.使用MH抽样方法从Rayleigh分布中抽样,Rayleigh分布的密度函数为: f(x)= e- , x≥0, σ>0. 建议分布取自由度为Xt的χ2分布。
时间: 2024-04-16 17:29:26 浏览: 163
要Metropolis-Hastings (MH)抽样方法从Ray分布中抽样,可以选择χ2分布作为建议分布。
首先,我们需要了解Rayleigh分布的概率密度函数和χ2分布的概率密度函数。
Rayleigh分布的概率密度函数为:
f(x) = (x / σ^2) * exp(-x^2 / (2σ^2)), x ≥ 0, σ > 0
χ2分布的概率密度函数为:
g(x) = (1 / (2^(ν/2) * Γ(ν/2))) * x^(ν/2 - 1) * exp(-x/2), x ≥ 0, ν > 0
其中,Γ(ν/2)表示Gamma函数。
接下来,我们可以使用MH抽样方法按以下步骤进行操作:
1. 初始化参数:设置初始样本值x0、步长参数ε和抽样次数N。
2. 对于每次抽样i从1到N:
a. 从建议分布χ2(ν)中抽取一个样本值y,可以使用numpy.random.chisquare函数。
b. 计算接受率α = min(1, (f(y) * g(x_i)) / (f(x_i) * g(y))),其中f(x)是Rayleigh分布的密度函数。
c. 生成一个随机数u,如果u < α,则接受新样本值x_i+1 = y,否则保持原样本值x_i+1 = x_i。
3. 返回抽样结果x1, x2, ..., xN。
以下是使用Python代码实现从Rayleigh分布中抽样的示例:
```python
import numpy as np
import matplotlib.pyplot as plt
# 定义Rayleigh分布的概率密度函数
def rayleigh_pdf(x, sigma):
return (x / sigma**2) * np.exp(-x**2 / (2 * sigma**2))
# 定义χ2分布的概率密度函数
def chi_square_pdf(x, nu):
return (1 / (2**(nu/2) * np.math.gamma(nu/2))) * x**((nu/2) - 1) * np.exp(-x/2)
# 使用MH抽样方法从Rayleigh分布中抽样
def mh_sampling(sigma, nu, x0, epsilon, num_samples):
samples = [x0]
for i in range(num_samples):
y = np.random.chisquare(nu)
acceptance_ratio = min(1, (rayleigh_pdf(y, sigma) * chi_square_pdf(samples[i], nu)) /
(rayleigh_pdf(samples[i], sigma) * chi_square_pdf(y, nu)))
u = np.random.uniform(0, 1)
if u < acceptance_ratio:
samples.append(y)
else:
samples.append(samples[i])
return samples
# 设置参数
sigma = 1 # Rayleigh分布的参数
nu = 2 # χ2分布的参数
x0 = 1 # 初始样本值
epsilon = 0.5 # 步长参数
num_samples = 10000
# 使用MH抽样方法从Rayleigh分布中抽样
samples = mh_sampling(sigma, nu, x0, epsilon, num_samples)
# 绘制抽样结果的直方图
plt.hist(samples, bins=50, density=True, alpha=0.7, label='Samples')
x = np.linspace(0, max(samples), 1000)
plt.plot(x, rayleigh_pdf(x, sigma), 'r', label='Rayleigh PDF')
plt.xlabel('x')
plt.ylabel('Density')
plt.title('MH Sampling from Rayleigh Distribution')
plt.legend()
plt.show()
```
在上述代码中,我们首先定义了Rayleigh分布的概率密度函数`rayleigh_pdf`和χ2分布的概率密度函数`chi_square_pdf`。
然后,我们实现了`mh_sampling`函数来执行MH抽样方法。在每次抽样中,从χ2分布中抽取一个样本值y,并计算接受率α。根据接受率和随机数u的比较,决定是否接受新样本值。
最后,我们设置了参数sigma、nu、x0、epsilon和num_samples,并调用`mh_sampling`函数来执行抽样过程。将抽样结果存储在`samples`数组中。
最终,我们使用`matplotlib.pyplot`库绘制了抽样结果的直方图,并将Rayleigh分布的概率密度函数绘制为红色曲线。
请注意,由于使用了随机数生成器,每次运行代码都会得到不同的结果。
阅读全文
相关推荐
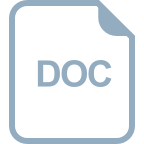
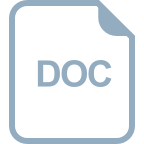
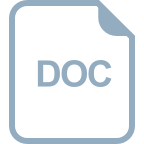



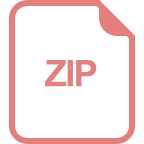
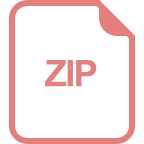
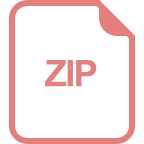
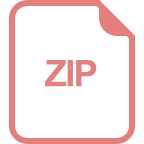
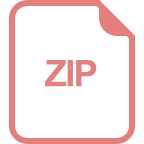
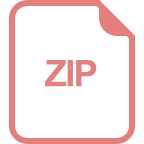
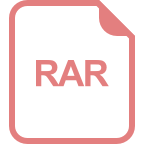
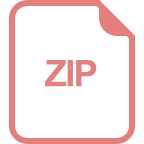
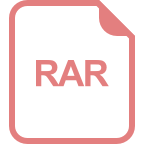
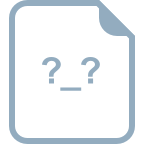
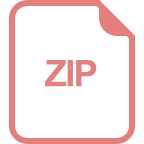
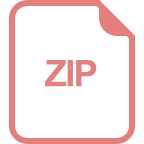
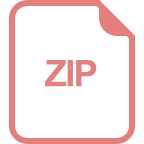