MADDPG pytorch
时间: 2023-11-15 11:59:18 浏览: 374
MADDPG pytorch是一个基于PyTorch框架实现的多智能体强化学习算法。它是一种深度强化学习算法,可以用于解决多智能体协作问题。MADDPG pytorch的实现可以在GitHub上找到,同时也提供了环境配置过程和使用方法。在使用MADDPG pytorch之前,需要先激活Python3.6环境,并将MPE环境下载并解压到虚拟环境中。然后,将MPE环境中的multiagent文件夹拷贝到MADDPG工程目录下,并在PyCharm中将common文件夹Mark Directory as Source Root,以便MADDPG工程能够较好地调用multiagent中创建的环境。最后,运行main即可开始使用MADDPG pytorch进行多智能体协作问题的解决。
相关问题
maddpg pytorch
你的问题是关于 MADDPG 在 PyTorch 中的实现吗?如果是的话,我可以提供一些信息。MADDPG 是多智能体强化学习算法(Multi-Agent Deep Deterministic Policy Gradient),它是 DDPG(Deep Deterministic Policy Gradient)算法的一个扩展。在 PyTorch 中实现 MADDPG 算法时,可以参考一些开源代码,例如 OpenAI 的 MADDPG 实现(https://github.com/openai/maddpg)或者 Udacity 的 DRLND 课程中的 MADDPG 项目(https://github.com/udacity/deep-reinforcement-learning/tree/master/multi-agent)。这些代码提供了 PyTorch 中实现 MADDPG 算法的参考,你可以根据需要进行修改和调整。
MADDPG的pytorch代码案例
以下是使用 PyTorch 实现的 MADDPG 代码示例:
```
import torch
import torch.nn as nn
import torch.optim as optim
import numpy as np
device = torch.device("cuda" if torch.cuda.is_available() else "cpu")
# 定义 Actor 和 Critic 网络
class Actor(nn.Module):
def __init__(self, state_dim, action_dim, hidden_dim):
super(Actor, self).__init__()
self.fc1 = nn.Linear(state_dim, hidden_dim)
self.fc2 = nn.Linear(hidden_dim, hidden_dim)
self.fc3 = nn.Linear(hidden_dim, action_dim)
def forward(self, state):
x = torch.relu(self.fc1(state))
x = torch.relu(self.fc2(x))
x = torch.tanh(self.fc3(x))
return x
class Critic(nn.Module):
def __init__(self, state_dim, action_dim, hidden_dim):
super(Critic, self).__init__()
self.fc1 = nn.Linear(state_dim + action_dim, hidden_dim)
self.fc2 = nn.Linear(hidden_dim, hidden_dim)
self.fc3 = nn.Linear(hidden_dim, 1)
def forward(self, state, action):
x = torch.cat([state, action], dim=1)
x = torch.relu(self.fc1(x))
x = torch.relu(self.fc2(x))
x = self.fc3(x)
return x
# 定义 MADDPG 算法类
class MADDPG:
def __init__(self, n_agents, state_dim, action_dim, hidden_dim, discount_factor=0.99, tau=0.01, critic_lr=0.001, actor_lr=0.001):
self.n_agents = n_agents
self.state_dim = state_dim
self.action_dim = action_dim
self.hidden_dim = hidden_dim
self.discount_factor = discount_factor
self.tau = tau
self.critic_local = [Critic(state_dim * n_agents, action_dim * n_agents, hidden_dim).to(device) for i in range(n_agents)]
self.critic_target = [Critic(state_dim * n_agents, action_dim * n_agents, hidden_dim).to(device) for i in range(n_agents)]
self.actor_local = [Actor(state_dim, action_dim, hidden_dim).to(device) for i in range(n_agents)]
self.actor_target = [Actor(state_dim, action_dim, hidden_dim).to(device) for i in range(n_agents)]
self.critic_optim = [optim.Adam(self.critic_local[i].parameters(), lr=critic_lr) for i in range(n_agents)]
self.actor_optim = [optim.Adam(self.actor_local[i].parameters(), lr=actor_lr) for i in range(n_agents)]
self.memory = ReplayBuffer()
def act(self, state):
actions = []
for i in range(self.n_agents):
state_tensor = torch.tensor(state[i], dtype=torch.float32).unsqueeze(0).to(device)
action_tensor = self.actor_local[i](state_tensor).detach().cpu().numpy()[0]
actions.append(action_tensor)
return np.array(actions)
def step(self, state, action, reward, next_state, done):
self.memory.add(state, action, reward, next_state, done)
if len(self.memory) > BATCH_SIZE:
experiences = self.memory.sample()
self.learn(experiences)
def learn(self, experiences):
states, actions, rewards, next_states, dones = experiences
for i in range(self.n_agents):
states_i = states.reshape(-1, self.state_dim)[i::self.n_agents]
actions_i = actions.reshape(-1, self.action_dim)[i::self.n_agents]
rewards_i = rewards[:, i].reshape(-1, 1)
next_states_i = next_states.reshape(-1, self.state_dim)[i::self.n_agents]
dones_i = dones[:, i].reshape(-1, 1)
# 计算 Q_target
actions_next = []
for j in range(self.n_agents):
next_states_j = next_states.reshape(-1, self.state_dim)[j::self.n_agents]
action_next_j = self.actor_target[j](next_states_j).detach().cpu().numpy()
actions_next.append(action_next_j)
actions_next = np.stack(actions_next).transpose()
q_next = self.critic_target[i](torch.tensor(next_states_i, dtype=torch.float32).to(device),
torch.tensor(actions_next, dtype=torch.float32).to(device))
q_target_i = rewards_i + (self.discount_factor * q_next * (1 - dones_i))
# 计算 Critic loss
q_local_i = self.critic_local[i](torch.tensor(states_i, dtype=torch.float32).to(device),
torch.tensor(actions_i, dtype=torch.float32).to(device))
critic_loss_i = nn.MSELoss()(q_local_i, q_target_i.detach())
# 更新 Critic 网络
self.critic_optim[i].zero_grad()
critic_loss_i.backward()
self.critic_optim[i].step()
# 计算 Actor loss
actions_pred = []
for j in range(self.n_agents):
states_j = states.reshape(-1, self.state_dim)[j::self.n_agents]
actions_pred_j = self.actor_local[j](torch.tensor(states_j, dtype=torch.float32).to(device))
if j == i:
actions_pred_i = actions_pred_j
else:
actions_pred.append(actions_pred_j.detach().cpu().numpy())
actions_pred.append(actions_pred_i.detach().cpu().numpy())
actions_pred = np.stack(actions_pred).transpose()
actor_loss_i = -self.critic_local[i](torch.tensor(states_i, dtype=torch.float32).to(device),
torch.tensor(actions_pred, dtype=torch.float32).to(device)).mean()
# 更新 Actor 网络
self.actor_optim[i].zero_grad()
actor_loss_i.backward()
self.actor_optim[i].step()
# 软更新 Critic 和 Actor 目标网络
self.soft_update(self.critic_local[i], self.critic_target[i], self.tau)
self.soft_update(self.actor_local[i], self.actor_target[i], self.tau)
def soft_update(self, local_model, target_model, tau):
for target_param, local_param in zip(target_model.parameters(), local_model.parameters()):
target_param.data.copy_(tau * local_param.data + (1.0 - tau) * target_param.data)
# 定义 ReplayBuffer 类
class ReplayBuffer:
def __init__(self, buffer_size=int(1e6)):
self.buffer_size = buffer_size
self.buffer = []
self.position = 0
def add(self, state, action, reward, next_state, done):
if len(self.buffer) < self.buffer_size:
self.buffer.append(None)
self.buffer[self.position] = (state, action, reward, next_state, done)
self.position = (self.position + 1) % self.buffer_size
def sample(self, batch_size=128):
batch = zip(*random.sample(self.buffer, batch_size))
return [torch.tensor(item, dtype=torch.float32).to(device) for item in batch]
def __len__(self):
return len(self.buffer)
# 定义训练函数
def train(agent, env, n_episodes=5000, max_t=1000):
scores = []
for i_episode in range(1, n_episodes+1):
env_info = env.reset(train_mode=True)[brain_name]
states = env_info.vector_observations
agent.reset()
score = np.zeros(num_agents)
for t in range(max_t):
actions = agent.act(states)
env_info = env.step(actions)[brain_name]
next_states = env_info.vector_observations
rewards = env_info.rewards
dones = env_info.local_done
agent.step(states, actions, rewards, next_states, dones)
states = next_states
score += rewards
if np.any(dones):
break
scores.append(np.max(score))
print('\rEpisode {}\tScore: {:.2f}'.format(i_episode, np.max(score)), end="")
if i_episode % 100 == 0:
print('\rEpisode {}\tAverage Score: {:.2f}'.format(i_episode, np.mean(scores[-100:])))
if np.mean(scores[-100:]) >= 0.5:
print('\nEnvironment solved in {:d} episodes!\tAverage Score: {:.2f}'.format(i_episode-100, np.mean(scores[-100:])))
torch.save(agent.actor_local.state_dict(), 'checkpoint_actor.pth')
torch.save(agent.critic_local.state_dict(), 'checkpoint_critic.pth')
break
# 创建 MADDPG 实例并训练
agent = MADDPG(n_agents=num_agents, state_dim=state_size, action_dim=action_size, hidden_dim=128)
train(agent, env)
```
以上代码示例中,Actor 和 Critic 网络均为全连接神经网络,其中 Actor 网络输出动作值,并使用 tanh 函数对动作进行了缩放。MADDPG 类定义了 MADDPG 算法的实现方法,其中包括了 Actor 和 Critic 的本地网络和目标网络,并定义了学习和软更新方法。ReplayBuffer 类实现了经验回放缓存。训练函数 train 利用以上类来实现 MADDPG 算法的训练。
阅读全文
相关推荐
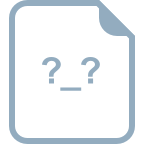
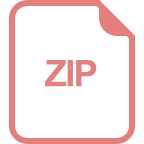
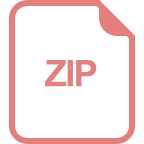


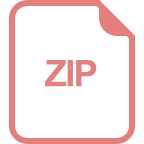
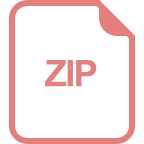
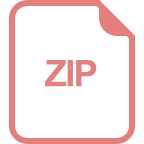
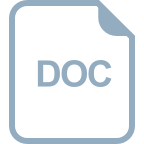

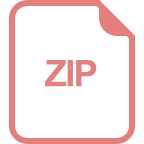
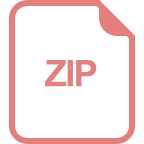
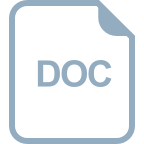
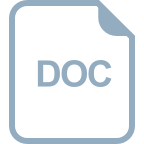
