluster, 2)
X = np.vstack((C1, C2, C3, C4, C5, C6))
# 进行OPTICS聚类
optics_model = OPTICS(min_samples=50, xi=.05, min_cluster_size=.05)
optics_model.fit(X)
# 根据聚类结果获取核心点
core_samples_mask = np.zeros_like(optics_model.labels_, dtype=bool)
core_samples_mask[optics_model.ordering_] = True
core_samples = optics_model._index[core_samples_mask]
print(core_samples)
```
在这个例子中,我们使用了`optics_model._index`来获取数据中每个点的索引,然后使用`core_samples_mask`获取核心点的索引。最后,通过`core_samples`获取核心点的坐标。
需要注意的是,使用内部属性时需要注意版本的兼容性,可能会存在不同版本之间的差异。另外,使用`_`开头的内部属性是不建议的,因为它们可能会在未来的版本中发生改变。
opencv find_shape_model
The OpenCV function for finding a shape model is cv::createShapeContextDistanceExtractor().
The createShapeContextDistanceExtractor() function creates an instance of a Shape Context Distance Extractor object. This object can be used to compare the shape of two images or contours using the Shape Context algorithm.
The Shape Context algorithm is a method for describing the shape of an object using a series of descriptors. These descriptors are based on the distribution of points on the object's contour relative to a set of reference points.
To use the createShapeContextDistanceExtractor() function, you need to provide the following parameters:
- The number of points to sample on the contour
- The number of angles to use for the Shape Context descriptors
- The radius of the circular region around each reference point used for computing the descriptors
Once you have created the Shape Context Distance Extractor object, you can use its compare() method to compare the shape of two images or contours. The compare() method returns a distance value that indicates how similar the two shapes are.
Here's an example of how to use the createShapeContextDistanceExtractor() function to compare the shape of two contours:
```
// Load two contours from file
std::vector<cv::Point> contour1, contour2;
cv::FileStorage fs("contours.yml", cv::FileStorage::READ);
fs["contour1"] >> contour1;
fs["contour2"] >> contour2;
fs.release();
// Create a Shape Context Distance Extractor object
cv::Ptr<cv::ShapeContextDistanceExtractor> extractor = cv::createShapeContextDistanceExtractor();
// Compute the distance between the two contours
float distance = extractor->compare(contour1, contour2);
// Print the distance
std::cout << "Distance: " << distance << std::endl;
```
In this example, the two contours are loaded from a file and then compared using the Shape Context Distance Extractor object. The distance between the two contours is then printed to the console.
相关推荐
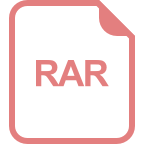
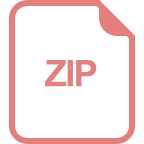
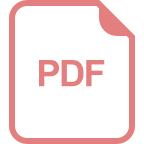












