pytorch adaptive pooling
时间: 2023-08-08 13:01:34 浏览: 56
PyTorch中的自适应池化(adaptive pooling)是一种可以动态调整输入尺寸的池化操作。与传统的池化操作不同,传统池化操作需要指定固定的池化窗口大小,导致输入尺寸必须被控制在特定的尺寸范围内。而自适应池化可以根据输入的尺寸自动调整池化操作的窗口大小。
通过自适应池化,我们可以传递任意大小的输入张量,并且输出的尺寸可以被动态地确定。具体来说,自适应池化接收一个输入张量和一个目标输出尺寸(通常是一个二维的元组或整数)。然后,它会根据目标输出尺寸自动计算池化窗口的大小,以及如何对输入进行采样。在池化操作中,自适应池化可以在水平和垂直维度上应用不同的采样尺寸。
自适应池化在处理卷积神经网络(CNN)中的图像数据时非常有用。它可以处理不同尺寸的图像,而不需要将它们缩放到相同的大小。这对于处理不同尺寸的图像输入非常方便,例如图像分类、目标检测和图像分割等任务。
PyTorch提供了两种类型的自适应池化操作,分别是AdaptiveMaxPool和AdaptiveAvgPool。AdaptiveMaxPool采用最大池化操作,并根据输入和目标输出尺寸动态确定池化窗口大小。AdaptiveAvgPool采用平均池化操作,同样可以根据输入和目标输出尺寸动态确定池化窗口大小。
总而言之,PyTorch的自适应池化是一种灵活的池化操作,可以动态调整输入尺寸,而不需要限制在固定的池化窗口大小上。这使得处理不同尺寸的数据变得更加便捷,并且在处理图像等任务时非常有用。
相关问题
用pytorch实现global avg pooling
### 回答1:
在PyTorch中,实现全局平均池化(global average pooling)非常简单。可以使用`torch.nn.functional`模块中的`adaptive_avg_pool2d`函数实现。以下是一个简单的代码示例:
```python
import torch.nn.functional as F
# 假设输入的维度为(batch_size, channels, height, width)
x = torch.randn(16, 64, 32, 32)
# 全局平均池化
pooling = F.adaptive_avg_pool2d(x, (1, 1))
# 输出维度为(batch_size, channels, 1, 1)
print(pooling.shape)
```
在这个示例中,`x`是一个随机初始化的四维张量。我们使用`F.adaptive_avg_pool2d`函数对`x`进行全局平均池化。函数的第一个参数是输入张量,第二个参数是目标输出尺寸,这里我们将输出的高度和宽度都设为1,即进行全局平均池化。最后,我们打印出`pooling`的形状,可以看到输出的形状为`(16, 64, 1, 1)`,即对于每个样本和通道,输出了一个标量平均值。
### 回答2:
用PyTorch实现全局平均池化(global average pooling),可以通过调用`torch.mean()`函数来实现。
全局平均池化是一种常用的池化操作,它将输入的特征图的每个通道上的所有元素求平均,得到每个通道上的一个标量值。这样就可以将任意大小的输入特征图汇集为固定大小的特征向量。
以下是一个实现全局平均池化的示例代码:
```
import torch
import torch.nn as nn
# 定义一个三通道的输入特征图
input = torch.randn(1, 3, 5, 5)
# 定义全局平均池化层
global_avg_pool = nn.AdaptiveAvgPool2d(1)
# 使用全局平均池化层进行池化操作
output = global_avg_pool(input)
print(output.shape) # 输出:torch.Size([1, 3, 1, 1])
```
在上述代码中,我们首先导入必要的库并定义一个三通道的输入特征图`input`。然后,我们使用`nn.AdaptiveAvgPool2d()`函数来定义一个全局平均池化层`global_avg_pool`,其中参数1表示输出的大小为1x1。
最后,我们将输入特征图传递给全局平均池化层进行池化操作,并打印输出的形状,可以看到输出的特征图形状为`torch.Size([1, 3, 1, 1])`,其中1表示batch size,3表示通道数,1x1表示池化后的特征图尺寸。
这样,我们就成功地使用PyTorch实现了全局平均池化。
### 回答3:
在PyTorch中,可以使用`nn.AdaptiveAvgPool2d`模块来实现全局平均池化(Global Average Pooling)操作。全局平均池化是一种常用于图像分类任务中的特征提取方法,其将输入特征图的每个通道的所有元素相加,并将结果除以特征图的尺寸,从而获得每个通道的平均值作为输出。
下面是使用PyTorch实现全局平均池化的示例代码:
```python
import torch
import torch.nn as nn
# 定义一个输入特征图
input_features = torch.randn(1, 64, 32, 32) # 输入特征图大小为[batch_size, channels, height, width]
# 使用nn.AdaptiveAvgPool2d实现全局平均池化
global_avg_pool = nn.AdaptiveAvgPool2d((1, 1)) # 将特征图的尺寸调整为(1, 1)
output = global_avg_pool(input_features)
# 打印输出的形状
print(output.shape) # 输出的形状为[batch_size, channels, 1, 1]
```
在上述代码中,我们首先创建了一个大小为[1, 64, 32, 32]的输入特征图,其中1表示batch大小,64表示通道数,32x32表示特征图的高度和宽度。然后,我们使用`nn.AdaptiveAvgPool2d`模块创建了一个全局平均池化层,将特征图的尺寸调整为(1, 1)。最后,我们将输入特征图通过该全局平均池化层进行处理,得到输出特征图。打印输出的形状可以看到,输出特征图的大小为[1, 64, 1, 1],其中64表示通道数,而1x1表示特征图的尺寸已经被调整为了(1, 1)。
pointrend pytorch
PointRend是Facebook AI Research在2019年提出的一种借助点采样(point sampling)技术提升实例分割(instance segmentation)性能的方法,这一方法被应用于Mask R-CNN模型中,提高了实例分割任务的表现。
PointRend的核心思想是通过点采样(point sampling)的方式对实例的局部区域进行特征提取,然后再对这些局部特征进行全局汇聚来获取最终的实例特征表示。具体地,PointRend将Mask R-CNN的RoIAlign操作替换为PointRoIAlign操作,这样可以在每个采样点处提取出一个局部特征。然后在这些局部特征上,PointRend使用自适应池化(adaptive pooling)的方式,将局部特征汇聚到一个全局表达中,这个表达即为最终的实例特征表示。
PointRend在实验中表现出了很好的性能,特别是在实例大小差距较大的情况下效果最为显著。目前,PointRend已经被整合到Facebook AI Research开源的detectron2库中,可以直接使用pytorch实现。
相关推荐
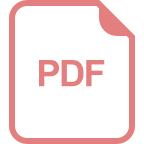
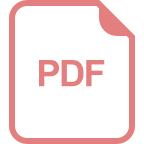











