pytorch使用一维卷积神经网络进行二分类
时间: 2023-06-30 20:08:13 浏览: 305
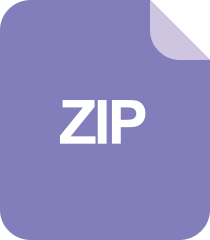
基于Pytorch的二维卷积神经网络手写数字识别功能实
一维卷积神经网络可以用于处理序列数据,如时间序列或文本数据。对于二分类问题,可以在网络的输出层使用一个sigmoid激活函数,输出一个0到1之间的概率值,表示该样本属于正类的概率。
下面是一个使用PyTorch实现的一维卷积神经网络进行二分类的示例:
```python
import torch
import torch.nn as nn
import torch.optim as optim
class Net(nn.Module):
def __init__(self):
super(Net, self).__init__()
self.conv1 = nn.Conv1d(in_channels=1, out_channels=16, kernel_size=3)
self.pool1 = nn.MaxPool1d(kernel_size=2)
self.conv2 = nn.Conv1d(in_channels=16, out_channels=32, kernel_size=3)
self.pool2 = nn.MaxPool1d(kernel_size=2)
self.fc1 = nn.Linear(in_features=32*23, out_features=128)
self.fc2 = nn.Linear(in_features=128, out_features=1)
self.sigmoid = nn.Sigmoid()
def forward(self, x):
x = self.conv1(x)
x = nn.functional.relu(x)
x = self.pool1(x)
x = self.conv2(x)
x = nn.functional.relu(x)
x = self.pool2(x)
x = x.view(-1, 32*23)
x = self.fc1(x)
x = nn.functional.relu(x)
x = self.fc2(x)
x = self.sigmoid(x)
return x
# 训练数据和标签
x_train = torch.randn(100, 1, 50)
y_train = torch.randint(low=0, high=2, size=(100, 1))
# 定义网络模型、损失函数、优化器
net = Net()
criterion = nn.BCELoss()
optimizer = optim.Adam(net.parameters(), lr=0.001)
# 训练
for epoch in range(100):
optimizer.zero_grad()
y_pred = net(x_train)
loss = criterion(y_pred, y_train.float())
loss.backward()
optimizer.step()
print(f"Epoch {epoch+1}: Loss = {loss.item():.4f}")
# 测试
x_test = torch.randn(10, 1, 50)
y_test = torch.randint(low=0, high=2, size=(10, 1))
with torch.no_grad():
y_pred = net(x_test)
predicted = torch.round(y_pred)
acc = (predicted == y_test.float()).sum().item() / len(y_test)
print(f"Test Accuracy: {acc:.4f}")
```
这个示例中,我们定义了一个包含两个卷积层和两个全连接层的神经网络,最后使用sigmoid函数输出一个概率值。在训练时,我们使用二分类交叉熵损失函数和Adam优化器。在测试时,我们计算模型的准确率。
阅读全文
相关推荐
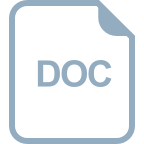
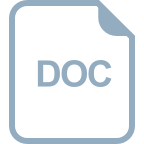

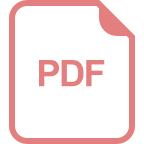
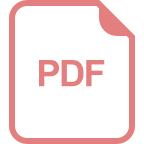
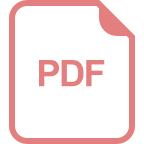
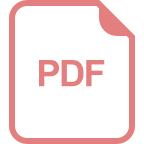
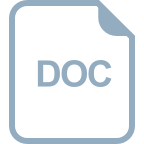
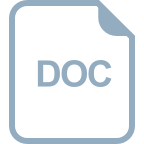
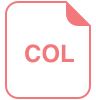
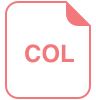






