pointwise 例子
时间: 2023-11-08 22:03:08 浏览: 311
Pointwise是一个将有限元数据进行离散化和插值的软件程序。它被广泛应用于CAD建模、流体动力学、有限元分析和声学等领域。以下是一个关于Pointwise的例子:
在航空航天工程中,Pointwise可以被用来生成飞机的外形轮廓。首先,航空工程师会根据飞机的设计需求和性能要求利用CAD软件绘制飞机的三维模型。然后,将该模型导入到Pointwise中进行处理。
在Pointwise中,航空工程师可以定义不同的几何参数,例如翼展、燃油箱位置和机翼弯曲等,并将这些参数应用到飞机的外形轮廓上。通过调整这些参数,航空工程师可以对飞机进行快速的设计迭代,以优化飞机的性能。
接下来,航空工程师会选择适当的网格生成方法。Pointwise提供了多种网格生成算法,例如结构化网格生成、非结构化网格生成和混合网格生成等。航空工程师可以根据飞机的复杂性和分析要求选择合适的算法。
最后,航空工程师可以在Pointwise中生成飞机的网格。该网格可以用于后续的流体动力学模拟,以评估飞机在不同飞行条件下的气动性能。
通过Pointwise,航空工程师可以高效地进行飞机的几何建模和网格生成。这样的过程不仅可以提高设计效率,还可以减少飞机设计中的错误和不确定性,从而更好地满足飞机设计的要求。
相关问题
有没有transformer的例子
是的,以下是一个使用Transformer模型进行文本分类的示例:
```python
import tensorflow as tf
import tensorflow_datasets as tfds
# 加载IMDB数据集
(train_data, test_data), info = tfds.load('imdb_reviews/subwords8k',
split=(tfds.Split.TRAIN, tfds.Split.TEST),
with_info=True, as_supervised=True)
encoder = info.features['text'].encoder
BUFFER_SIZE = 10000
BATCH_SIZE = 64
# 训练数据和测试数据预处理
padded_shapes = ([None], ())
train_batches = (train_data.shuffle(BUFFER_SIZE).padded_batch(BATCH_SIZE, padded_shapes=padded_shapes))
test_batches = (test_data.padded_batch(BATCH_SIZE, padded_shapes=padded_shapes))
# Transformer模型定义
class TransformerModel(tf.keras.Model):
def __init__(self, num_layers, d_model, num_heads, dff, input_vocab_size, target_vocab_size, dropout_rate=0.1):
super(TransformerModel, self).__init__()
self.encoder = tf.keras.layers.Embedding(input_vocab_size, d_model)
self.pos_encoding = positional_encoding(input_vocab_size, d_model)
self.transformer_blocks = [TransformerBlock(d_model, num_heads, dff, dropout_rate) for _ in range(num_layers)]
self.dropout = tf.keras.layers.Dropout(dropout_rate)
self.final_layer = tf.keras.layers.Dense(target_vocab_size)
def call(self, inputs, training):
input_seq, input_mask = inputs
input_emb = self.encoder(input_seq)
input_emb *= tf.math.sqrt(tf.cast(self.encoder.embedding_dim, tf.float32))
input_emb += self.pos_encoding[:input_emb.shape[1], :]
x = self.dropout(input_emb, training=training)
for i in range(len(self.transformer_blocks)):
x = self.transformer_blocks[i](x, input_mask, training)
x = tf.reduce_mean(x, axis=1)
x = self.final_layer(x)
return x
# Transformer块定义
class TransformerBlock(tf.keras.layers.Layer):
def __init__(self, d_model, num_heads, dff, dropout_rate=0.1):
super(TransformerBlock, self).__init__()
self.multi_head_attention = MultiHeadAttention(d_model, num_heads)
self.feed_forward_network = point_wise_feed_forward_network(d_model, dff)
self.layer_norm1 = tf.keras.layers.LayerNormalization(epsilon=1e-6)
self.layer_norm2 = tf.keras.layers.LayerNormalization(epsilon=1e-6)
self.dropout1 = tf.keras.layers.Dropout(dropout_rate)
self.dropout2 = tf.keras.layers.Dropout(dropout_rate)
def call(self, x, mask, training):
attn_output, _ = self.multi_head_attention(x, x, x, mask)
attn_output = self.dropout1(attn_output, training=training)
out1 = self.layer_norm1(x + attn_output)
ffn_output = self.feed_forward_network(out1)
ffn_output = self.dropout2(ffn_output, training=training)
out2 = self.layer_norm2(out1 + ffn_output)
return out2
# 多头注意力机制定义
class MultiHeadAttention(tf.keras.layers.Layer):
def __init__(self, d_model, num_heads):
super(MultiHeadAttention, self).__init__()
self.num_heads = num_heads
self.d_model = d_model
assert d_model % self.num_heads == 0
self.depth = d_model // self.num_heads
self.wq = tf.keras.layers.Dense(d_model)
self.wk = tf.keras.layers.Dense(d_model)
self.wv = tf.keras.layers.Dense(d_model)
self.dense = tf.keras.layers.Dense(d_model)
def split_heads(self, x, batch_size):
x = tf.reshape(x, (batch_size, -1, self.num_heads, self.depth))
return tf.transpose(x, perm=[0, 2, 1, 3])
def call(self, q, k, v, mask):
batch_size = tf.shape(q)[0]
q = self.wq(q)
k = self.wk(k)
v = self.wv(v)
q = self.split_heads(q, batch_size)
k = self.split_heads(k, batch_size)
v = self.split_heads(v, batch_size)
scaled_attention, attention_weights = scaled_dot_product_attention(q, k, v, mask)
scaled_attention = tf.transpose(scaled_attention, perm=[0, 2, 1, 3])
concat_attention = tf.reshape(scaled_attention, (batch_size, -1, self.d_model))
output = self.dense(concat_attention)
return output, attention_weights
# 点式前馈网络定义
def point_wise_feed_forward_network(d_model, dff):
return tf.keras.Sequential([
tf.keras.layers.Dense(dff, activation='relu'),
tf.keras.layers.Dense(d_model)
])
# 编码器位置编码定义
def get_angles(pos, i, d_model):
angle_rates = 1 / np.power(10000, (2 * (i // 2)) / np.float32(d_model))
return pos * angle_rates
def positional_encoding(position, d_model):
angle_rads = get_angles(np.arange(position)[:, np.newaxis], np.arange(d_model)[np.newaxis, :], d_model)
angle_rads[:, 0::2] = np.sin(angle_rads[:, 0::2])
angle_rads[:, 1::2] = np.cos(angle_rads[:, 1::2])
pos_encoding = angle_rads[np.newaxis, ...]
return tf.cast(pos_encoding, dtype=tf.float32)
# 损失函数定义
loss_object = tf.keras.losses.BinaryCrossentropy(from_logits=True)
def loss_function(real, pred):
mask = tf.math.logical_not(tf.math.equal(real, 0))
loss_ = loss_object(real, pred)
mask = tf.cast(mask, dtype=loss_.dtype)
loss_ *= mask
return tf.reduce_mean(loss_)
# 评估指标定义
train_loss = tf.keras.metrics.Mean(name='train_loss')
train_accuracy = tf.keras.metrics.BinaryAccuracy(name='train_accuracy')
test_loss = tf.keras.metrics.Mean(name='test_loss')
test_accuracy = tf.keras.metrics.BinaryAccuracy(name='test_accuracy')
# 模型训练
EPOCHS = 10
num_layers = 4
d_model = 128
num_heads = 8
dff = 512
dropout_rate = 0.1
input_vocab_size = encoder.vocab_size
target_vocab_size = 2
transformer = TransformerModel(num_layers, d_model, num_heads, dff, input_vocab_size, target_vocab_size, dropout_rate)
optimizer = tf.keras.optimizers.Adam()
for epoch in range(EPOCHS):
train_loss.reset_states()
train_accuracy.reset_states()
test_loss.reset_states()
test_accuracy.reset_states()
for (batch, (input_seq, target)) in enumerate(train_batches):
input_mask = tf.math.logical_not(tf.math.equal(input_seq, 0))
target = tf.expand_dims(target, axis=-1)
with tf.GradientTape() as tape:
predictions = transformer((input_seq, input_mask), True)
loss = loss_function(target, predictions)
gradients = tape.gradient(loss, transformer.trainable_variables)
optimizer.apply_gradients(zip(gradients, transformer.trainable_variables))
train_loss(loss)
train_accuracy(target, predictions)
if batch % 100 == 0:
print('Epoch {} Batch {} Train Loss {:.4f} Train Accuracy {:.4f}'.format(
epoch + 1, batch, train_loss.result(), train_accuracy.result()))
for (batch, (input_seq, target)) in enumerate(test_batches):
input_mask = tf.math.logical_not(tf.math.equal(input_seq, 0))
target = tf.expand_dims(target, axis=-1)
predictions = transformer((input_seq, input_mask), False)
loss = loss_function(target, predictions)
test_loss(loss)
test_accuracy(target, predictions)
print('Epoch {} Test Loss {:.4f} Test Accuracy {:.4f}'.format(
epoch + 1, test_loss.result(), test_accuracy.result()))
```
这个示例使用Transformer模型来对IMDB电影评论进行情感分析。它使用TensorFlow Datasets中的IMDB数据集,将每个评论进行编码并将其输入到Transformer模型中,以预测评论的情感(正面或负面)。
decoder的key_pos是如何使用,能否提供代码例子
在ChitGPT中,Decoder的key_pos是通过以下方式使用的:
首先,我们需要在Decoder的初始化函数中定义key_pos:
```python
class Decoder(nn.Module):
def __init__(self, num_layers, d_model, num_heads, dff, vocab_size, max_seq_len, rate=0.1):
super(Decoder, self).__init__()
self.d_model = d_model
self.num_layers = num_layers
self.embedding = nn.Embedding(vocab_size, d_model)
self.pos_encoding = positional_encoding(max_seq_len, d_model)
self.dec_layers = [DecoderLayer(d_model, num_heads, dff, rate) for _ in range(num_layers)]
self.dropout = nn.Dropout(rate)
self.final_layer_norm = nn.LayerNorm(d_model)
# Define key_pos
self.key_pos = torch.arange(0, max_seq_len, dtype=torch.long).unsqueeze(0)
def forward(self, x, enc_output, look_ahead_mask, padding_mask):
seq_len = x.size(1)
attention_weights = {}
# Adding embedding and position encoding.
x = self.embedding(x) # (batch_size, target_seq_len, d_model)
x *= torch.sqrt(torch.FloatTensor([self.d_model])).to(x.device)
x += self.pos_encoding[:, :seq_len, :]
# Applying dropout to the input of the decoder.
x = self.dropout(x)
for i in range(self.num_layers):
x, block1, block2 = self.dec_layers[i](x, enc_output, look_ahead_mask, padding_mask)
# Save attention weights
attention_weights[f'decoder_layer{i+1}_block1'] = block1
attention_weights[f'decoder_layer{i+1}_block2'] = block2
# Applying a final layer normalization for decoder output.
x = self.final_layer_norm(x)
return x, attention_weights
```
然后,在DecoderLayer中,我们可以使用key_pos来计算注意力分数:
```python
class DecoderLayer(nn.Module):
def __init__(self, d_model, num_heads, dff, rate=0.1):
super(DecoderLayer, self).__init__()
self.mha1 = MultiHeadAttention(d_model, num_heads)
self.mha2 = MultiHeadAttention(d_model, num_heads)
self.ffn = point_wise_feed_forward_network(d_model, dff)
self.layer_norm1 = nn.LayerNorm(d_model)
self.layer_norm2 = nn.LayerNorm(d_model)
self.layer_norm3 = nn.LayerNorm(d_model)
self.dropout1 = nn.Dropout(rate)
self.dropout2 = nn.Dropout(rate)
self.dropout3 = nn.Dropout(rate)
def forward(self, x, enc_output, look_ahead_mask, padding_mask):
# Multi-head attention layer with masking for decoder self-attention
attn1, block1 = self.mha1(x, x, x, look_ahead_mask)
attn1 = self.dropout1(attn1)
out1 = self.layer_norm1(attn1 + x)
# Multi-head attention layer with padding masking for encoder-decoder attention
key_pos = self.mha2.decoder_key_pos.unsqueeze(0)
attn2, block2 = self.mha2(out1, enc_output, enc_output, padding_mask, key_pos=key_pos)
attn2 = self.dropout2(attn2)
out2 = self.layer_norm2(attn2 + out1)
# Point wise feed forward network
ffn_output = self.ffn(out2)
ffn_output = self.dropout3(ffn_output)
out3 = self.layer_norm3(ffn_output + out2)
return out3, block1, block2
```
在这个例子中,我们使用 `self.mha2` 来计算Decoder与Encoder之间的注意力,其中 `key_pos` 是 `self.mha2` 中的一个参数,它被设置为 `self.mha2.decoder_key_pos.unsqueeze(0)`,这将 `key_pos` 转换为一个形状为 `(1, max_seq_len)` 的张量,从而与encoder输出的形状相同。在计算注意力分数时,`key_pos` 用于查找encoder输出中每个位置的位置编码,以便在进行注意力计算时使用。
阅读全文
相关推荐
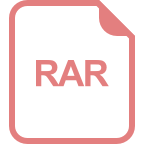
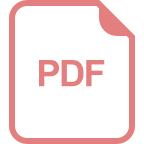
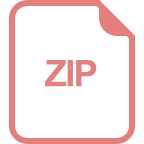









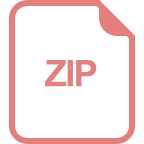
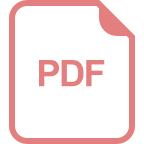
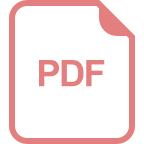
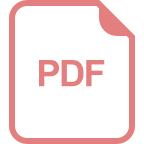
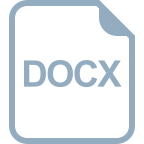