transformer源代码
时间: 2023-10-02 16:13:56 浏览: 62
Transformer模型的源代码如下所示:
```python
class Transformer(nn.Module):
def __init__(self):
super(Transformer, self).__init__()
self.encoder = Encoder() # 编码层
self.decoder = Decoder() # 解码层
self.projection = nn.Linear(d_model, tgt_vocab_size, bias=False) # 输出层
def forward(self, enc_inputs, dec_inputs):
# 这里有两个数据进行输入,一个是enc_inputs 形状为[batch_size, src_len],主要是作为编码段的输入
# 一个dec_inputs,形状为[batch_size, tgt_len],主要是作为解码端的输入
# 以下是转置代码
q_s = self.W_Q(Q).view(batch_size, -1, n_heads, d_k).transpose(1, 2)
# 输出dec_outputs[batch_size, tgt_len, d_model] positionalEncodding位置编码
```
相关问题
Transformer源代码
Transformer是一种基于自注意力机制的神经网络模型,用于序列到序列的任务,如机器翻译、文本摘要等。以下是Transformer的PyTorch源代码:
```python
import torch
import torch.nn as nn
import torch.nn.functional as F
class Transformer(nn.Module):
def __init__(self, input_dim, output_dim, hidden_dim, num_layers, num_heads, dropout):
super().__init__()
self.embedding = nn.Embedding(input_dim, hidden_dim)
self.pos_encoding = PositionalEncoding(hidden_dim, dropout)
self.layers = nn.ModuleList([TransformerLayer(hidden_dim, num_heads, dropout) for _ in range(num_layers)])
self.fc_out = nn.Linear(hidden_dim, output_dim)
def forward(self, src):
# src: [batch_size, src_len]
embedded = self.embedding(src) * math.sqrt(self.hidden_dim)
embedded = self.pos_encoding(embedded)
for layer in self.layers:
embedded = layer(embedded)
output = self.fc_out(embedded[:, 0])
return output
class TransformerLayer(nn.Module):
def __init__(self, hidden_dim, num_heads, dropout):
super().__init__()
self.self_attn = MultiHeadAttention(hidden_dim, num_heads)
self.norm1 = nn.LayerNorm(hidden_dim)
self.ff = FeedForward(hidden_dim)
self.norm2 = nn.LayerNorm(hidden_dim)
self.dropout = nn.Dropout(dropout)
def forward(self, src):
# src: [batch_size, src_len, hidden_dim]
attn_output, _ = self.self_attn(src, src, src)
src = self.norm1(src + self.dropout(attn_output))
ff_output = self.ff(src)
src = self.norm2(src + self.dropout(ff_output))
return src
class MultiHeadAttention(nn.Module):
def __init__(self, hidden_dim, num_heads):
super().__init__()
self.hidden_dim = hidden_dim
self.num_heads = num_heads
self.head_dim = hidden_dim // num_heads
self.fc_q = nn.Linear(hidden_dim, hidden_dim)
self.fc_k = nn.Linear(hidden_dim, hidden_dim)
self.fc_v = nn.Linear(hidden_dim, hidden_dim)
self.fc_o = nn.Linear(hidden_dim, hidden_dim)
def forward(self, query, key, value):
# query: [batch_size, query_len, hidden_dim]
# key: [batch_size, key_len, hidden_dim]
# value: [batch_size, value_len, hidden_dim]
batch_size = query.shape[0]
Q = self.fc_q(query).view(batch_size, -1, self.num_heads, self.head_dim).transpose(1, 2) # [batch_size, num_heads, query_len, head_dim]
K = self.fc_k(key).view(batch_size, -1, self.num_heads, self.head_dim).transpose(1, 2) # [batch_size, num_heads, key_len, head_dim]
V = self.fc_v(value).view(batch_size, -1, self.num_heads, self.head_dim).transpose(1, 2) # [batch_size, num_heads, value_len, head_dim]
attn_scores = torch.matmul(Q, K.transpose(-2, -1)) / math.sqrt(self.head_dim) # [batch_size, num_heads, query_len, key_len]
attn_probs = F.softmax(attn_scores, dim=-1)
attn_output = torch.matmul(self.dropout(attn_probs), V) # [batch_size, num_heads, query_len, head_dim]
attn_output = attn_output.transpose(1, 2).contiguous().view(batch_size, -1, self.hidden_dim) # [batch_size, query_len, hidden_dim]
output = self.fc_o(attn_output)
return output, attn_probs
class FeedForward(nn.Module):
def __init__(self, hidden_dim):
super().__init__()
self.fc1 = nn.Linear(hidden_dim, hidden_dim * 4)
self.fc2 = nn.Linear(hidden_dim * 4, hidden_dim)
def forward(self, src):
# src: [batch_size, src_len, hidden_dim]
output = F.relu(self.fc1(src))
output = self.fc2(output)
return output
class PositionalEncoding(nn.Module):
def __init__(self, hidden_dim, dropout, max_len=5000):
super().__init__()
self.dropout = nn.Dropout(dropout)
pe = torch.zeros(max_len, hidden_dim)
position = torch.arange(0, max_len, dtype=torch.float).unsqueeze(1)
div_term = torch.exp(torch.arange(0, hidden_dim, 2).float() * (-math.log(10000.0) / hidden_dim))
pe[:, 0::2] = torch.sin(position * div_term)
pe[:, 1::2] = torch.cos(position * div_term)
pe = pe.unsqueeze(0).transpose(0, 1)
self.register_buffer('pe', pe)
def forward(self, x):
# x: [batch_size, seq_len, hidden_dim]
x = x + self.pe[:x.size(1), :]
return self.dropout(x)
```
Transformer源代码解析
Transformer是一种基于自注意力机制的神经网络模型,广泛应用于自然语言处理领域。其源代码主要包括以下几个部分:
1. Encoder和Decoder模块:Transformer模型由多个Encoder和Decoder模块组成,每个模块包括多头注意力机制、前馈神经网络和残差连接等组件。
2. Attention机制:Transformer中的自注意力机制通过计算查询向量、键向量和值向量之间的相似度来实现对输入序列的编码和解码。
3. Positional Encoding:为了保留输入序列中的位置信息,Transformer在输入嵌入向量中加入了位置编码向量,以便模型能够区分不同位置的单词。
4. Masking:在Decoder模块中,为了避免模型在预测时使用未来的信息,需要对解码器的输入进行masking操作。
5. 损失函数:Transformer模型通常使用交叉熵损失函数来衡量预测结果与真实标签之间的差距。
相关推荐
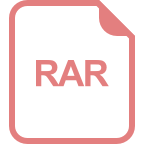
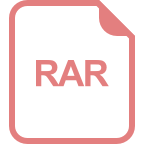












