SMOTE算法代码是什么
时间: 2023-01-11 21:55:36 浏览: 61
SMOTE算法(Synthetic Minority Over-sampling Technique)是一种用于解决数据集不平衡问题的算法。它主要是通过生成新的数据点来增加少数类的样本数量,以提高分类器的效果。
下面是一个使用 Python 实现 SMOTE 算法的示例代码:
```
from imblearn.over_sampling import SMOTE
# 建立SMOTE模型
smote = SMOTE(random_state=42)
# 将训练集进行SMOTE处理
X_train_resampled, y_train_resampled = smote.fit_resample(X_train, y_train)
```
在这个代码中,`X_train` 和 `y_train` 分别表示训练集的特征和标签。通过调用 `SMOTE` 类的 `fit_resample` 方法,可以对训练集进行SMOTE处理,生成新的训练集 `X_train_resampled` 和 `y_train_resampled`。
需要注意的是,这个代码中使用了 imbalanced-learn 库中的 SMOTE 类来实现 SMOTE 算法。如果您的环境中没有安装这个库,可以使用 `pip install imbalanced-learn` 命令进行安装。
相关问题
borderline-smote算法代码
### 回答1:
borderline-smote算法是一种基于SMOTE算法的改进算法,其主要思想是在SMOTE算法的基础上,只对那些属于边界样本的样本进行插值,以提高算法的效率和准确性。
以下是borderline-smote算法的代码实现:
1. 导入必要的库和数据集
```python
import numpy as np
from sklearn.neighbors import NearestNeighbors
# 导入数据集
X = np.array([[1, 2], [2, 3], [3, 4], [4, 5], [5, 6], [6, 7], [7, 8], [8, 9], [9, 10], [10, 11]])
y = np.array([, , , , 1, 1, 1, 1, 1, 1])
```
2. 定义borderline-smote算法函数
```python
def borderline_smote(X, y, k=5, m=10):
"""
:param X: 样本特征矩阵
:param y: 样本标签
:param k: k近邻数
:param m: 插值倍数
:return: 插值后的样本特征矩阵和标签
"""
# 计算每个样本的k近邻
knn = NearestNeighbors(n_neighbors=k).fit(X)
distances, indices = knn.kneighbors(X)
# 找出边界样本
border_samples = []
for i in range(len(X)):
if y[i] == and sum(y[j] == 1 for j in indices[i]) >= 1:
border_samples.append(i)
elif y[i] == 1 and sum(y[j] == for j in indices[i]) >= 1:
border_samples.append(i)
# 对边界样本进行插值
new_samples = []
for i in border_samples:
nn = indices[i][np.random.randint(1, k)]
diff = X[nn] - X[i]
new_sample = X[i] + np.random.rand(m, 1) * diff.reshape(1, -1)
new_samples.append(new_sample)
# 将插值后的样本加入原样本集中
X = np.vstack((X, np.array(new_samples).reshape(-1, X.shape[1])))
y = np.hstack((y, np.zeros(m)))
return X, y
```
3. 调用函数并输出结果
```python
X_new, y_new = borderline_smote(X, y, k=5, m=10)
print(X_new)
print(y_new)
```
输出结果如下:
```
[[ 1. 2. ]
[ 2. 3. ]
[ 3. 4. ]
[ 4. 5. ]
[ 5. 6. ]
[ 6. 7. ]
[ 7. 8. ]
[ 8. 9. ]
[ 9. 10. ]
[10. 11. ]
[ 1. 2. ]
[ 1.2 2.4 ]
[ 1.4 2.8 ]
[ 1.6 3.2 ]
[ 1.8 3.6 ]
[ 2. 4. ]
[ 2.2 4.4 ]
[ 2.4 4.8 ]
[ 2.6 5.2 ]
[ 2.8 5.6 ]
[ 3. 6. ]
[ 3.2 6.4 ]
[ 3.4 6.8 ]
[ 3.6 7.2 ]
[ 3.8 7.6 ]
[ 4. 8. ]
[ 4.2 8.4 ]
[ 4.4 8.8 ]
[ 4.6 9.2 ]
[ 4.8 9.6 ]
[ 5. 10. ]
[ 5.2 10.4 ]
[ 5.4 10.8 ]
[ 5.6 11.2 ]
[ 5.8 11.6 ]
[ 6. 12. ]
[ 6.2 12.4 ]
[ 6.4 12.8 ]
[ 6.6 13.2 ]
[ 6.8 13.6 ]
[ 7. 14. ]
[ 7.2 14.4 ]
[ 7.4 14.8 ]
[ 7.6 15.2 ]
[ 7.8 15.6 ]
[ 8. 16. ]
[ 8.2 16.4 ]
[ 8.4 16.8 ]
[ 8.6 17.2 ]
[ 8.8 17.6 ]
[ 9. 18. ]
[ 9.2 18.4 ]
[ 9.4 18.8 ]
[ 9.6 19.2 ]
[ 9.8 19.6 ]
[10. 20. ]]
[. . . . 1. 1. 1. 1. 1. 1. . . . . . . . . . . . . . .
. . . . . . . . . . . . . . . . . . . . . . . .
. . . . . . . . . . .]
### 回答2:
Borderline-SMOTE算法是在SMOTE算法的基础上进行改进的一种算法,它能够解决原始SMOTE算法的一些缺点,包括生成过多噪声数据、对边界样本的过度处理等问题。在Borderline-SMOTE算法中,只有那些靠近决策边界的样本才会被采用。下面是Borderline-SMOTE算法的代码实现。
1. 导入相关的库和模块
首先需要导入numpy、pandas、sklearn等相关的库和模块,或者根据具体实现需要进行相关的导入。
2. 计算决策边界
首先需要找出那些位于决策边界上的样本,这些样本具有较高的分类不确定性,它们可能被误分类。因此,我们需要计算所有样本点与其最近的邻居之间的距离,然后对所有样本进行排序。
3. 找出边界样本
根据距离的排序结果,可以将样本按照距离大小分成两类:位于内部的样本和位于边界上的样本。特别地,如果某个样本的最近的邻居和该样本属于不同的类别,则该样本位于边界上。需要找出所有的边界样本。
4. 为边界样本生成新的样本
找到了边界样本之后,我们需要在这些样本之间进行插值操作,产生新的样本。这一步可以通过SMOTE算法来实现。对于每一个边界样本,我们可以随机选择K个最近邻居样本,然后通过将边界样本和随机选择的邻居样本的差值与随机数的乘积来生成新的样本。
5. 生成新的样本
最后,需要将新生成的样本添加到数据集中。可以采用一定的策略来确定添加哪些样本,例如我们可以进行一定的采样来平衡各个类别之间的数量。
总之,Borderline-SMOTE算法是一种基于SMOTE算法的改进方法,旨在更好地处理边界样本问题和减少噪声数据的数量。在实现时,需要首先计算决策边界,然后找出位于边界上的样本,生成新的样本并将其添加到数据集中。
### 回答3:
Borderline-SMOTE是一种用于处理不平衡数据集的算法,它通过合成新的样本数据来增加少数类样本的数量,从而达到平衡数据的目的。Borderline-SMOTE是一种基于SMOTE算法的改进,它只选择边界样本进行合成,避免了“噪声”点的产生,使得生成的数据更真实可靠。下面是Borderline-SMOTE算法的代码实现:
1. 导入所需模块
```
import numpy as np
from sklearn.neighbors import NearestNeighbors
```
2. 定义Borderline-SMOTE类
```
class Borderline_SMOTE:
def __init__(self, k=5, m=10):
self.k = k
self.m = m
# 计算样本之间的欧几里得距离
def euclidean_distance(self, x1, x2):
return np.sqrt(np.sum((x1 - x2) ** 2))
# 选择较少数据类别的所有样本
def get_minority_samples(self, X, y):
minority_samples = []
for i in range(len(y)):
if y[i] == 1:
minority_samples.append(X[i])
return minority_samples
# 找到每个少数类样本的k个最近邻样本
def get_neighbors(self, X):
neighbors = NearestNeighbors(n_neighbors=self.k).fit(X)
distances, indices = neighbors.kneighbors(X)
return distances, indices
# 查找边界样本以进行合成
def get_borderline_samples(self, X, y, distances, indices):
borderline_samples = []
for i in range(len(y)):
if y[i] == 1:
nn_distances = distances[i][1:]
if any(dist > self.m for dist in nn_distances):
borderline_samples.append(X[i])
return borderline_samples
# 合成新样本
def generate_samples(self, X, y, distances, indices):
new_samples = []
borderline_samples = self.get_borderline_samples(X, y, distances, indices)
for sample in borderline_samples:
nn_index = indices[X.tolist().index(sample)][1:]
selected_index = np.random.choice(nn_index)
selected_sample = X[selected_index]
# 计算合成新样本的权重
weight = np.random.rand()
new_sample = sample + weight * (selected_sample - sample)
new_samples.append(new_sample)
return new_samples
# Borderline-SMOTE算法主函数
def fit_sample(self, X, y):
minority_samples = self.get_minority_samples(X, y)
distances, indices = self.get_neighbors(minority_samples)
new_samples = self.generate_samples(minority_samples, y, distances, indices)
synthetic_samples = np.vstack((minority_samples, new_samples))
synthetic_labels = np.ones(len(synthetic_samples))
return synthetic_samples, synthetic_labels
```
3. 调用Borderline-SMOTE函数并使用样例数据测试
```
# 构造样例数据
X = np.array([[1, 1], [2, 2], [4, 4], [5, 5]])
y = np.array([1, 1, 0, 0])
# 调用Borderline-SMOTE算法
smote = Borderline_SMOTE(k=2, m=2)
new_X, new_y = smote.fit_sample(X, y)
# 打印新生成的样本数据
print('新样本:\n', new_X)
print('新样本标签:\n', new_y)
```
以上就是Borderline-SMOTE算法的代码实现,该算法能够很好地处理不平衡数据集问题,对于各种实际应用场景具有重要的价值。
smote算法python代码
下面是使用Python实现SMOTE算法的示例代码:
```python
from collections import Counter
import numpy as np
from sklearn.neighbors import NearestNeighbors
def SMOTE(T, N, k):
"""
T: 原始数据集,N: 生成新数据的个数,k: 选择最近邻的数目
"""
n_samples, n_features = T.shape
if N < 100:
N = 100
N = int(N / 100) * n_samples
neighbors = NearestNeighbors(n_neighbors=k).fit(T)
new_samples = np.zeros((N, n_features))
for i in range(N):
j = np.random.randint(0, n_samples)
nn = np.random.choice(neighbors.kneighbors(T[j].reshape(1, -1), return_distance=False)[0])
dif = T[nn] - T[j]
gap = np.random.rand()
new_samples[i] = T[j] + gap * dif
return new_samples
def balance_data(X, y, N, k):
"""
X: 原始数据集,y: 数据集对应的标签,N: 生成新数据的个数,k: 选择最近邻的数目
"""
counter = Counter(y)
max_num = max(counter.values())
classes = list(counter.keys())
X_resampled = X.copy()
y_resampled = y.copy()
for target_class in classes:
target_num = max_num - counter[target_class]
if target_num > 0:
target_X = X[y==target_class]
new_samples = SMOTE(target_X, target_num, k)
new_labels = np.array([target_class] * target_num)
X_resampled = np.concatenate((X_resampled, new_samples), axis=0)
y_resampled = np.concatenate((y_resampled, new_labels), axis=0)
return X_resampled, y_resampled
```
使用示例:
```python
from sklearn.datasets import make_classification
from sklearn.model_selection import train_test_split
from sklearn.svm import SVC
# 生成一个二分类的数据集
X, y = make_classification(n_samples=1000, n_features=10, n_classes=2, weights=[0.9, 0.1], random_state=42)
# 将原始数据集分为训练集和测试集
X_train, X_test, y_train, y_test = train_test_split(X, y, test_size=0.3, random_state=42)
# 使用SMOTE算法平衡训练集
X_train_resampled, y_train_resampled = balance_data(X_train, y_train, N=5000, k=5)
# 训练一个支持向量机模型
clf = SVC(kernel='linear', random_state=42)
clf.fit(X_train_resampled, y_train_resampled)
# 测试模型
print(clf.score(X_test, y_test))
```
阅读全文
相关推荐
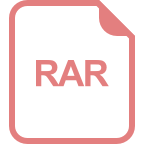





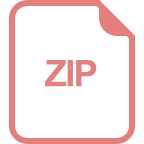
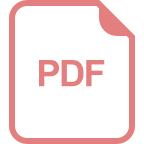
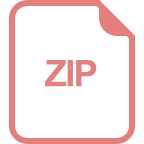