pytorch的一维卷积神经网络代码以及向前传播代码
时间: 2023-09-23 22:09:44 浏览: 58
以下是Python中使用Pytorch实现一维卷积神经网络的代码和向前传播的代码:
```python
import torch
import torch.nn as nn
# 定义一维卷积神经网络模型
class ConvNet(nn.Module):
def __init__(self, num_classes=10):
super(ConvNet, self).__init__()
self.layer1 = nn.Sequential(
nn.Conv1d(in_channels=1, out_channels=16, kernel_size=5, stride=1, padding=2),
nn.BatchNorm1d(16),
nn.ReLU(),
nn.MaxPool1d(kernel_size=2, stride=2))
self.layer2 = nn.Sequential(
nn.Conv1d(16, 32, kernel_size=5, stride=1, padding=2),
nn.BatchNorm1d(32),
nn.ReLU(),
nn.MaxPool1d(kernel_size=2, stride=2))
self.fc = nn.Linear(320, num_classes)
def forward(self, x):
out = self.layer1(x)
out = self.layer2(out)
out = out.reshape(out.size(0), -1)
out = self.fc(out)
return out
# 定义向前传播代码
model = ConvNet()
criterion = nn.CrossEntropyLoss()
optimizer = torch.optim.Adam(model.parameters(), lr=0.001)
# 训练循环
for epoch in range(num_epochs):
for i, (images, labels) in enumerate(train_loader):
images = images.reshape(-1, 1, 784)
outputs = model(images)
loss = criterion(outputs, labels)
optimizer.zero_grad()
loss.backward()
optimizer.step()
# 测试
with torch.no_grad():
correct = 0
total = 0
for images, labels in test_loader:
images = images.reshape(-1, 1, 784)
outputs = model(images)
_, predicted = torch.max(outputs.data, 1)
total += labels.size(0)
correct += (predicted == labels).sum().item()
print('Accuracy of the network on the 10000 test images: {} %'.format(100 * correct / total))
```
希望以上代码能够帮到你。
相关推荐
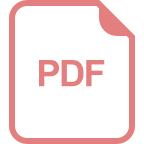
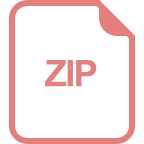














