java并行计算圆周率
时间: 2023-11-10 18:56:36 浏览: 53
要在Java中进行并行计算圆周率,可以使用多线程和并行算法。下面是一种常见的方法:
1. 首先,将圆周率计算问题分解为多个子问题,每个子问题计算一部分圆周率值。
2. 使用Java的多线程机制,创建一个线程池,并将每个子问题分配给一个线程进行计算。
3. 每个线程独立计算其分配的子问题,并累积最终的结果。
4. 最后,将所有线程的结果合并,得出最终的圆周率值。
这种方法充分利用了多核处理器的并行能力,可以加速圆周率的计算过程。
相关问题
怎么在java里计算圆周率
在Java中计算圆周率可以使用蒙特卡罗方法或者使用数学公式。下面给出两种方法的示例代码:
1. 蒙特卡罗方法
```java
import java.util.Random;
public class CalculatePi {
public static void main(String[] args) {
Random random = new Random();
int n = 10000000; // 投掷点数
int count = 0; // 落在圆内的点数
for (int i = 0; i < n; i++) {
double x = random.nextDouble();
double y = random.nextDouble();
if (x * x + y * y < 1) {
count++;
}
}
double pi = 4.0 * count / n;
System.out.println("圆周率的近似值为:" + pi);
}
}
```
2. 数学公式
```java
public class CalculatePi {
public static void main(String[] args) {
int n = 1000000; // 迭代次数
double pi = 0;
for (int i = 0; i < n; i++) {
pi += Math.pow(-1, i) / (2 * i + 1);
}
pi *= 4;
System.out.println("圆周率的近似值为:" + pi);
}
}
```
以上两种方法都是近似计算圆周率的方法,实际应用中需要根据精度和效率要求选择合适的方法。
用java 计算圆周率
可以使用蒙特卡罗方法来计算圆周率,具体步骤如下:
1. 在一个正方形内画一个内切圆,圆心为正方形中心。
2. 在正方形内随机生成大量的点。
3. 统计落在内切圆内的点的数量。
4. 计算圆周率的近似值:π ≈ 4 * (落在内切圆内的点的数量) / (正方形内生成的点的总数)。
以下是一个 Java 代码示例:
```java
import java.util.Random;
public class CalculatePi {
public static void main(String[] args) {
int totalPoints = 1000000; // 生成点的总数
int pointsInCircle = 0; // 落在内切圆内的点的数量
Random rand = new Random();
for (int i = 0; i < totalPoints; i++) {
// 生成随机点的 x, y 坐标
double x = rand.nextDouble();
double y = rand.nextDouble();
// 判断该点是否落在内切圆内
if (x * x + y * y <= 1) {
pointsInCircle++;
}
}
// 计算圆周率的近似值
double pi = 4.0 * pointsInCircle / totalPoints;
System.out.println("Approximate value of pi: " + pi);
}
}
```
注意:这种方法只能得到圆周率的近似值,精度随生成的点的数量增加而提高。
相关推荐
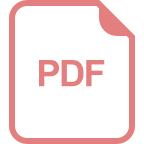
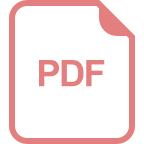












